Deep Born Operator Learning for Reflection Tomographic Imaging.
ICASSP(2023)
摘要
Recent developments in wave-based sensor technologies, such as ground penetrating radar (GPR), provide new opportunities for accurate imaging of underground scenes. Given measurements of the scattered electromagnetic wavefield, the goal is to estimate the spatial distribution of the permittivity of the underground scenes. However, such problems are highly ill-posed, difficult to formulate, and computationally expensive. In this paper, we propose a physics-inspired machine learning-based method to learn the wave-matter interaction under the GPR setting. The learned forward model is combined with a learned signal prior to recover the permittivity distribution of the unknown underground scenes. We test our approach on a dataset of 400 permittivity maps with a three-layer background, which is challenging to solve using existing methods. We demonstrate via numerical simulation that our method achieves a 50% improvement in mean squared error over benchmark machine learning-based solvers for reconstructing layered underground scenes.
更多查看译文
关键词
Underground Imaging,Diffraction Tomography,Full-waveform Inversion
AI 理解论文
溯源树
样例
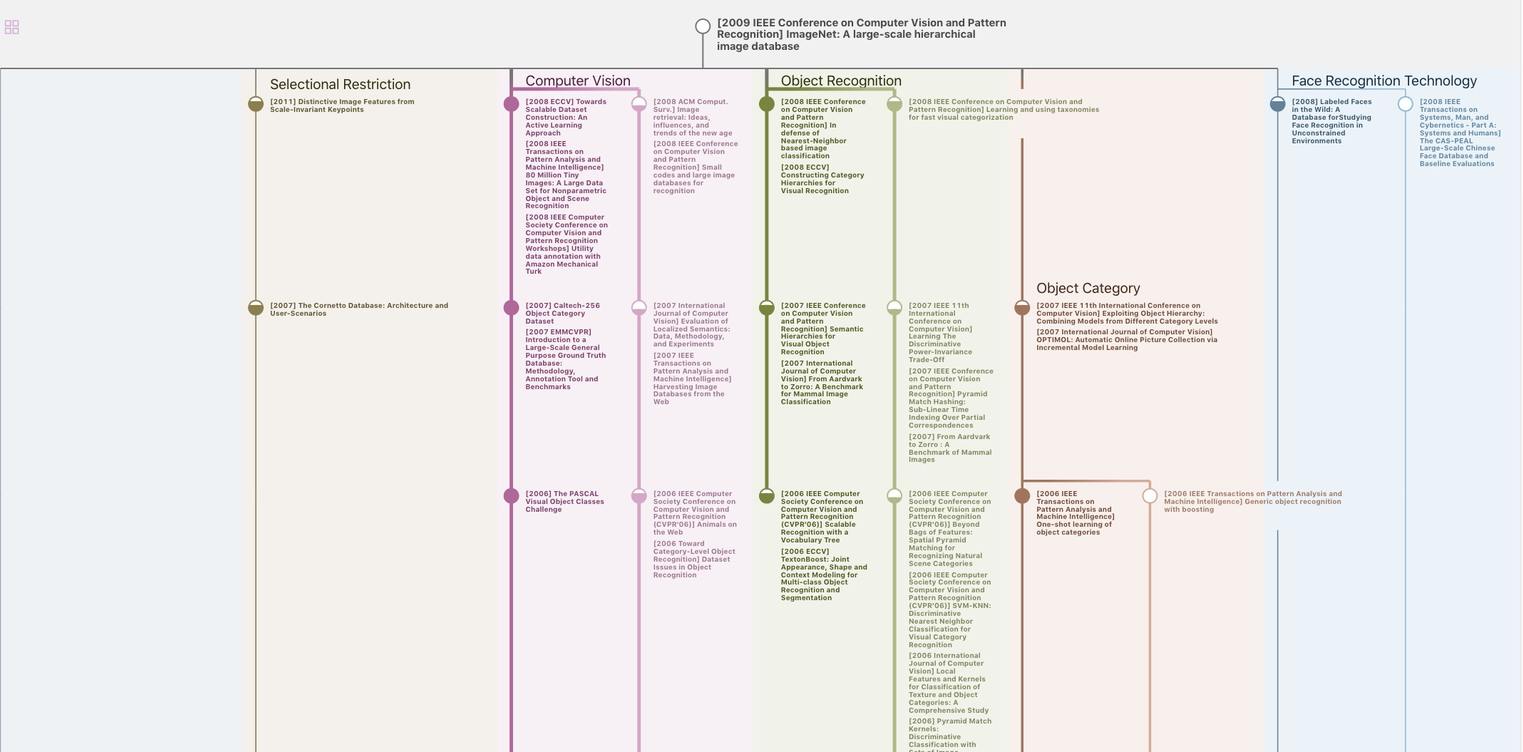
生成溯源树,研究论文发展脉络
Chat Paper
正在生成论文摘要