Moving Horizon Estimation for Digital Twins Using Deep Autoencoders
IFAC-PapersOnLine(2023)
摘要
Digital twins have emerged in recent years as black-box, software-based simulation tools that can mirror the behavior of complex dynamical systems. Digital twin simulations generate the same outputs as the target system using internal states; however, these states are not readily available online from the real system. In this paper, we develop a data-driven moving horizon estimation framework capable of using online noisy measurements of the real system in order to estimate digital twin states. Our framework combines the high expressiveness of deep autoencoders with a moving horizon state estimator that accurately predicts the internal state of the black-box digital twin without access to an analytical model of the system dynamics. We demonstrate that our approach outperforms extended and Koopman Kalman filter solutions on a benchmark reverse van der Pol oscillator example.
更多查看译文
关键词
Learning,state observers,nonlinear systems,Koopman operator,system identification,black-box models
AI 理解论文
溯源树
样例
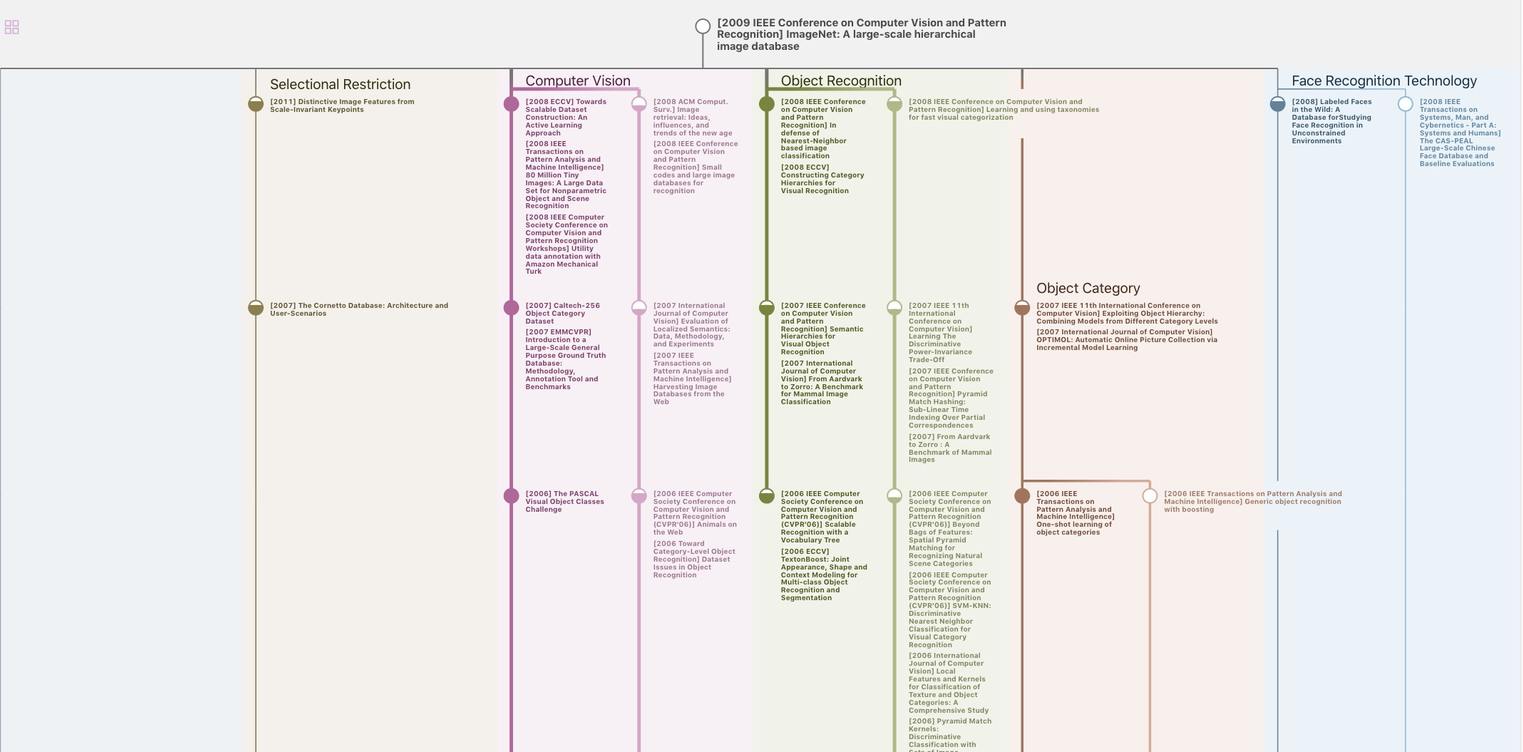
生成溯源树,研究论文发展脉络
Chat Paper
正在生成论文摘要