Cudabrkga-Cnn: an Approach for Optimizing Convolutional Neural Network Architectures
2023 IEEE Congress on Evolutionary Computation (CEC)(2023)
摘要
This paper proposes an approach based on the Biased Random-Key Genetic Algorithm (BRKGA) metaheuristic to optimize hyperparameters of Convolutional Neural Network (CNN) architectures. We developed a Default version for computers that only have access to Central Processing Units (CPUs) and a version for Graphics Processing Units (GPUs) called cudaBRKGA-CNN, guaranteeing better computational performance during CNN training. Additionally, we developed a decoder that searches for CNN hyperparameters and returns competitive solutions. We compared the performance of our two proposed approaches with other state-of-the-art deep learning models using two datasets from the literature. Our approaches demonstrated high performance in terms of solution quality and convergence speed. Furthermore, our cudaBRKGA-CNN proposal presented competitive results and better computational time than the other evaluated models.
更多查看译文
关键词
BRKGA,CNN,hyperparameters,optimization
AI 理解论文
溯源树
样例
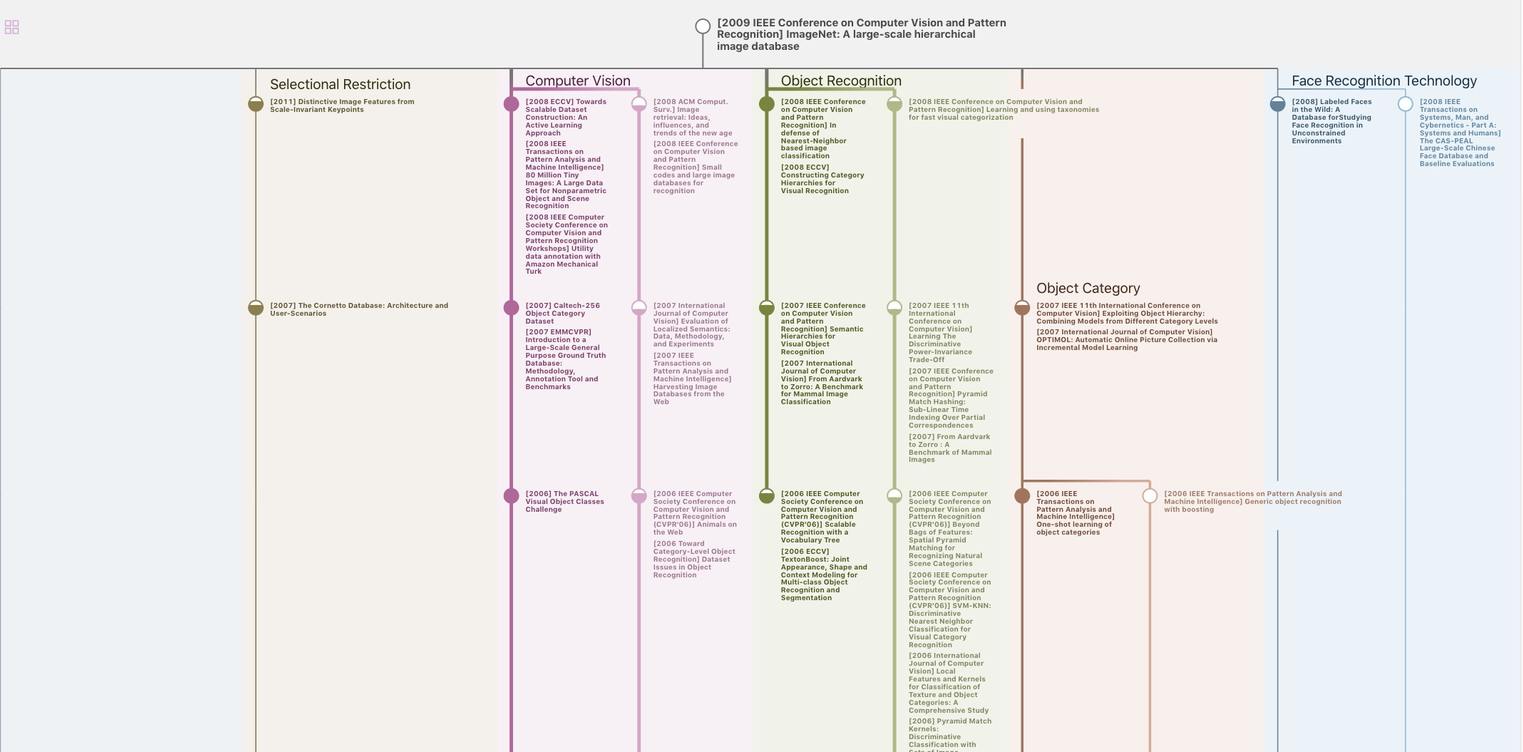
生成溯源树,研究论文发展脉络
Chat Paper
正在生成论文摘要