NanoSNP: a Progressive and Haplotype-Aware SNP Caller on Low-Coverage Nanopore Sequencing Data
Bioinformatics (Oxford, England)(2023)
摘要
Motivation: Oxford Nanopore sequencing has great potential and advantages in population-scale studies. Due to the cost of sequencing, the depth of whole-genome sequencing for per individual sample must be small. However, the existing single nucleotide polymorphism (SNP) callers are aimed at high-coverage Nanopore sequencing reads. Detecting the SNP variants on low-coverage Nanopore sequencing data is still a challenging problem.Results: We developed a novel deep learning-based SNP calling method, NanoSNP, to identify the SNP sites (excluding short indels) based on low-coverage Nanopore sequencing reads. In this method, we design a multi-step, multi-scale and haplotype-aware SNP detection pipeline. First, the pileup model in NanoSNP utilizes the naive pileup feature to predict a subset of SNP sites with a Bi-long short-term memory (LSTM) network. These SNP sites are phased and used to divide the low-coverage Nanopore reads into different haplotypes. Finally, the long-range haplotype feature and short-range pileup feature are extracted from each haplotype. The haplotype model combines two features and predicts the genotype for the candidate site using a Bi-LSTM network. To evaluate the performance of NanoSNP, we compared NanoSNP with Clair, Clair3, Pepper-DeepVariant and NanoCaller on the low-coverage (similar to 16x) Nanopore sequencing reads. We also performed cross-genome testing on six human genomes HG002-HG007, respectively. Comprehensive experiments demonstrate that NanoSNP outperforms Clair, Pepper-DeepVariant and NanoCaller in identifying SNPs on low-coverage Nanopore sequencing data, including the difficult to-map regions and major histocompatibility complex regions in the human genome. NanoSNP is comparable to Clair3 when the coverage exceeds 16x.
更多查看译文
关键词
Transcriptional Landscape
AI 理解论文
溯源树
样例
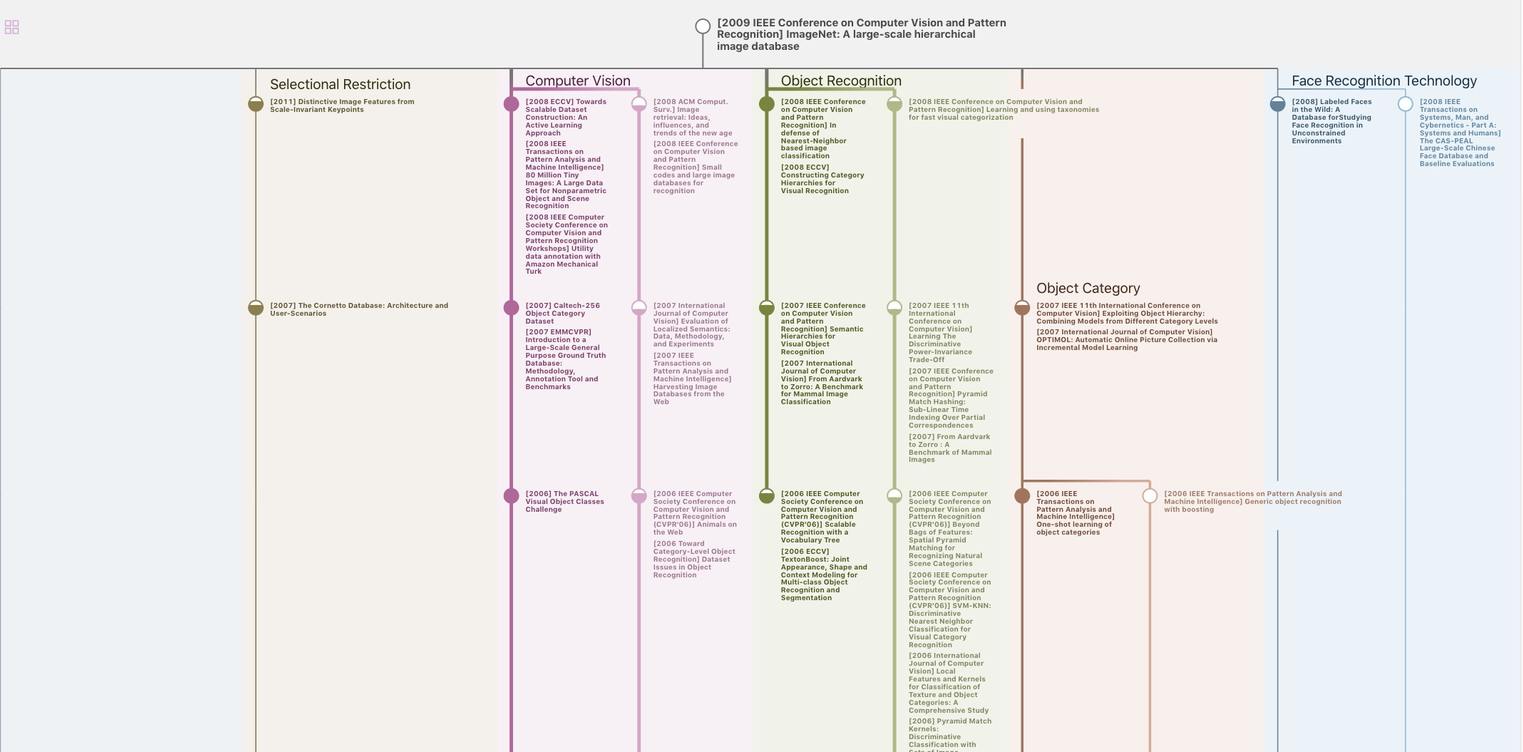
生成溯源树,研究论文发展脉络
Chat Paper
正在生成论文摘要