Action-slot: Visual Action-centric Representations for Multi-label Atomic Activity Recognition in Traffic Scenes
Computer Vision and Pattern Recognition(2024)
摘要
In this paper, we study multi-label atomic activity recognition. Despite thenotable progress in action recognition, it is still challenging to recognizeatomic activities due to a deficiency in a holistic understanding of bothmultiple road users' motions and their contextual information. In this paper,we introduce Action-slot, a slot attention-based approach that learns visualaction-centric representations, capturing both motion and contextualinformation. Our key idea is to design action slots that are capable of payingattention to regions where atomic activities occur, without the need forexplicit perception guidance. To further enhance slot attention, we introduce abackground slot that competes with action slots, aiding the training process inavoiding unnecessary focus on background regions devoid of activities. Yet, theimbalanced class distribution in the existing dataset hampers the assessment ofrare activities. To address the limitation, we collect a synthetic datasetcalled TACO, which is four times larger than OATS and features a balanceddistribution of atomic activities. To validate the effectiveness of our method,we conduct comprehensive experiments and ablation studies against variousaction recognition baselines. We also show that the performance of multi-labelatomic activity recognition on real-world datasets can be improved bypretraining representations on TACO. We will release our source code anddataset. See the videos of visualization on the project page:https://hcis-lab.github.io/Action-slot/
更多查看译文
关键词
Action Recognition,Slot Attention,Video Understanding,Traffic Activity,Autonomous Driving
AI 理解论文
溯源树
样例
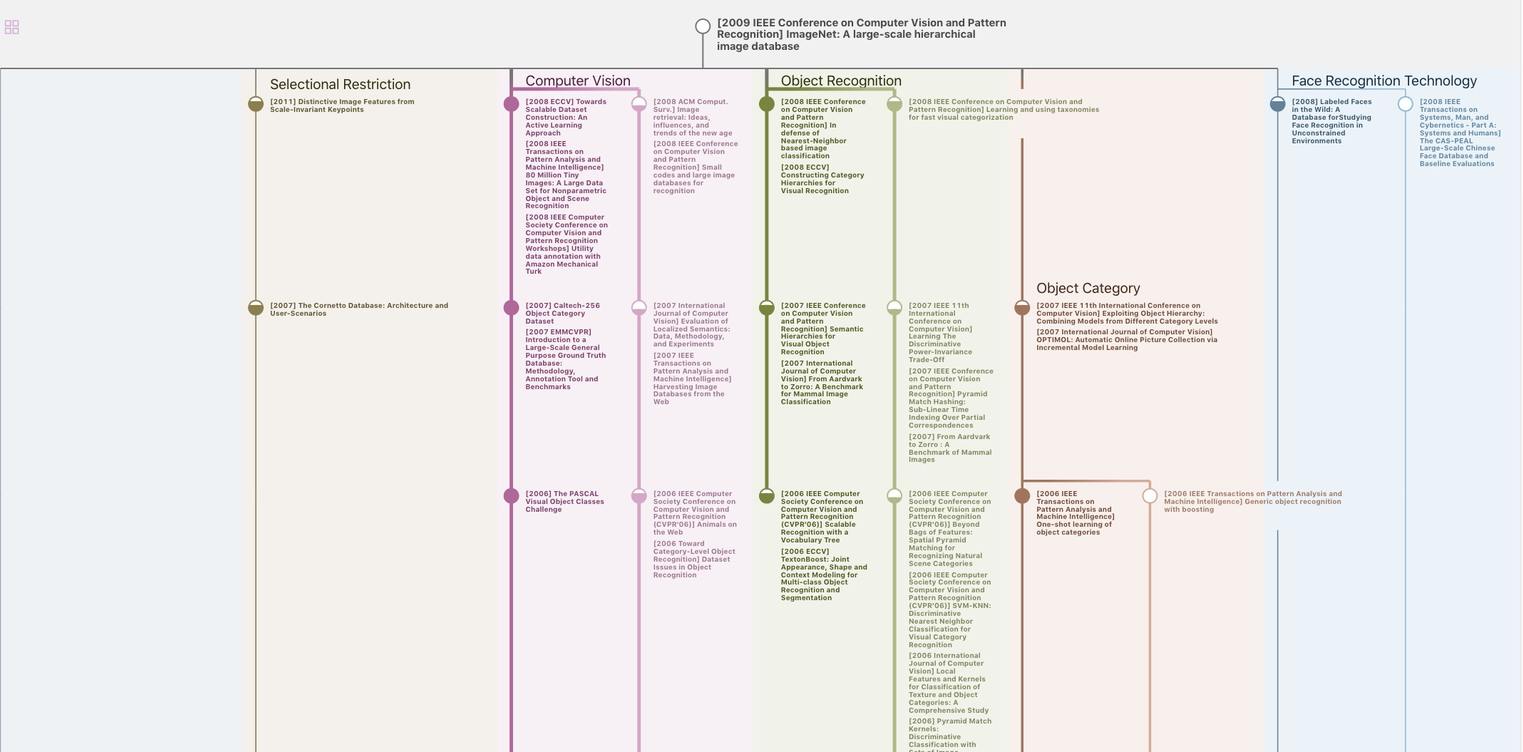
生成溯源树,研究论文发展脉络
Chat Paper
正在生成论文摘要