Modeling Structure and Country-specific Heterogeneity in Misclassification Matrices of Verbal Autopsy-based Cause of Death Classifiers
arxiv(2023)
Abstract
Verbal autopsy (VA) algorithms are routinely used to determine
individual-level causes of death (COD) in many low-and-middle-income countries,
which are then aggregated to derive population-level cause-specific mortality
fractions (CSMF), essential to informing public health policies. However, VA
algorithms frequently misclassify COD and introduce bias in CSMF estimates. A
recent method, VA-calibration, can correct for this bias using a VA
misclassification matrix estimated from paired data on COD from both VA and
minimally invasive tissue sampling (MITS) from the Child Health and Mortality
Prevention Surveillance (CHAMPS) Network. Due to the limited sample size,
CHAMPS data are pooled across all countries, implicitly assuming that the
misclassification rates are homogeneous.
In this research, we show that the VA misclassification matrices are
substantially heterogeneous across countries, thereby biasing the
VA-calibration. We develop a coherent framework for modeling country-specific
misclassification matrices in data-scarce settings. We first introduce a novel
base model based on two latent mechanisms: intrinsic accuracy and systematic
preference to parsimoniously characterize misclassifications. We prove that
they are identifiable from the data and manifest as a form of invariance in
certain misclassification odds, a pattern evident in the CHAMPS data. Then we
expand from this base model, adding higher complexity and country-specific
heterogeneity via interpretable effect sizes. Shrinkage priors balance the
bias-variance tradeoff by adaptively favoring simpler models. We publish
uncertainty-quantified estimates of VA misclassification rates for 6 countries.
This effort broadens VA-calibration's future applicability and strengthens
ongoing efforts of using VA for mortality surveillance.
MoreTranslated text
AI Read Science
Must-Reading Tree
Example
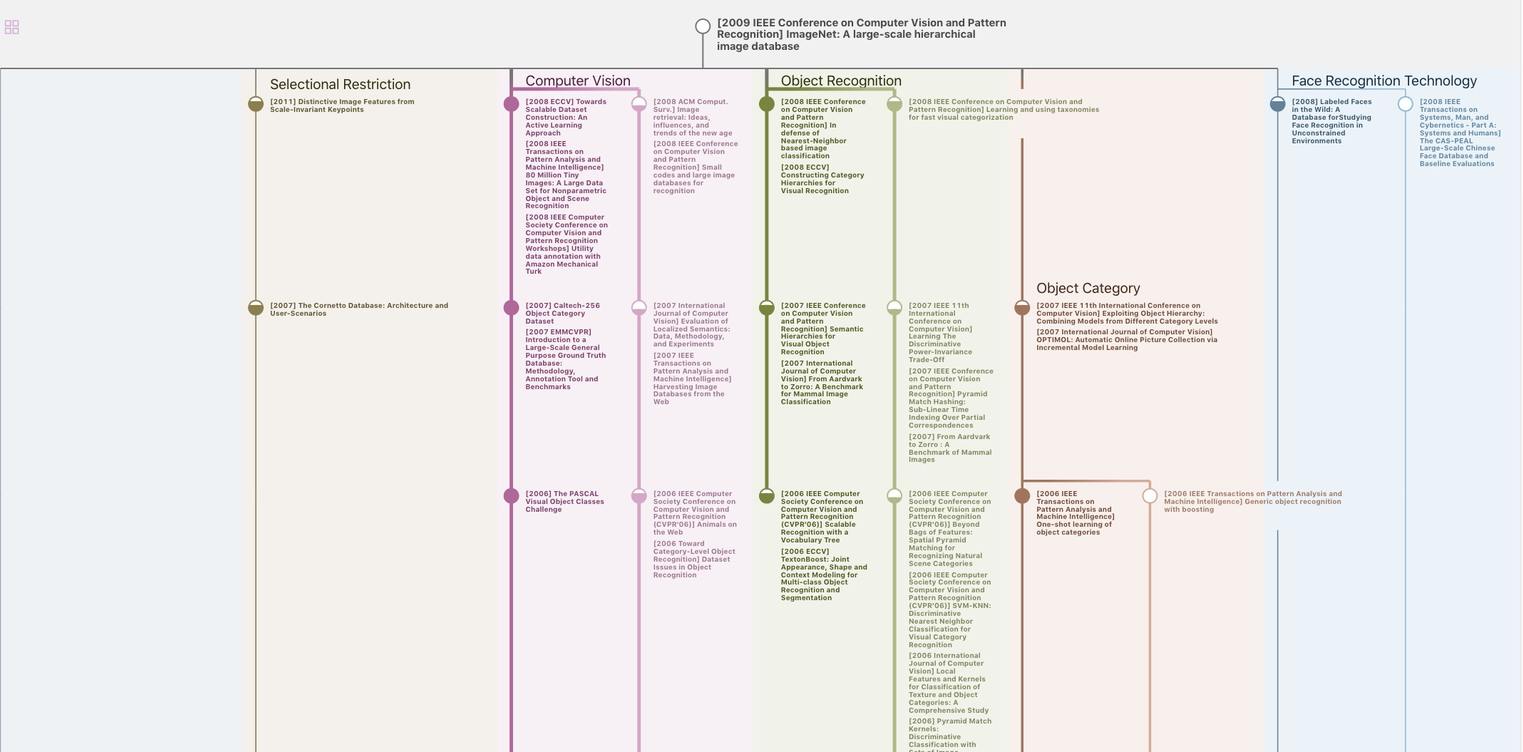
Generate MRT to find the research sequence of this paper
Chat Paper
Summary is being generated by the instructions you defined