A Probabilistic Framework for Task-Aligned Intra- and Inter-Area Neural Manifold Estimation
arXiv (Cornell University)(2022)
摘要
Latent manifolds provide a compact characterization of neural population activity and of shared co-variability across brain areas. Nonetheless, existing statistical tools for extracting neural manifolds face limitations in terms of interpretability of latents with respect to task variables, and can be hard to apply to datasets with no trial repeats. Here we propose a novel probabilistic framework that allows for interpretable partitioning of population variability within and across areas in the context of naturalistic behavior. Our approach for task aligned manifold estimation (TAME-GP) extends a probabilistic variant of demixed PCA by (1) explicitly partitioning variability into private and shared sources, (2) using a Poisson noise model, and (3) introducing temporal smoothing of latent trajectories in the form of a Gaussian Process prior. This TAME-GP graphical model allows for robust estimation of task-relevant variability in local population responses, and of shared co-variability between brain areas. We demonstrate the efficiency of our estimator on within model and biologically motivated simulated data. We also apply it to neural recordings in a closed-loop virtual navigation task in monkeys, demonstrating the capacity of TAME-GP to capture meaningful intra- and inter-area neural variability with single trial resolution.
更多查看译文
关键词
Working Memory,Neuroimaging Data Analysis,Cortical Parcellation,Neural Ensemble Physiology,Brain Network Development
AI 理解论文
溯源树
样例
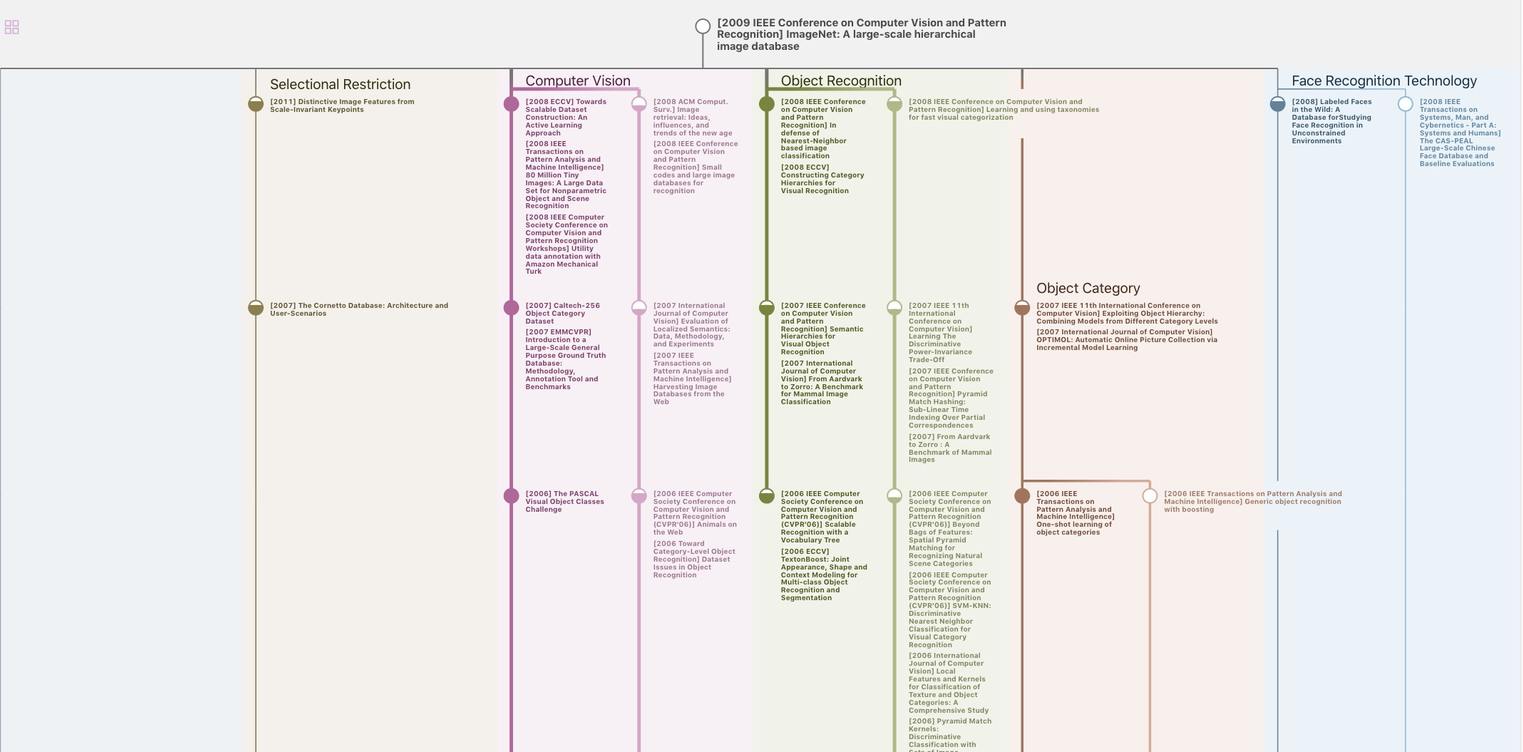
生成溯源树,研究论文发展脉络
Chat Paper
正在生成论文摘要