Interpretability and Fairness in Machine Learning: A Formal Methods Approach.
IJCAI 2023(2023)
摘要
The last decades have witnessed significant progress in machine learning with a host of applications of algorithmic decision-making in different safety-critical domains, such as medical, law, education, and transportation. In high-stake domains, machine learning predictions have far-reaching consequences on the end-users. With the aim of applying machine learning for societal goods, there have been increasing efforts to regulate machine learning by imposing interpretability, fairness, robustness, etc. in predictions. Towards responsible and trustworthy machine learning, we propose two research themes in our dissertation research: interpretability and fairness of machine learning classifiers. In particular, we design algorithms to learn interpretable rule-based classifiers, formally verify fairness, and explain the sources of unfairness. Prior approaches to these problems are often limited by scalability, accuracy, or both. To overcome these limitations, we closely integrate automated reasoning, formal methods, and statistics with fairness and interpretability to develop scalable and accurate solutions.
更多查看译文
关键词
General: General,AI Ethics, Trust, Fairness: ETF: Explainability and interpretability,AI Ethics, Trust, Fairness: ETF: Fairness and diversity
AI 理解论文
溯源树
样例
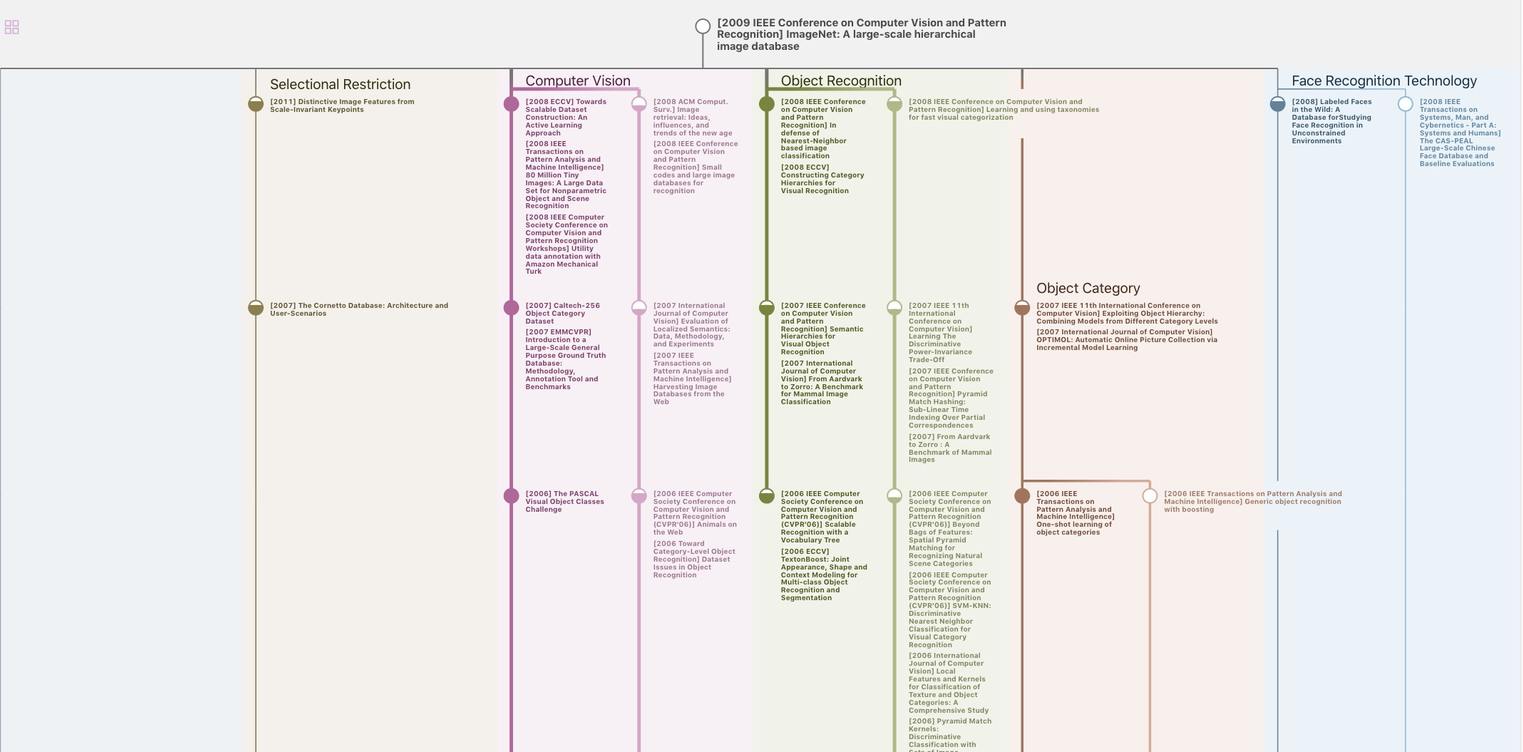
生成溯源树,研究论文发展脉络
Chat Paper
正在生成论文摘要