Machine Learning-Based Jet and Event Classification at the Electron-Ion Collider with Applications to Hadron Structure and Spin Physics
Journal of High Energy Physics(2023)
摘要
A bstract We explore machine learning-based jet and event identification at the future Electron-Ion Collider (EIC). We study the effectiveness of machine learning-based classifiers at relatively low EIC energies, focusing on (i) identifying the flavor of the jet and (ii) identifying the underlying hard process of the event. We propose applications of our machine learning-based jet identification in the key research areas at the future EIC and current Relativistic Heavy Ion Collider program, including enhancing constraints on (transverse momentum dependent) parton distribution functions, improving experimental access to transverse spin asymmetries, studying photon structure, and quantifying the modification of hadrons and jets in the cold nuclear matter environment in electron-nucleus collisions. We establish first benchmarks and contrast the estimated performance of flavor tagging at the EIC with that at the Large Hadron Collider. We perform studies relevant to aspects of detector design including particle identification, charge information, and minimum transverse momentum capabilities. Additionally, we study the impact of using full event information instead of using only information associated with the identified jet. These methods can be deployed either on suitably accurate Monte Carlo event generators, or, for several applications, directly on experimental data. We provide an outlook for ultimately connecting these machine learning-based methods with first principles calculations in quantum chromodynamics.
更多查看译文
关键词
Deep Inelastic Scattering or Small-x Physics,Jets and Jet Substructure,Parton Distributions,Properties of Hadrons
AI 理解论文
溯源树
样例
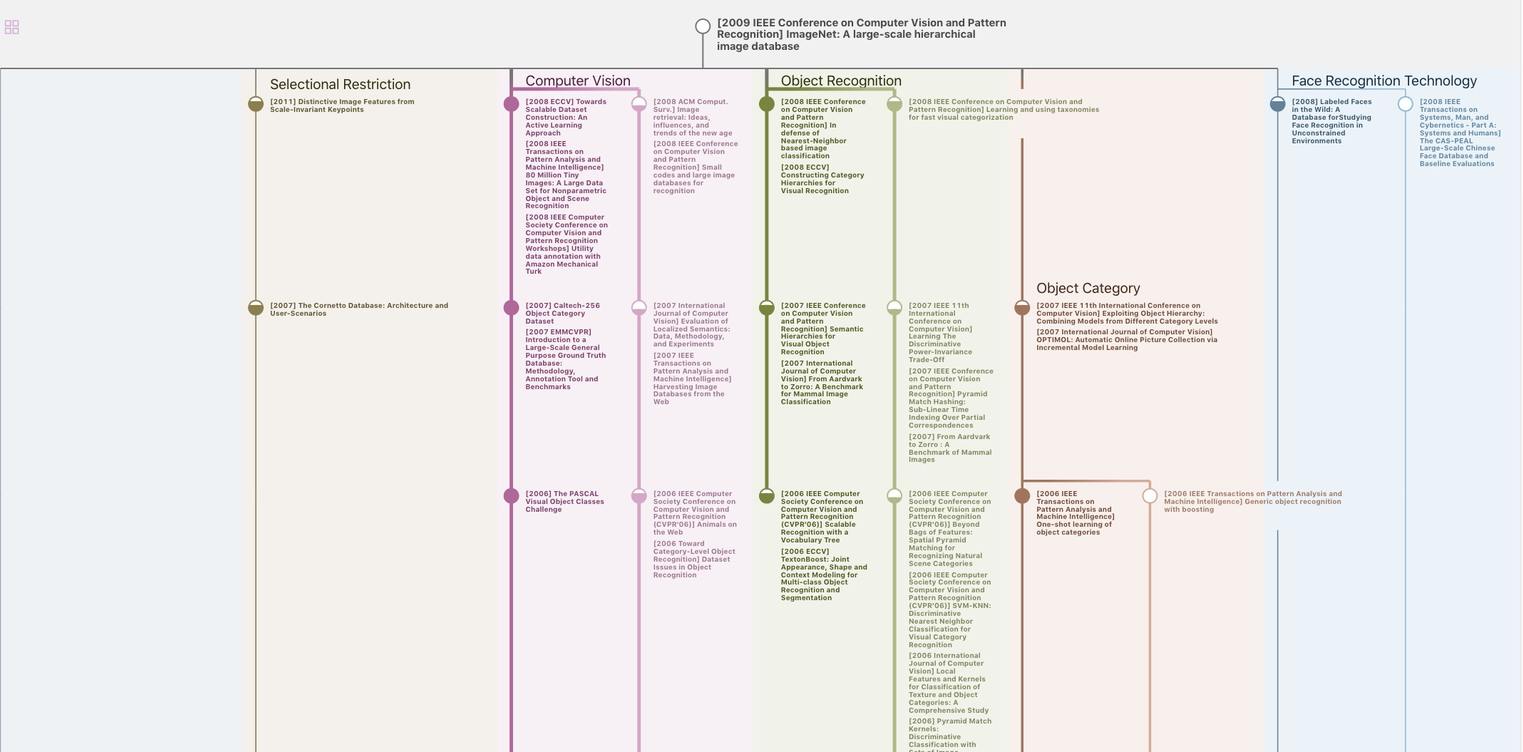
生成溯源树,研究论文发展脉络
Chat Paper
正在生成论文摘要