Chain-of-Table: Evolving Tables in the Reasoning Chain for Table Understanding
ICLR(2024)
摘要
Table-based reasoning with large language models (LLMs) is a promisingdirection to tackle many table understanding tasks, such as table-basedquestion answering and fact verification. Compared with generic reasoning,table-based reasoning requires the extraction of underlying semantics from bothfree-form questions and semi-structured tabular data. Chain-of-Thought and itssimilar approaches incorporate the reasoning chain in the form of textualcontext, but it is still an open question how to effectively leverage tabulardata in the reasoning chain. We propose the Chain-of-Table framework, wheretabular data is explicitly used in the reasoning chain as a proxy forintermediate thoughts. Specifically, we guide LLMs using in-context learning toiteratively generate operations and update the table to represent a tabularreasoning chain. LLMs can therefore dynamically plan the next operation basedon the results of the previous ones. This continuous evolution of the tableforms a chain, showing the reasoning process for a given tabular problem. Thechain carries structured information of the intermediate results, enabling moreaccurate and reliable predictions. Chain-of-Table achieves new state-of-the-artperformance on WikiTQ, FeTaQA, and TabFact benchmarks across multiple LLMchoices.
更多查看译文
关键词
Table Understanding,In-context Learning,Large Language Model
AI 理解论文
溯源树
样例
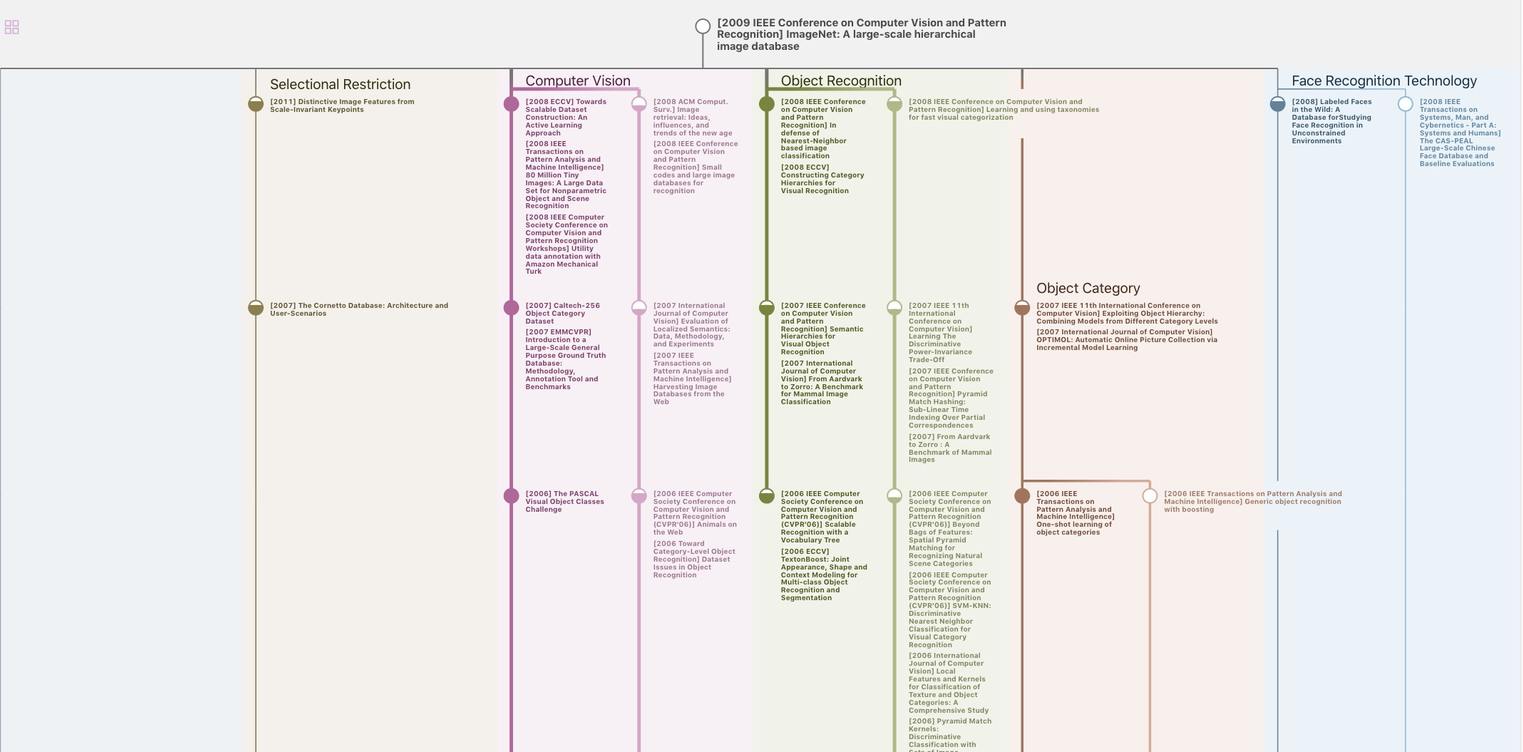
生成溯源树,研究论文发展脉络
Chat Paper
正在生成论文摘要