CBBQ: A Chinese Bias Benchmark Dataset Curated with Human-AI Collaboration for Large Language Models
arXiv (Cornell University)(2023)
摘要
Holistically measuring societal biases of large language models is crucial for detecting and reducing ethical risks in highly capable AI models. In this work, we present a Chinese Bias Benchmark dataset that consists of over 100K questions jointly constructed by human experts and generative language models, covering stereotypes and societal biases in 14 social dimensions related to Chinese culture and values. The curation process contains 4 essential steps: bias identification via extensive literature review, ambiguous context generation, AI-assisted disambiguous context generation, snd manual review \& recomposition. The testing instances in the dataset are automatically derived from 3K+ high-quality templates manually authored with stringent quality control. The dataset exhibits wide coverage and high diversity. Extensive experiments demonstrate the effectiveness of the dataset in detecting model bias, with all 10 publicly available Chinese large language models exhibiting strong bias in certain categories. Additionally, we observe from our experiments that fine-tuned models could, to a certain extent, heed instructions and avoid generating outputs that are morally harmful in some types, in the way of "moral self-correction". Our dataset and results are publicly available at \href{https://github.com/YFHuangxxxx/CBBQ}{https://github.com/YFHuangxxxx/CBBQ}, offering debiasing research opportunities to a widened community.
更多查看译文
关键词
Topic Modeling,Language Modeling
AI 理解论文
溯源树
样例
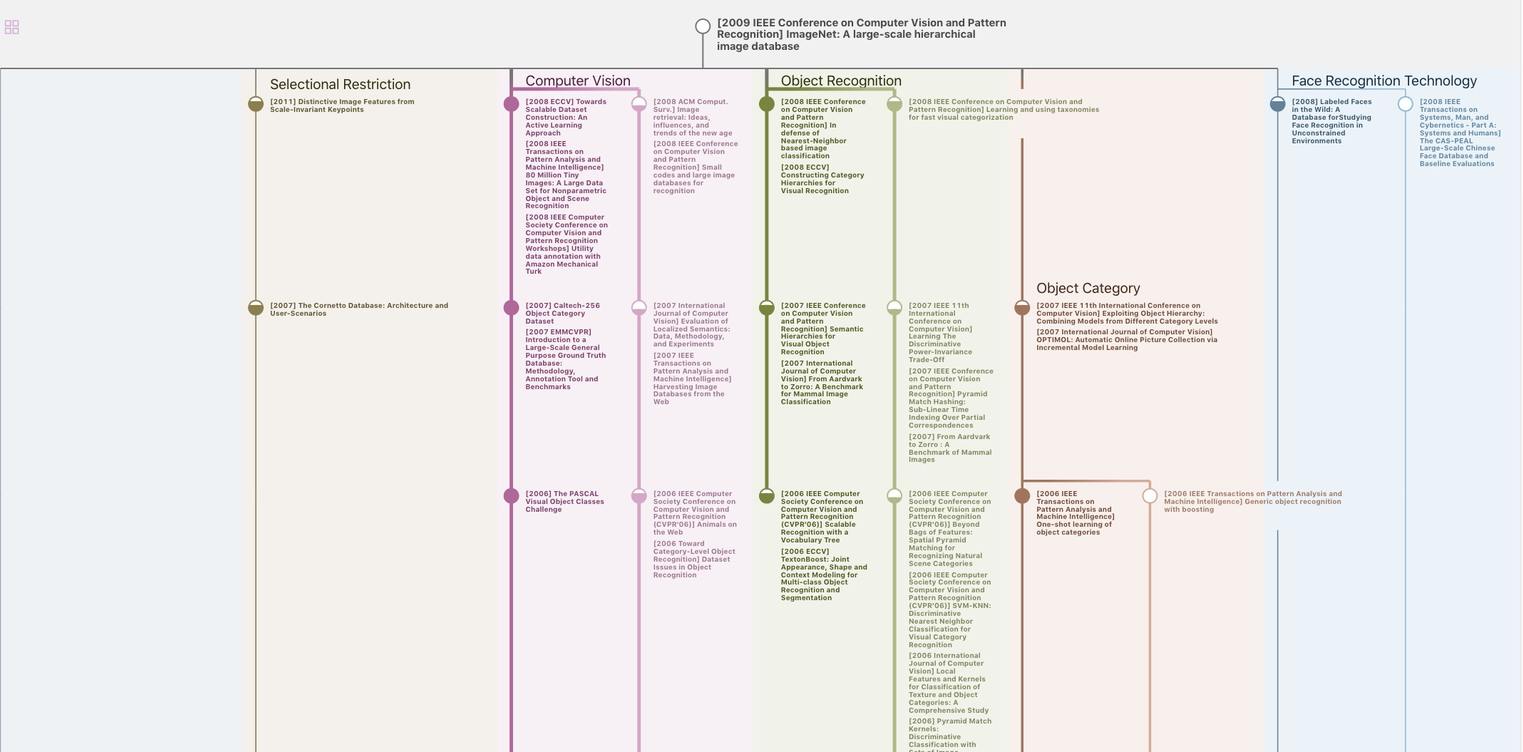
生成溯源树,研究论文发展脉络
Chat Paper
正在生成论文摘要