Video OWL-ViT: Temporally-consistent Open-World Localization in Video
arXivorg(2023)
摘要
We present an architecture and a training recipe that adapts pre-trained open-world image models to localization in videos. Understanding the open visual world (without being constrained by fixed label spaces) is crucial for many real-world vision tasks. Contrastive pre-training on large image-text datasets has recently led to significant improvements for image-level tasks. For more structured tasks involving object localization applying pre-trained models is more challenging. This is particularly true for video tasks, where task-specific data is limited. We show successful transfer of open-world models by building on the OWL-ViT open-vocabulary detection model and adapting it to video by adding a transformer decoder. The decoder propagates object representations recurrently through time by using the output tokens for one frame as the object queries for the next. Our model is end-to-end trainable on video data and enjoys improved temporal consistency compared to tracking-by-detection baselines, while retaining the open-world capabilities of the backbone detector. We evaluate our model on the challenging TAO-OW benchmark and demonstrate that open-world capabilities, learned from large-scale image-text pre-training, can be transferred successfully to open-world localization across diverse videos.
更多查看译文
关键词
Object Detection,Transfer Learning,Visual Question Answering,Meta-Learning,Visual Recognition
AI 理解论文
溯源树
样例
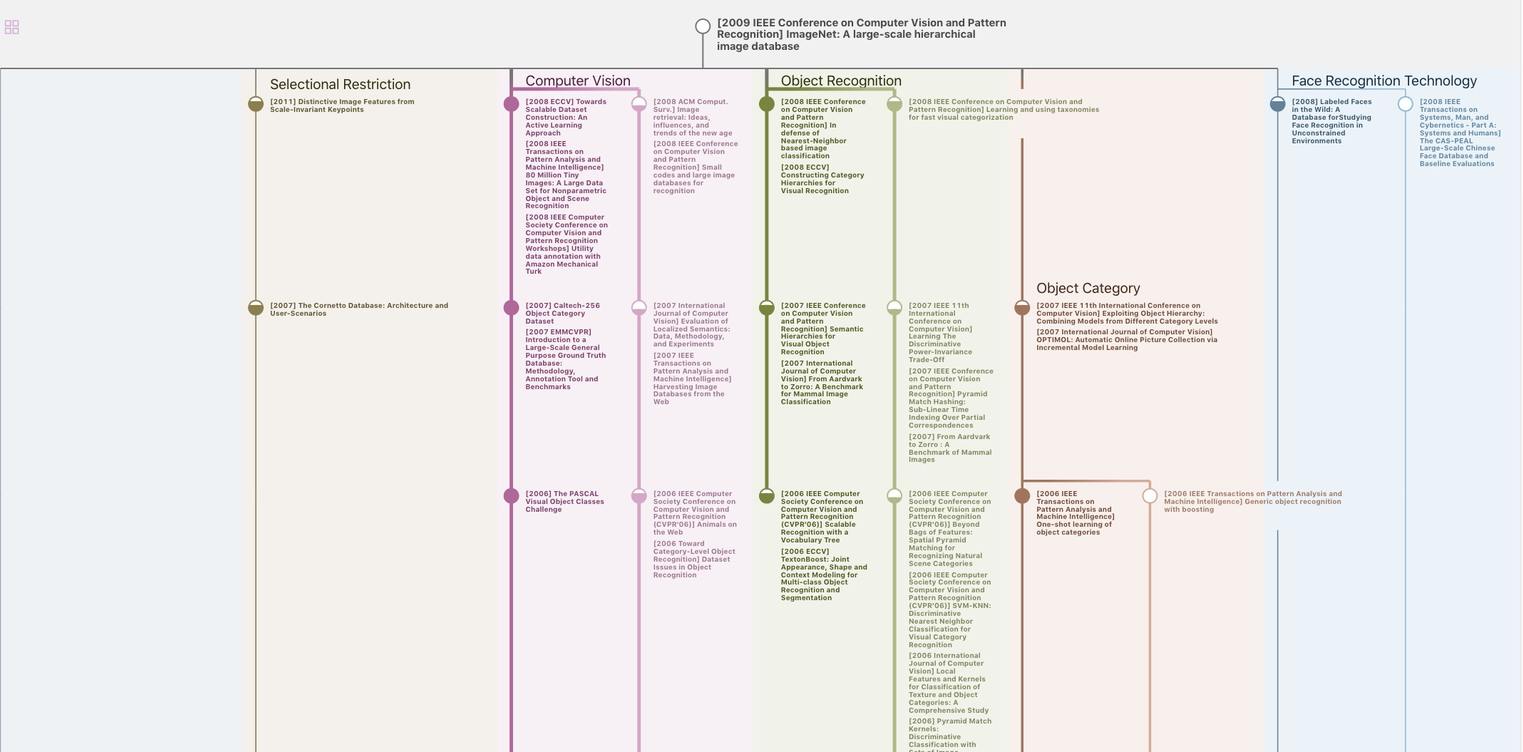
生成溯源树,研究论文发展脉络
Chat Paper
正在生成论文摘要