Sliding-Priors for Bayesian Information Fusion in SCADA+PMU-based State Estimation
2022 17TH INTERNATIONAL CONFERENCE ON PROBABILISTIC METHODS APPLIED TO POWER SYSTEMS (PMAPS)(2022)
摘要
This paper describes a new development within the conceptual framework BAYSE (Bayesian State Estimation), which enables the full integration of the SCADA (Supervisory Control and Data Acquisition) data with PMU (phasor measurement units) data. It is based on Bayesian inference principles and extends the concept of the prior distributions to accommodate a broad set of past state conditions, under a sliding window approach. By choosing an appropriate window length, the method enhances accuracy under stationary conditions, with a reduced impact under system changes. The work also submits a rectangular coordinates transformation procedure, based on the Jacobian method, to consistently integrate polar coordinates estimations with the PMU linear model (in rectangular coordinates). The paper presents the new approach in proof-of concept mode over a didactic test-bed, using real PMU time series, to emphasize the enhanced accuracy and good asymptotic properties.
更多查看译文
关键词
State Estimation,Bayesian Inference,Information Fusion,Phasor Measurement Units,Power Systems
AI 理解论文
溯源树
样例
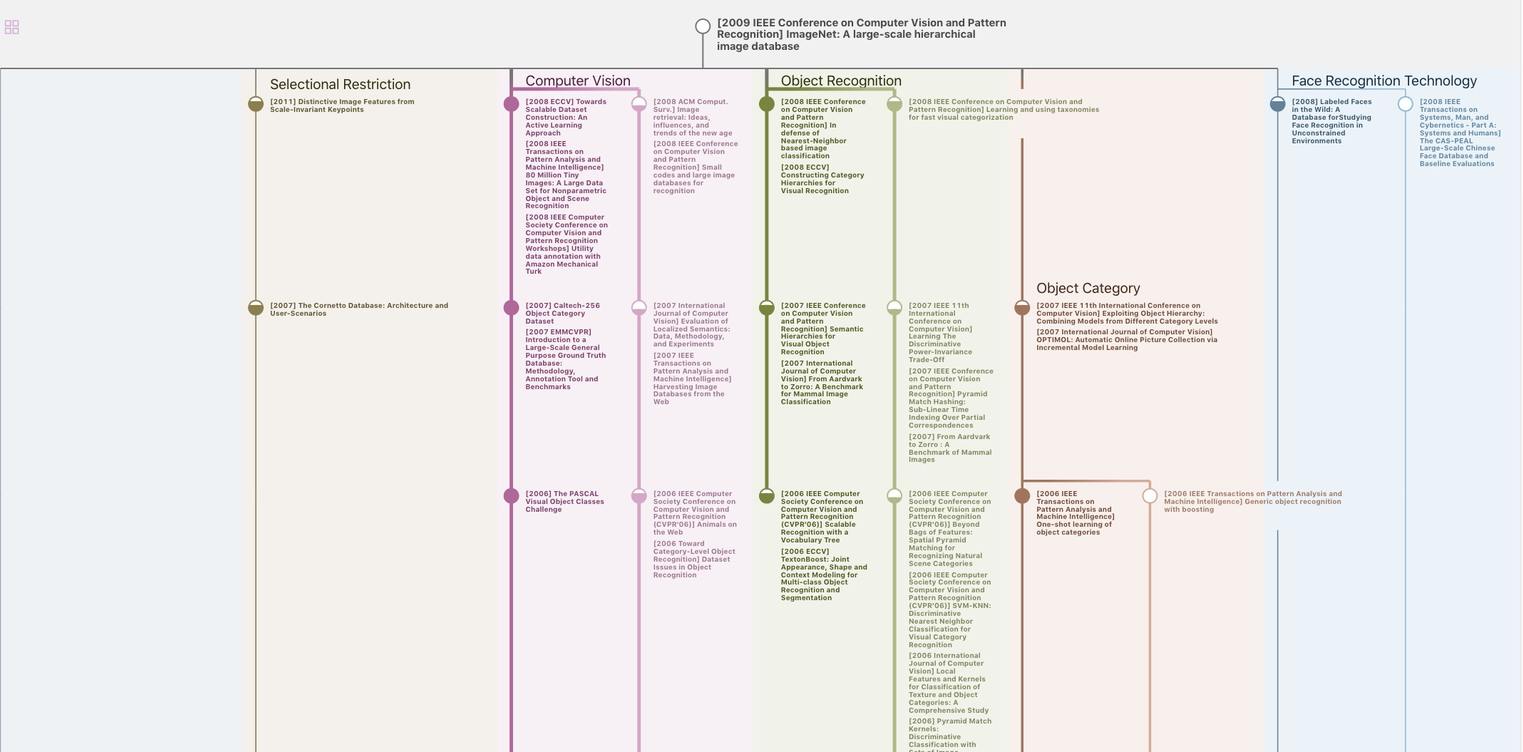
生成溯源树,研究论文发展脉络
Chat Paper
正在生成论文摘要