Learning Implicit Sentiment for Explainable Review-Based Recommendation
DATABASES THEORY AND APPLICATIONS, ADC 2023(2024)
摘要
Users can publish reviews to express their detailed feelings about the items. Positive and negative sentiments about various aspects of an item co-existing in the same review may cause confusion in recommendations and generate inappropriate explanations. However, most current explainable recommendation methods fail to capture users’ implicit sentiment behind the reviews. In this paper, we propose a novel Implicit Sentiment learning model for Explainable review-based Recommendation, named ISER, which learns users’ implicit sentiments from reviews and explores them to generate recommendations with more fine-grained explanations. Specifically, we first propose a novel representation learning to model users/items based on the implicit sentiment behind the reviews. Then we propose two implicit sentiment fusion strategies for rating prediction and explanation generation respectively. Finally, we propose a multi-task learning framework to jointly optimize the rating prediction task and the explanation generation task, which improves the recommendation quality in a mutual promotion manner. The experiments demonstrate the effectiveness and efficiency of our proposed model compared to the baseline models.
更多查看译文
关键词
Explainable recommendation,Implicit sentiment learning,Fusion strategy,Multi-task learning
AI 理解论文
溯源树
样例
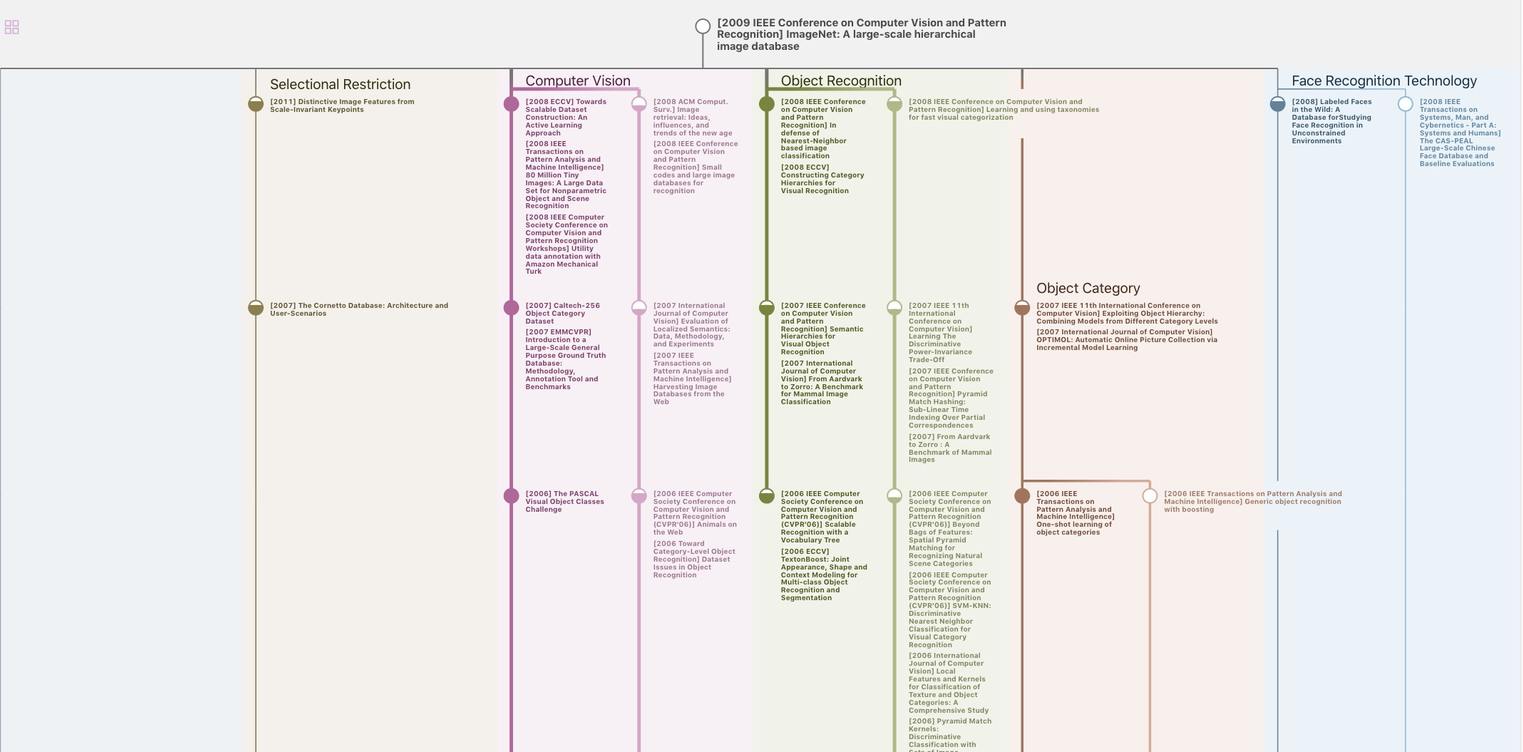
生成溯源树,研究论文发展脉络
Chat Paper
正在生成论文摘要