CoDeF: Content Deformation Fields for Temporally Consistent Video Processing
Computer Vision and Pattern Recognition(2024)
摘要
We present the content deformation field CoDeF as a new type of video representation, which consists of a canonical content field aggregating the static contents in the entire video and a temporal deformation field recording the transformations from the canonical image (i.e., rendered from the canonical content field) to each individual frame along the time axis.Given a target video, these two fields are jointly optimized to reconstruct it through a carefully tailored rendering pipeline.We advisedly introduce some regularizations into the optimization process, urging the canonical content field to inherit semantics (e.g., the object shape) from the video.With such a design, CoDeF naturally supports lifting image algorithms for video processing, in the sense that one can apply an image algorithm to the canonical image and effortlessly propagate the outcomes to the entire video with the aid of the temporal deformation field.We experimentally show that CoDeF is able to lift image-to-image translation to video-to-video translation and lift keypoint detection to keypoint tracking without any training.More importantly, thanks to our lifting strategy that deploys the algorithms on only one image, we achieve superior cross-frame consistency in processed videos compared to existing video-to-video translation approaches, and even manage to track non-rigid objects like water and smog.Project page can be found at https://qiuyu96.github.io/CoDeF/.
更多查看译文
关键词
Deformation Field,Temporal Consistency,Object Shape,Imaging Algorithm,Temporal Field,Image Processing,3D Space,Multilayer Perceptron,Diffusion Model,Natural Images,Video Frames,Semantic Segmentation,Complex Scenarios,Hash Function,Powerful Capability,Extensive Dataset,Video Content,Complex Motion,Corner Points,Video Editing,Implicit Representation
AI 理解论文
溯源树
样例
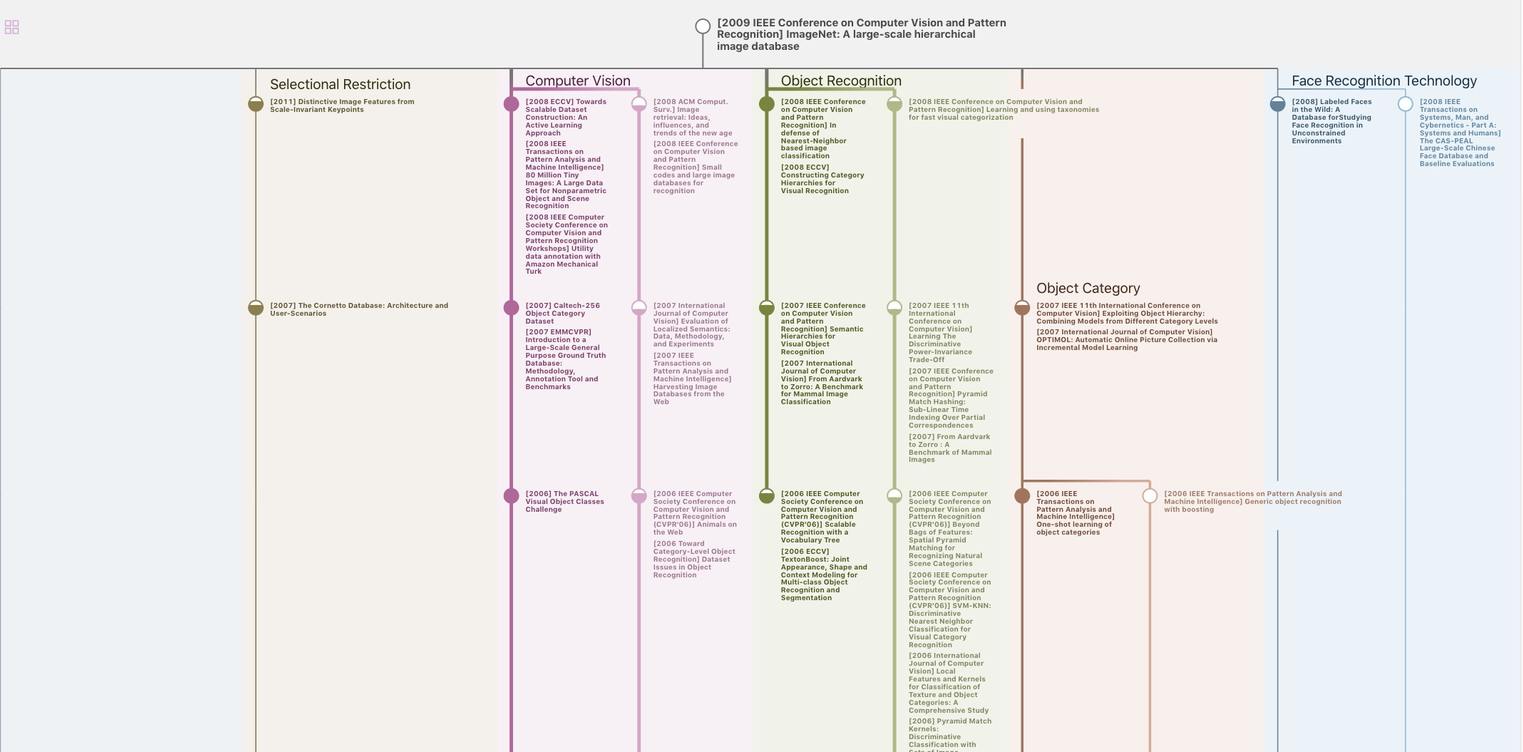
生成溯源树,研究论文发展脉络
Chat Paper
正在生成论文摘要