A Sample Based Method for Understanding The Decisions of Neural Networks Semantically
ICLR 2023(2023)
摘要
Interpretability in deep learning is one of the largest obstacles to its more widespread adoption in critical applications. A variety of methods have been introduced to understand and explain decisions made by Deep Models. A class of these methods highlights which features are most influential to model predictions. These methods have some key weaknesses. First, most of these methods are applicable only to the atomic elements that make up raw inputs to the model (e.g., pixels or words). Second, these methods generally don't distinguish between the importance of features individually versus due to interactions with other features. As a result, it is difficult to explore high level questions about how models use features. We tackle these issues by proposing Sample-Based Semantic Analysis (SBSA). We use Sobol sensitivity analysis as our sample-based method. Sobol-SBSA allows us to quantify the importance of semantic combinations of raw inputs and highlight the extent to which these features are important individually as opposed to due to interactions with other features. We demonstrate the ability of Sobol-SBSA to answer a richer class of questions about the behavior of Deep Learning models by exploring how CNN models from AlexNet to DenseNet use regions when classifying images. We present two key findings. 1) The architectural improvements from AlexNet to DenseNet manifested themselves in CNN models utilizing greater levels of region interactions for predictions. 2) Adversarially robust CNNs resist exploiting spurious correlations in ImageNet data by forcing these architectures to rely less on region-to-region interaction. Our proposed method is generalizable to a wide variety of network and input types and can help provide greater clarity about model decisions.
更多查看译文
关键词
Machine Learning Interpretability,Bias,ImageNet,AlexNet,ResNet,VGG-16,Inception,CNNs,Bag of Words
AI 理解论文
溯源树
样例
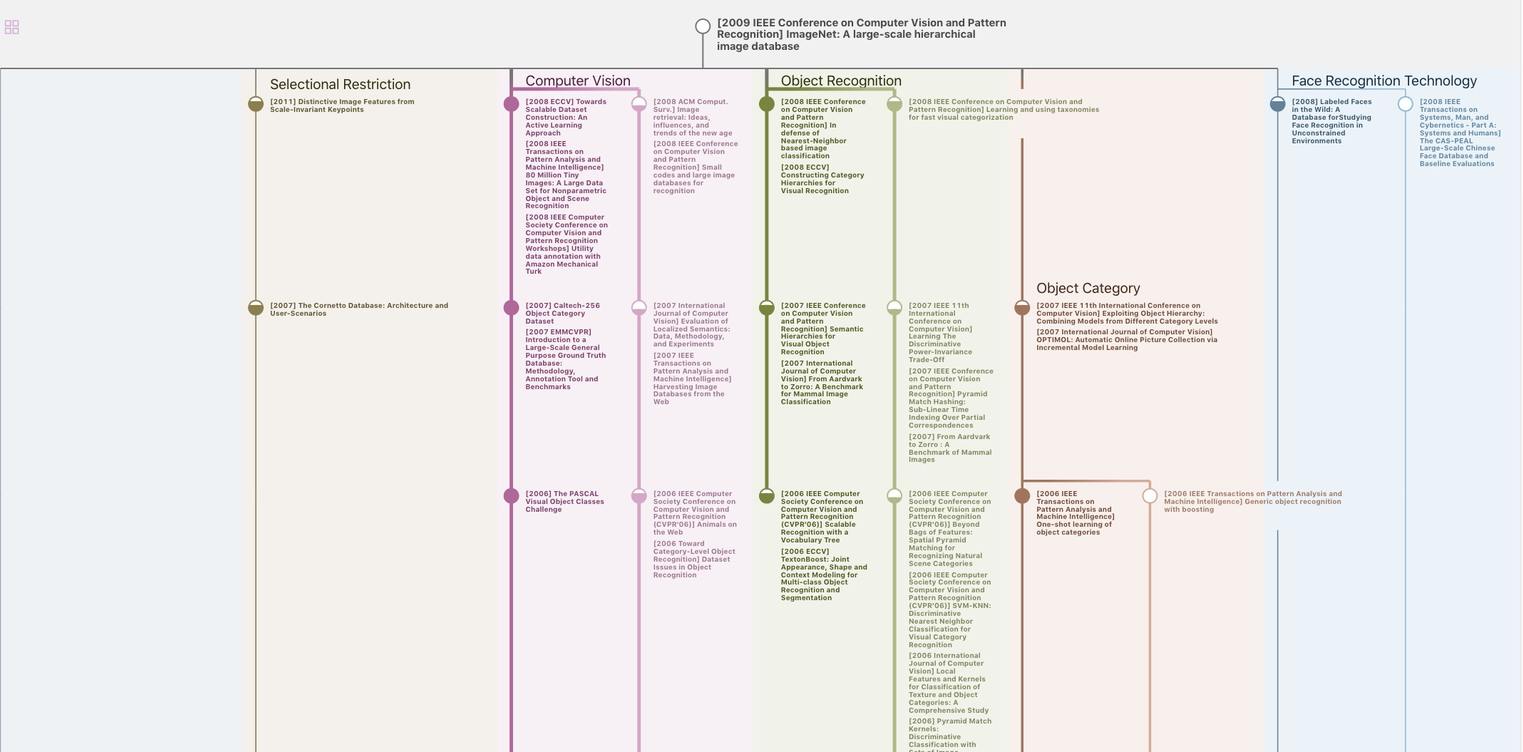
生成溯源树,研究论文发展脉络
Chat Paper
正在生成论文摘要