Deep Implicit Distribution Alignment Networks for Cross-Corpus Speech Emotion Recognition.
IEEE TRANSACTIONS ON COMPUTATIONAL SOCIAL SYSTEMS(2024)
摘要
In this paper, we propose a novel deep transfer learning method called deep implicit distribution alignment networks (DIDAN) to deal with cross-corpus speech emotion recognition (SER) problem, in which the labeled training (source) and unlabeled testing (target) speech signals come from different corpora. Specifically, DIDAN first adopts a simple deep regression network consisting of a set of convolutional and fully connected layers to directly regress the source speech spectrums into the emotional labels such that the proposed DIDAN can own the emotion discriminative ability. Then, such ability is transferred to be also applicable to the target speech samples regardless of corpus variance by resorting to a well-designed regularization term called implicit distribution alignment (IDA). Unlike widely-used maximum mean discrepancy (MMD) and its variants, the proposed IDA absorbs the idea of sample reconstruction to implicitly align the distribution gap, which enables DIDAN to learn both emotion discriminative and corpus invariant features from speech spectrums. To evaluate the proposed DIDAN, extensive cross-corpus SER experiments on widely-used speech emotion corpora are carried out. Experimental results show that the proposed DIDAN can outperform lots of recent state-of-the-art methods in coping with the cross-corpus SER tasks.
更多查看译文
关键词
Cross-corpus speech emotion recognition,deep learning,speech emotion recognition (SER),transfer learning,unsupervised domain adaptation (DA)
AI 理解论文
溯源树
样例
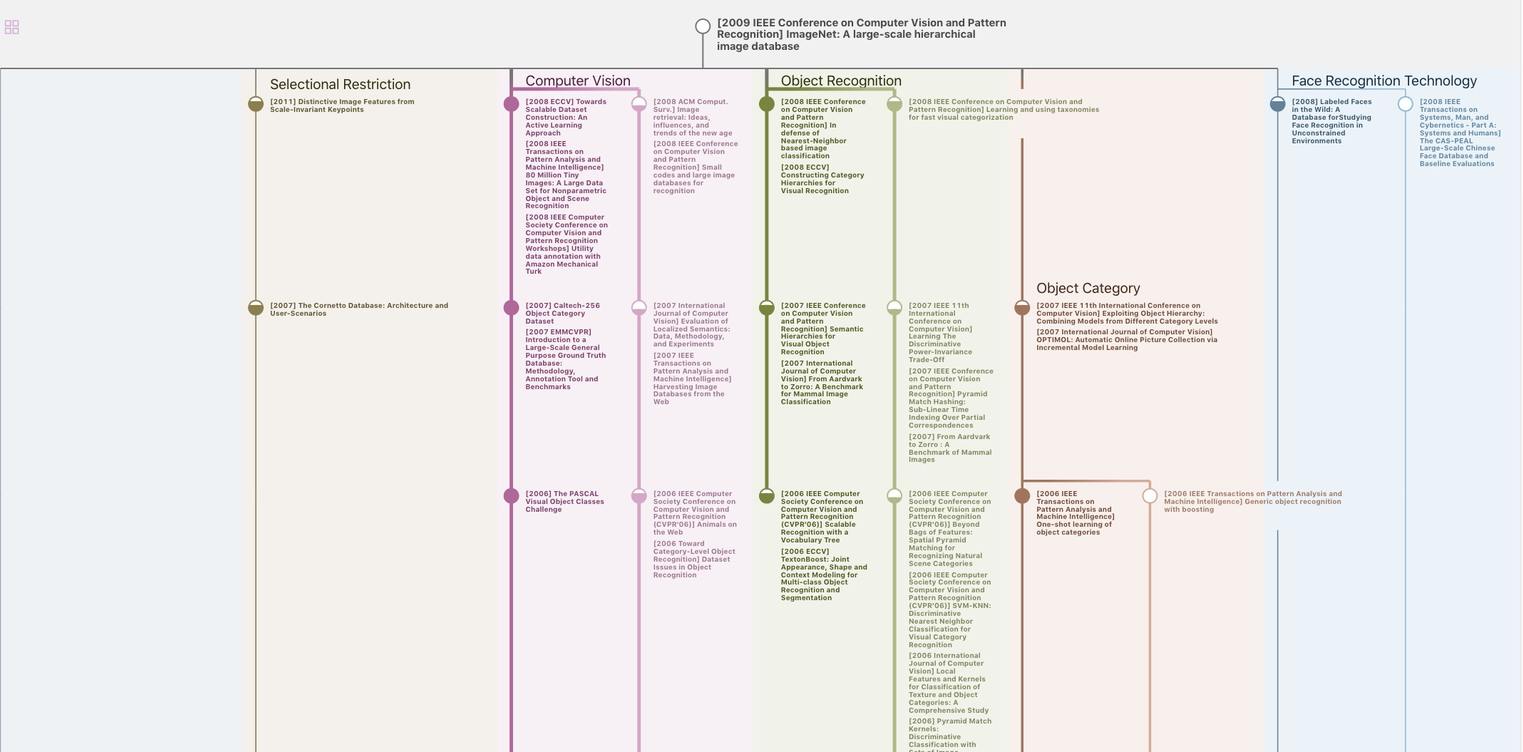
生成溯源树,研究论文发展脉络
Chat Paper
正在生成论文摘要