Digger: Detecting Copyright Content Mis-usage in Large Language Model Training
CoRR(2024)
摘要
Pre-training, which utilizes extensive and varied datasets, is a critical
factor in the success of Large Language Models (LLMs) across numerous
applications. However, the detailed makeup of these datasets is often not
disclosed, leading to concerns about data security and potential misuse. This
is particularly relevant when copyrighted material, still under legal
protection, is used inappropriately, either intentionally or unintentionally,
infringing on the rights of the authors.
In this paper, we introduce a detailed framework designed to detect and
assess the presence of content from potentially copyrighted books within the
training datasets of LLMs. This framework also provides a confidence estimation
for the likelihood of each content sample's inclusion. To validate our
approach, we conduct a series of simulated experiments, the results of which
affirm the framework's effectiveness in identifying and addressing instances of
content misuse in LLM training processes. Furthermore, we investigate the
presence of recognizable quotes from famous literary works within these
datasets. The outcomes of our study have significant implications for ensuring
the ethical use of copyrighted materials in the development of LLMs,
highlighting the need for more transparent and responsible data management
practices in this field.
更多查看译文
AI 理解论文
溯源树
样例
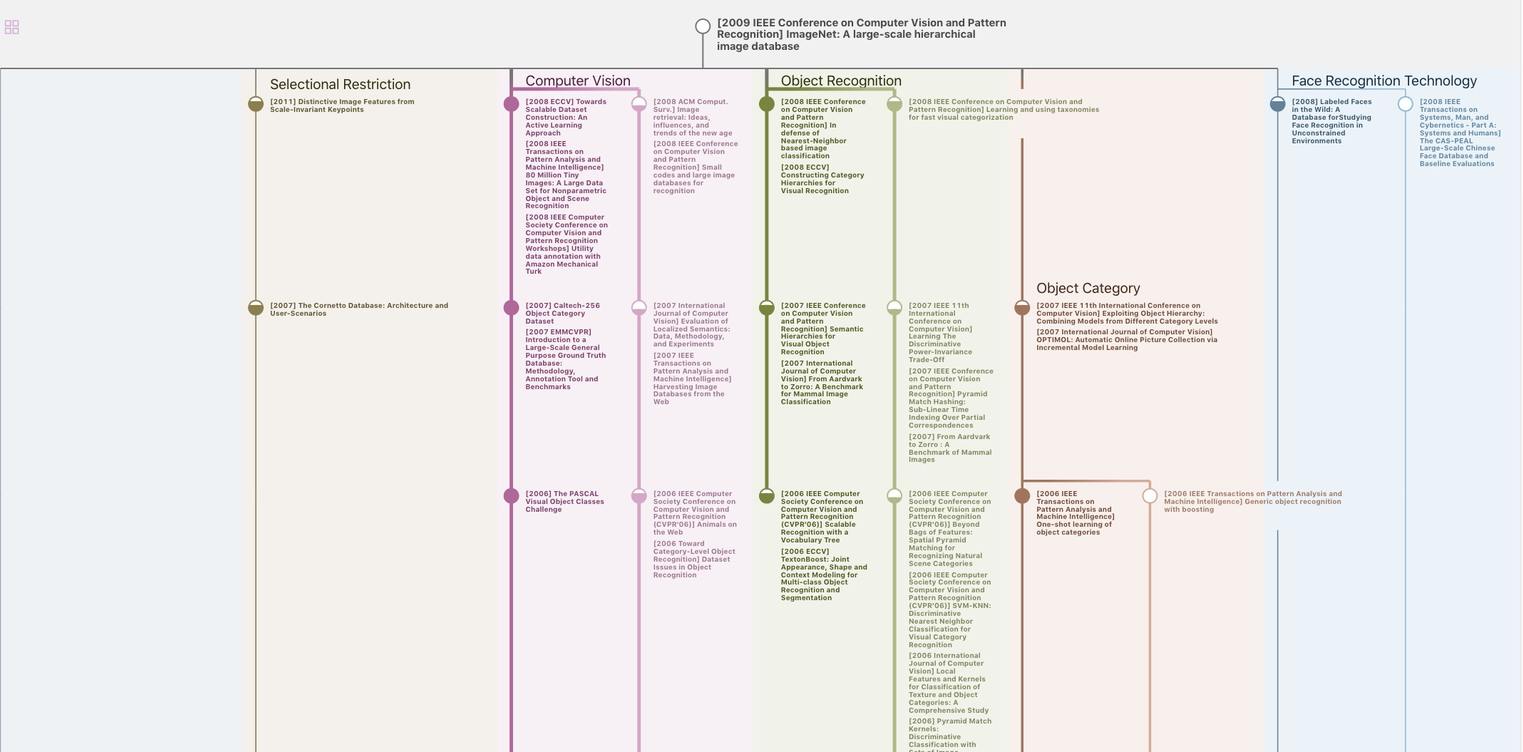
生成溯源树,研究论文发展脉络
Chat Paper
正在生成论文摘要