Incorporating Unlabelled Data into Bayesian Neural Networks.
Trans Mach Learn Res(2024)
摘要
Conventional Bayesian Neural Networks (BNNs) cannot leverage unlabelled data to improve their predictions. To overcome this limitation, we introduce Self-Supervised Bayesian Neural Networks, which use unlabelled data to learn improved prior predictive distributions by maximising an evidence lower bound during an unsupervised pre-training step. With a novel methodology developed to better understand prior predictive distributions, we then show that self-supervised prior predictives capture image semantics better than conventional BNN priors. In our empirical evaluations, we see that self-supervised BNNs offer the label efficiency of self-supervised methods and the uncertainty estimates of Bayesian methods, particularly outperforming conventional BNNs in low-to-medium data regimes.
更多查看译文
AI 理解论文
溯源树
样例
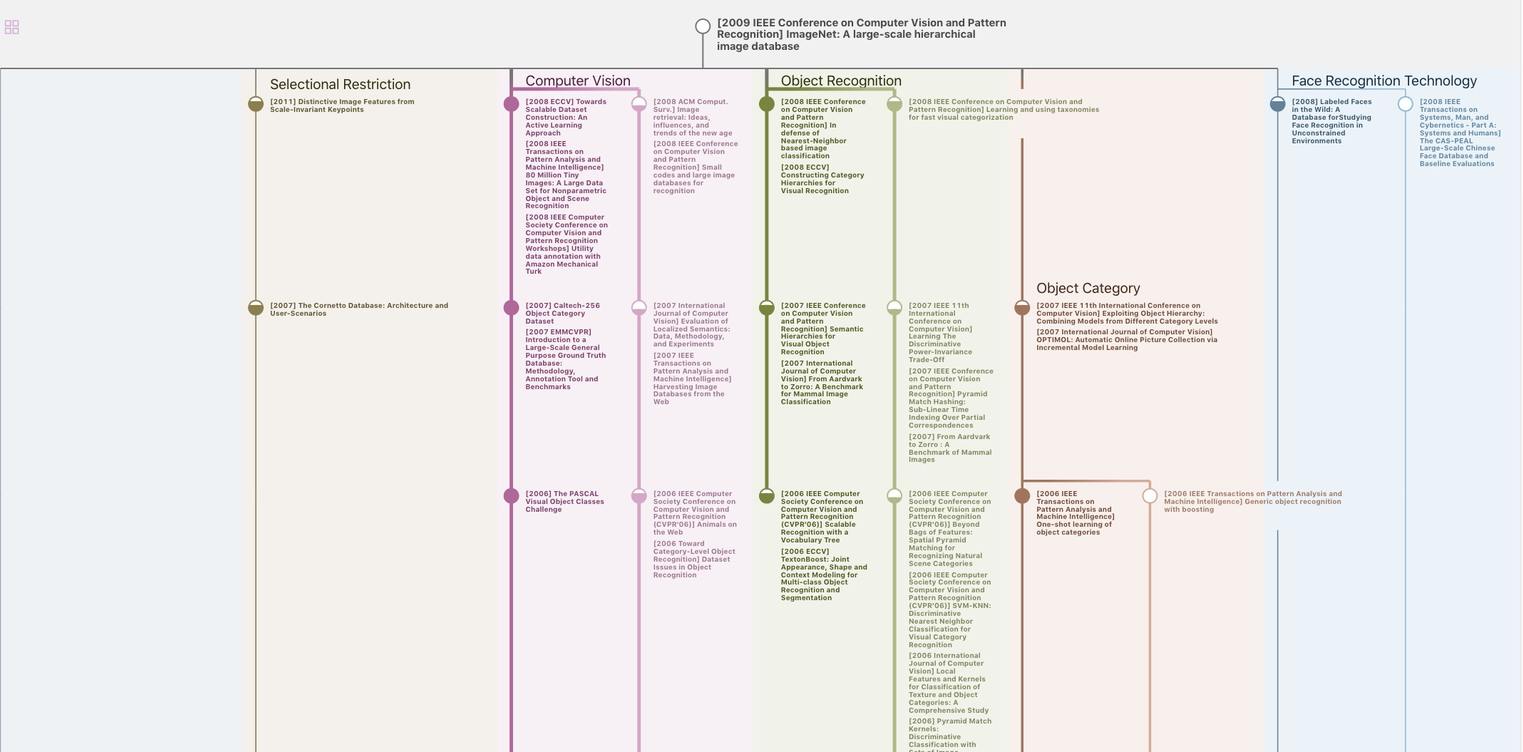
生成溯源树,研究论文发展脉络
Chat Paper
正在生成论文摘要