Towards Universal Image Embeddings: A Large-Scale Dataset and Challenge for Generic Image Representations
2023 IEEE/CVF International Conference on Computer Vision (ICCV)(2023)
摘要
Fine-grained and instance-level recognition methods are commonly trained and evaluated on specific domains, in a model per domain scenario. Such an approach, however, is impractical in real large-scale applications. In this work, we address the problem of universal image embedding, where a single universal model is trained and used in multiple domains. First, we leverage existing domain-specific datasets to carefully construct a new large-scale public benchmark for the evaluation of universal image embeddings, with 241k query images, 1.4M index images and 2.8M training images across 8 different domains and 349k classes. We define suitable metrics, training and evaluation protocols to foster future research in this area. Second, we provide a comprehensive experimental evaluation on the new dataset, demonstrating that existing approaches and simplistic extensions lead to worse performance than an assembly of models trained for each domain separately. Finally, we conducted a public research competition on this topic, leveraging industrial datasets, which attracted the participation of more than 1k teams worldwide. This exercise generated many interesting research ideas and findings which we present in detail. Project webpage: https://cmp.felk.cvut.cz/univ_emb/
更多查看译文
关键词
Representation Learning,Transfer Learning,Visual Recognition,Medical Imaging,Image Captioning
AI 理解论文
溯源树
样例
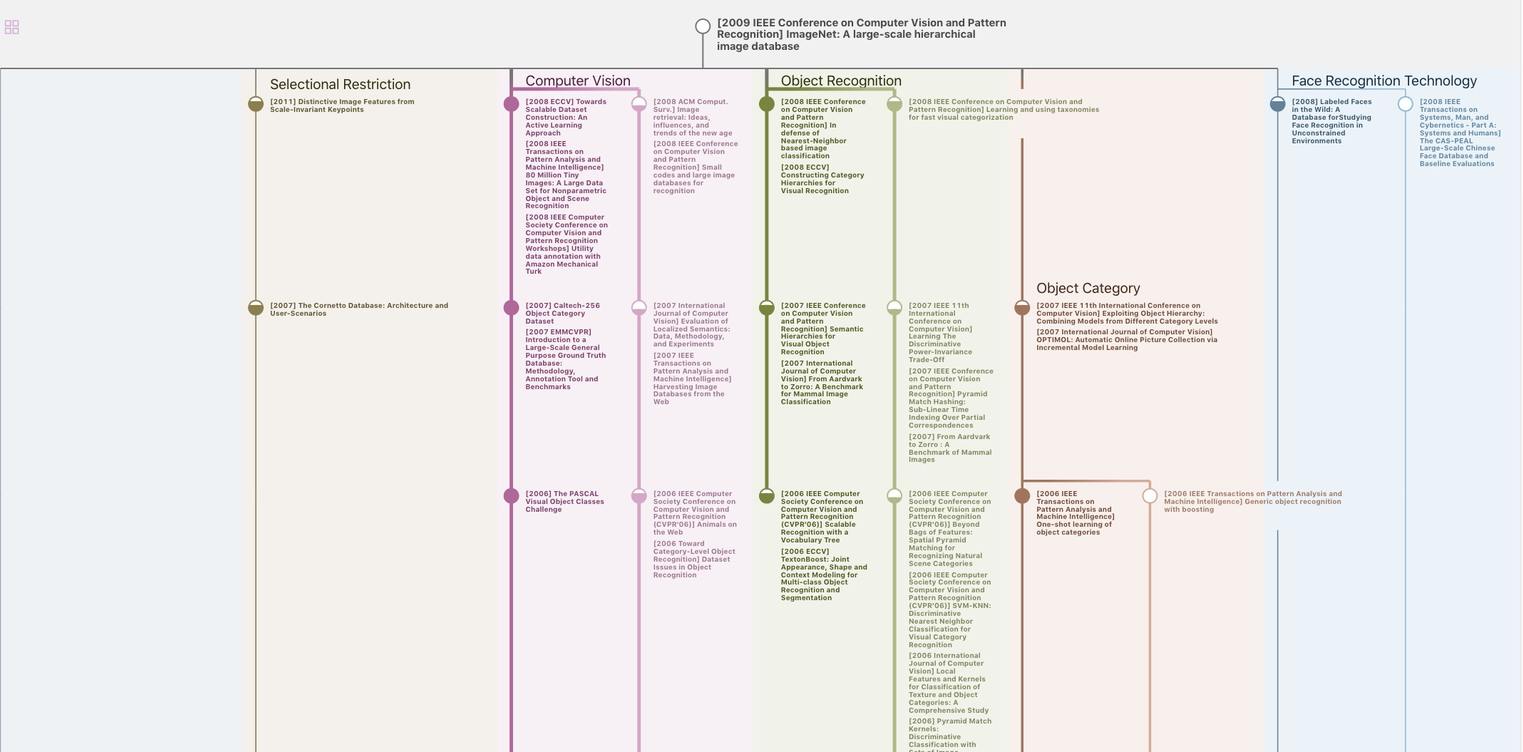
生成溯源树,研究论文发展脉络
Chat Paper
正在生成论文摘要