Improving Multilingual and Code-Switching ASR Using Large Language Model Generated Text.
Automatic Speech Recognition & Understanding(2023)
摘要
We investigate using large language models (LLMs) to generate text-only training data for improving multilingual and code-switching automatic speech recognition (ASR) through a text injection method. In a multilingual setup or a low-resource scenario such as code-switching, we propose to generate text data using the state-of-the-art PaLM 2. To better match the generated text data with specific tasks, we use prompt tuning to adapt PaLM 2 to generate domain-relevant multilingual or code-switched text data for text injection. We can achieve significant improvements in Word Error Rate (WER) in both multilingual and code-switching scenarios. The multilingual experiment shows a $6.2 \%$ relative WER reduction on average, i.e., from $11.25 \%$ to $10.55 \%$, compared to a baseline without text injection. The improvement is up to $23.1 \%$ improvement for certain languages. While in the code-switching scenario, we use English-only prompts to generate Mandarin-English code-switching text and achieve a 3.6% relative WER reduction for a code-switching test set, as well as WER reductions in both English and Mandarin monolingual scenarios, $5.3 \%$ and $8.5 \%$ relative, respectively. Our findings demonstrate that leveraging LLMs for text generation and then injection benefits multilingual or code-switching ASR tasks.
更多查看译文
关键词
text injection,large language model,prompt tuning,multilingual,code-switching
AI 理解论文
溯源树
样例
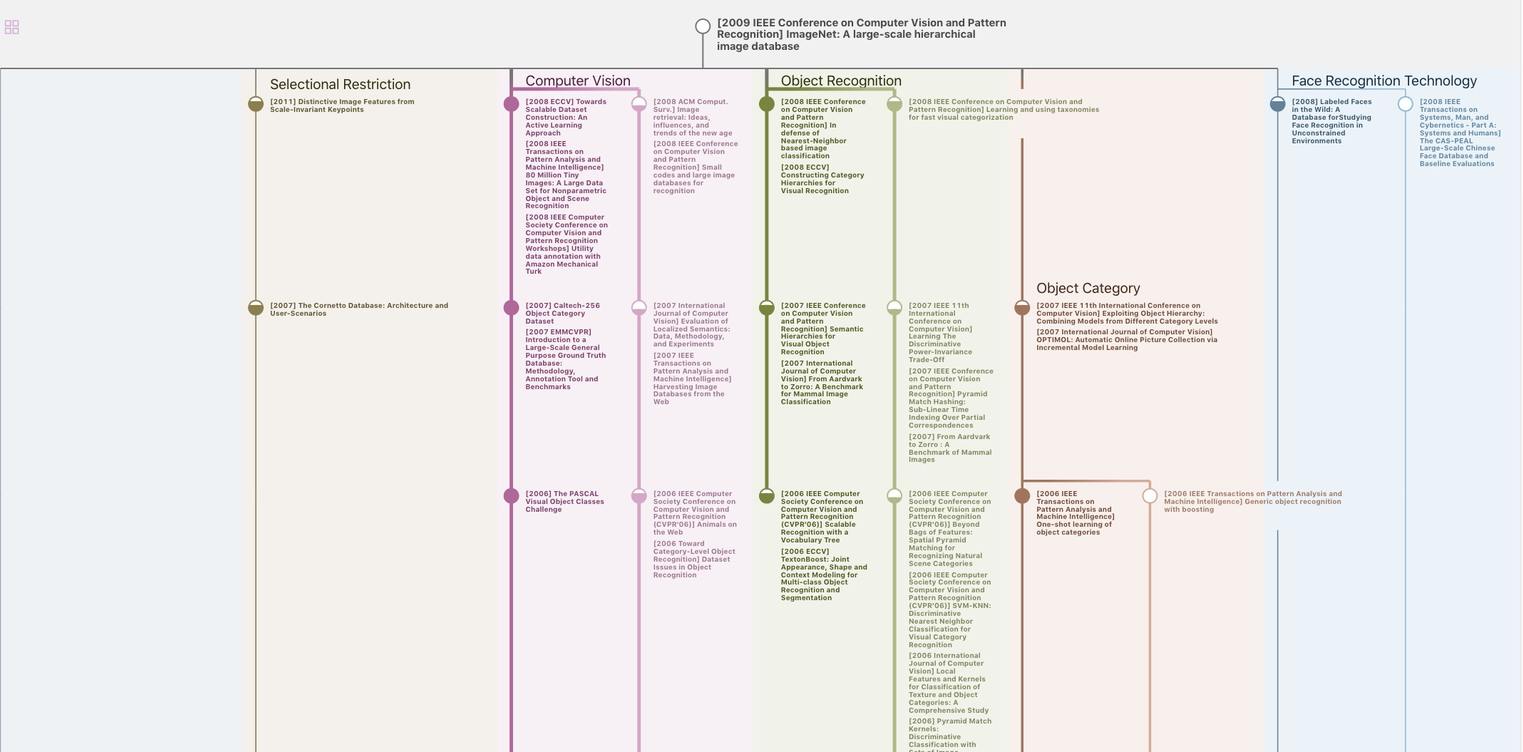
生成溯源树,研究论文发展脉络
Chat Paper
正在生成论文摘要