RIGA: Rotation-Invariant and Globally-Aware Descriptors for Point Cloud Registration
IEEE TRANSACTIONS ON PATTERN ANALYSIS AND MACHINE INTELLIGENCE(2024)
摘要
Successful point cloud registration relies on accurate correspondences established upon powerful descriptors. However, existing neural descriptors either leverage a rotation-variant backbone whose performance declines under large rotations, or encode local geometry that is less distinctive. To address this issue, we introduce RIGA to learn descriptors that are Rotation-Invariant by design and Globally-Aware. From the Point Pair Features (PPFs) of sparse local regions, rotation-invariant local geometry is encoded into geometric descriptors. Global awareness of 3D structures and geometric context is subsequently incorporated, both in a rotation-invariant fashion. More specifically, 3D structures of the whole frame are first represented by our global PPF signatures, from which structural descriptors are learned to help geometric descriptors sense the 3D world beyond local regions. Geometric context from the whole scene is then globally aggregated into descriptors. Finally, the description of sparse regions is interpolated to dense point descriptors, from which correspondences are extracted for registration. To validate our approach, we conduct extensive experiments on both object- and scene-level data. With large rotations, RIGA surpasses the state-of-the-art methods by a margin of 8 ° in terms of the Relative Rotation Error on ModelNet40 and improves the Feature Matching Recall by at least 5 percentage points on 3DLoMatch.
更多查看译文
关键词
Point cloud compression,Geometry,Three-dimensional displays,Task analysis,Benchmark testing,Shape,Pattern analysis,Point cloud registration,rotation-invariant descriptors,globally-aware descriptors,coarse-to-fine correspondences
AI 理解论文
溯源树
样例
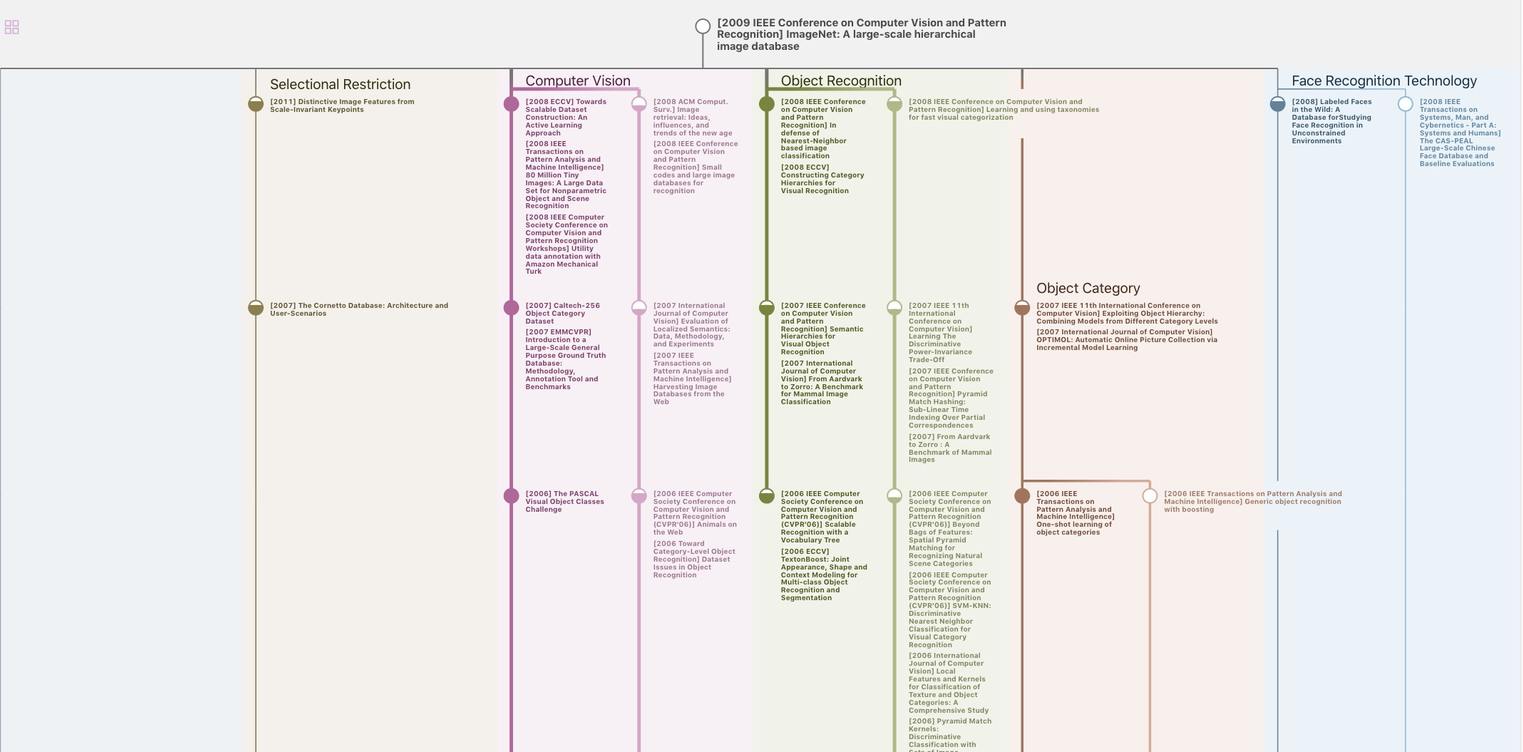
生成溯源树,研究论文发展脉络
Chat Paper
正在生成论文摘要