Inadequate Sampling of the Soundscape Leads to Overoptimistic Estimates of Recogniser Performance: a Case Study of Two Sympatric Macaw Species
BIOACOUSTICS-THE INTERNATIONAL JOURNAL OF ANIMAL SOUND AND ITS RECORDING(2024)
摘要
Passive acoustic monitoring (PAM) - autonomously recording ambient sound - could dramatically increase the scale and robustness of species monitoring in rainforest ecosystems. PAM generates large volumes of data that require automated methods of target species detection. Species-specific recognisers, which often use supervised machine learning, can achieve this goal. However, they require a large training dataset of target and non-target signals, which is time-consuming and challenging to create. Unfortunately, very little information about creating training datasets for supervised machine learning recognisers is available, especially for tropical ecosystems. Here, we show an iterative approach to creating a training dataset that improved recogniser precision from 0.12 to 0.55. By sampling background noise using an initial small recogniser, we can address one of the significant challenges of training dataset creation in acoustically diverse environments. Our work demonstrates that recognisers will likely fail in real-world settings unless the training dataset size is large enough and sufficiently representative of the ambient soundscape. We outline a workflow that can provide users with an accessible way to create a species-specific PAM recogniser that addresses these issues for tropical rainforest environments. Our work provides important lessons for PAM practitioners wanting to develop species-specific recognisers for acoustically diverse ecosystems.
更多查看译文
关键词
Passive acoustic monitoring,machine learning,training dataset,iteration,species-specific
AI 理解论文
溯源树
样例
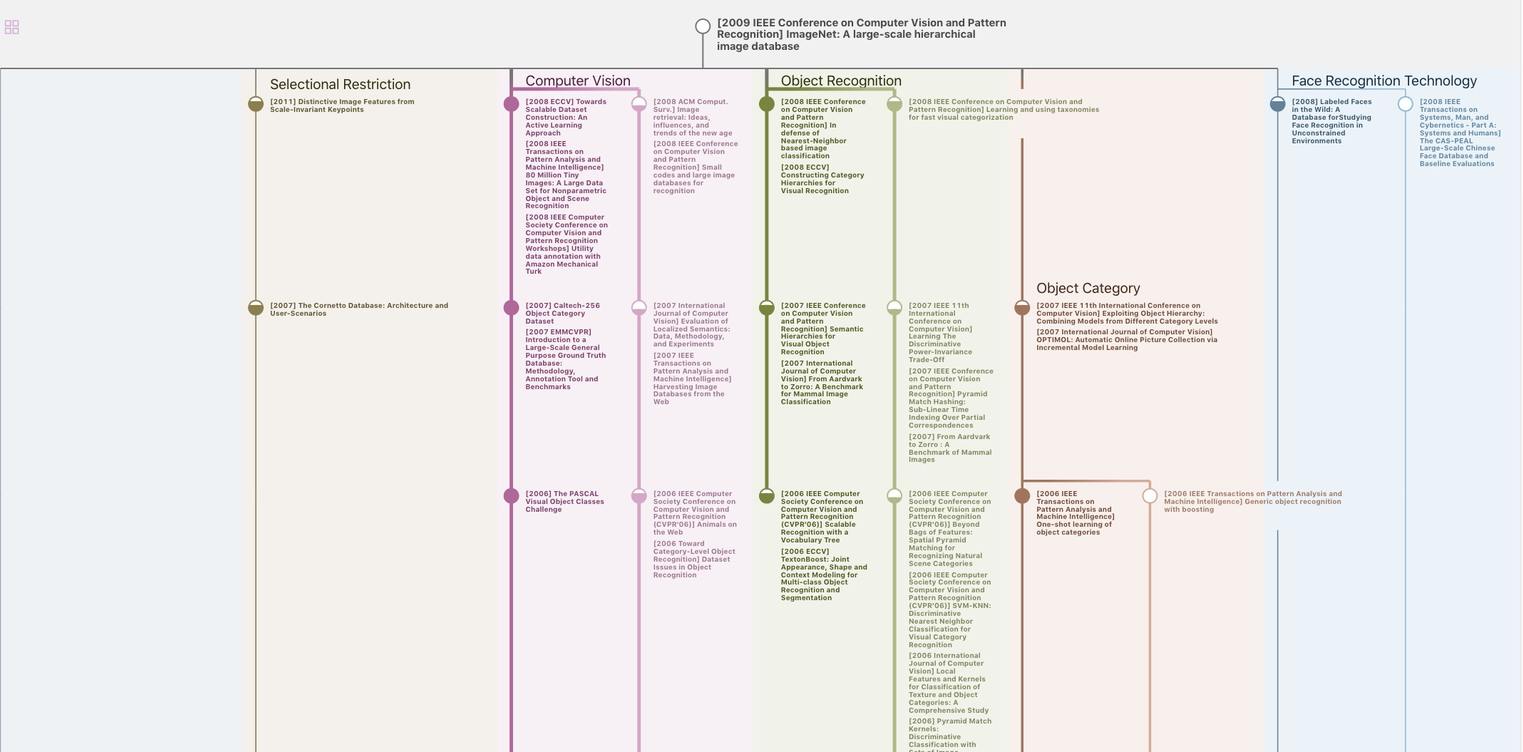
生成溯源树,研究论文发展脉络
Chat Paper
正在生成论文摘要