Augmenting Deep Learning Adaptation for Wearable Sensor Data through Combined Temporal-Frequency Image Encoding
CoRR(2023)
摘要
Deep learning advancements have revolutionized scalable classification in many domains including computer vision. However, when it comes to wearable-based classification and domain adaptation, existing computer vision-based deep learning architectures and pretrained models trained on thousands of labeled images for months fall short. This is primarily because wearable sensor data necessitates sensor-specific preprocessing, architectural modification, and extensive data collection. To overcome these challenges, researchers have proposed encoding of wearable temporal sensor data in images using recurrent plots. In this paper, we present a novel modified-recurrent plot-based image representation that seamlessly integrates both temporal and frequency domain information. Our approach incorporates an efficient Fourier transform-based frequency domain angular difference estimation scheme in conjunction with the existing temporal recurrent plot image. Furthermore, we employ mixup image augmentation to enhance the representation. We evaluate the proposed method using accelerometer-based activity recognition data and a pretrained ResNet model, and demonstrate its superior performance compared to existing approaches.
更多查看译文
关键词
deep learning adaptation,wearable sensor data,deep learning,temporal-frequency
AI 理解论文
溯源树
样例
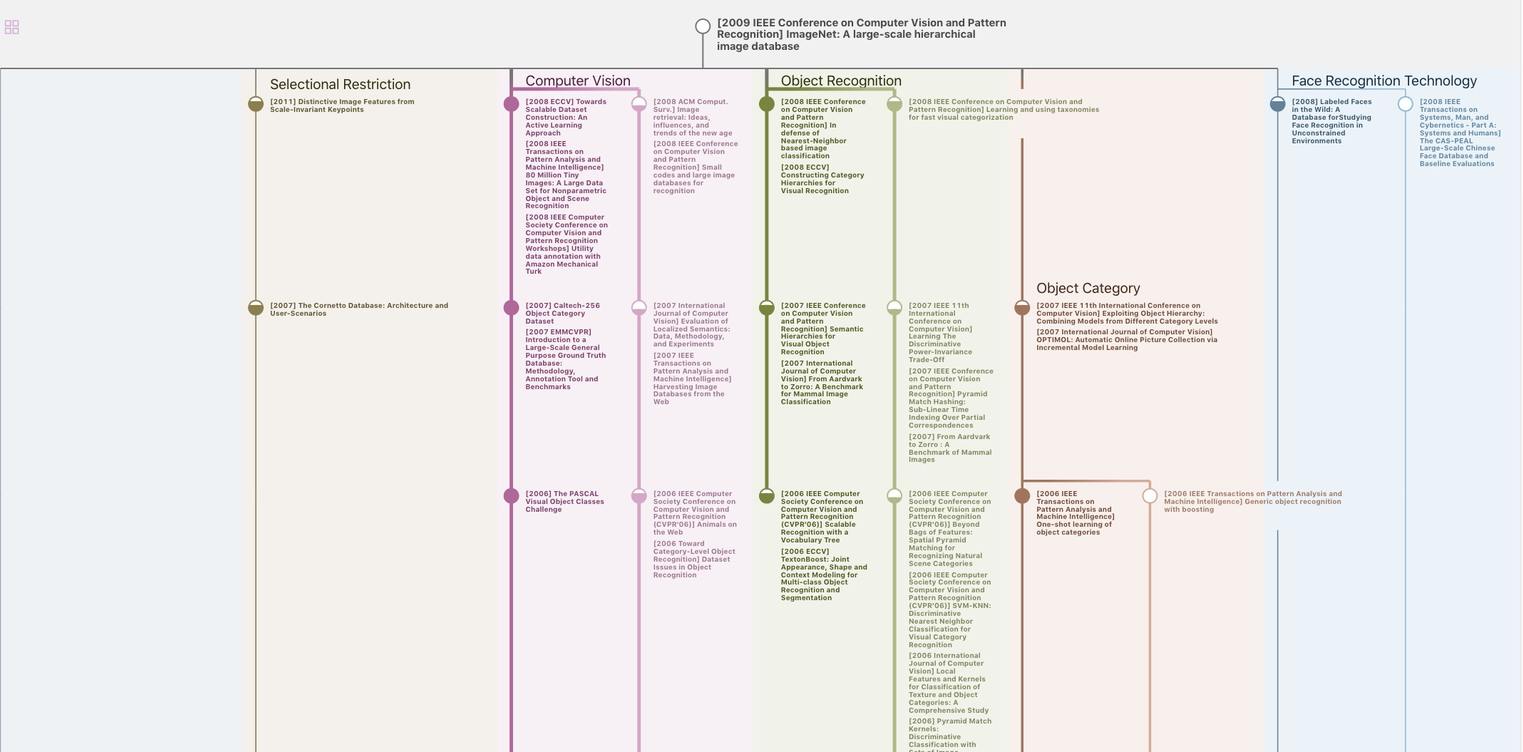
生成溯源树,研究论文发展脉络
Chat Paper
正在生成论文摘要