Event-Enhanced Multi-Modal Spiking Neural Network for Dynamic Obstacle Avoidance
Proceedings of the 31st ACM International Conference on Multimedia(2023)
摘要
Autonomous obstacle avoidance is of vital importance for an intelligent agent such as a mobile robot to navigate in its environment. Existing state-of-the-art methods train a spiking neural network (SNN) with deep reinforcement learning (DRL) to achieve energy-efficient and fast inference speed in complex/unknown scenes. These methods typically assume that the environment is static while the obstacles in real-world scenes are often dynamic. The movement of obstacles increases the complexity of the environment and poses a great challenge to the existing methods. In this work, we approach robust dynamic obstacle avoidance twofold. First, we introduce the neuromorphic vision sensor (i.e., event camera) to provide motion cues complementary to the traditional Laser depth data for handling dynamic obstacles. Second, we develop an DRL-based event-enhanced multimodal spiking actor network (EEM-SAN) that extracts information from motion events data via unsupervised representation learning and fuses Laser and event camera data with learnable thresholding. Experiments demonstrate that our EEM-SAN outperforms state-of-the-art obstacle avoidance methods by a significant margin, especially for dynamic obstacle avoidance.
更多查看译文
关键词
Dynamic Vision Sensor (DVS),Spiking Neural Network (SNN),Deep Reinforcement Learning (DRL),dynamic obstacle avoidance
AI 理解论文
溯源树
样例
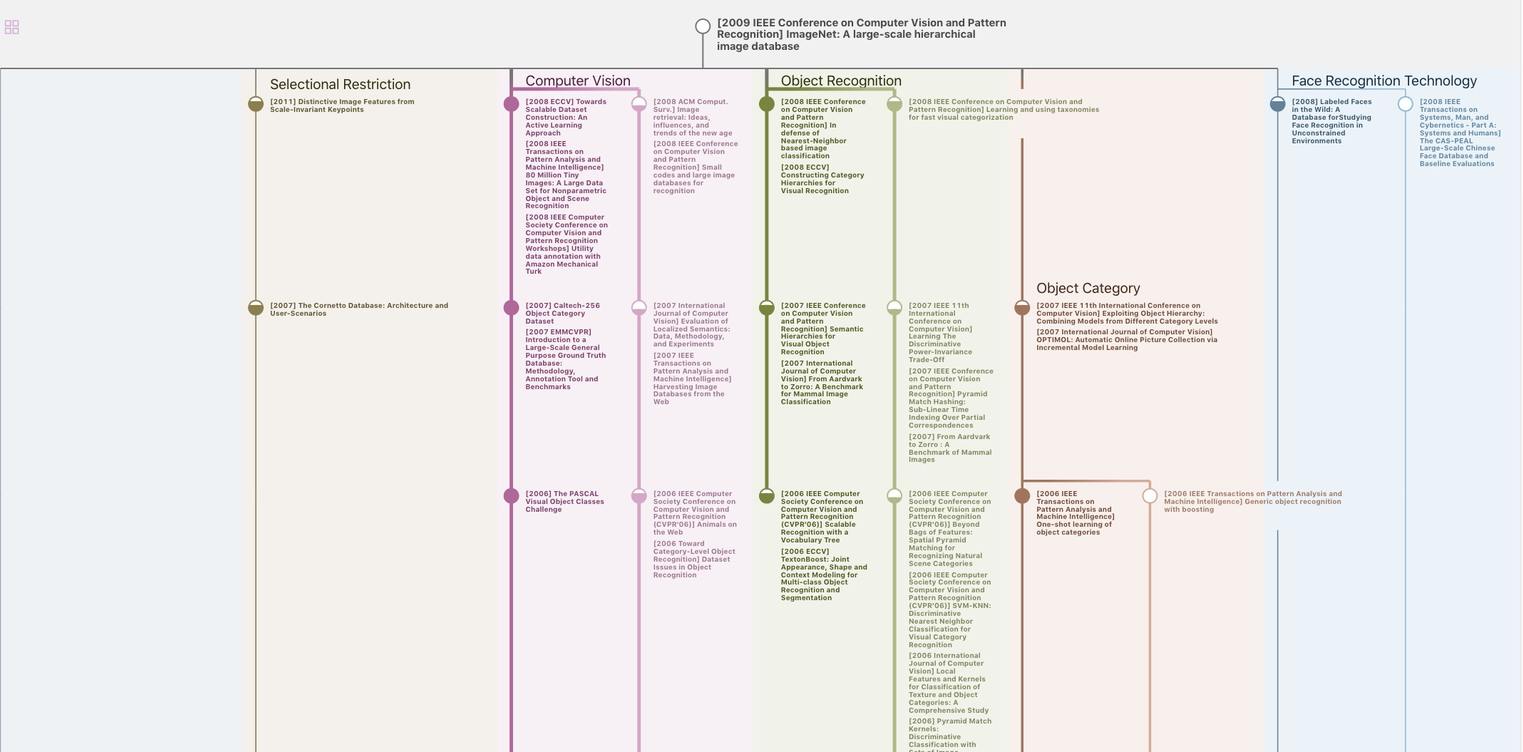
生成溯源树,研究论文发展脉络
Chat Paper
正在生成论文摘要