Physics-informed Active Learning for Accelerating Quantum Chemical Simulations.
arXiv (Cornell University)(2024)
摘要
Quantum chemical simulations can be greatly accelerated by constructing machine learning potentials, which is often done using active learning (AL). The usefulness of the constructed potentials is often limited by the high effort required and their insufficient robustness in the simulations. Here, we introduce the end-to-end AL for constructing robust data-efficient potentials with affordable investment of time and resources and minimum human interference. Our AL protocol is based on the physics-informed sampling of training points, automatic selection of initial data, uncertainty quantification, and convergence monitoring. The versatility of this protocol is shown in our implementation of quasi-classical molecular dynamics for simulating vibrational spectra, conformer search of a key biochemical molecule, and time-resolved mechanism of the Diels-Alder reaction. These investigations took us days instead of weeks of pure quantum chemical calculations on a high-performance computing cluster.
更多查看译文
关键词
Virtual Laboratories,Quantum,Chemistry Education,Distance Learning,Green Chemistry
AI 理解论文
溯源树
样例
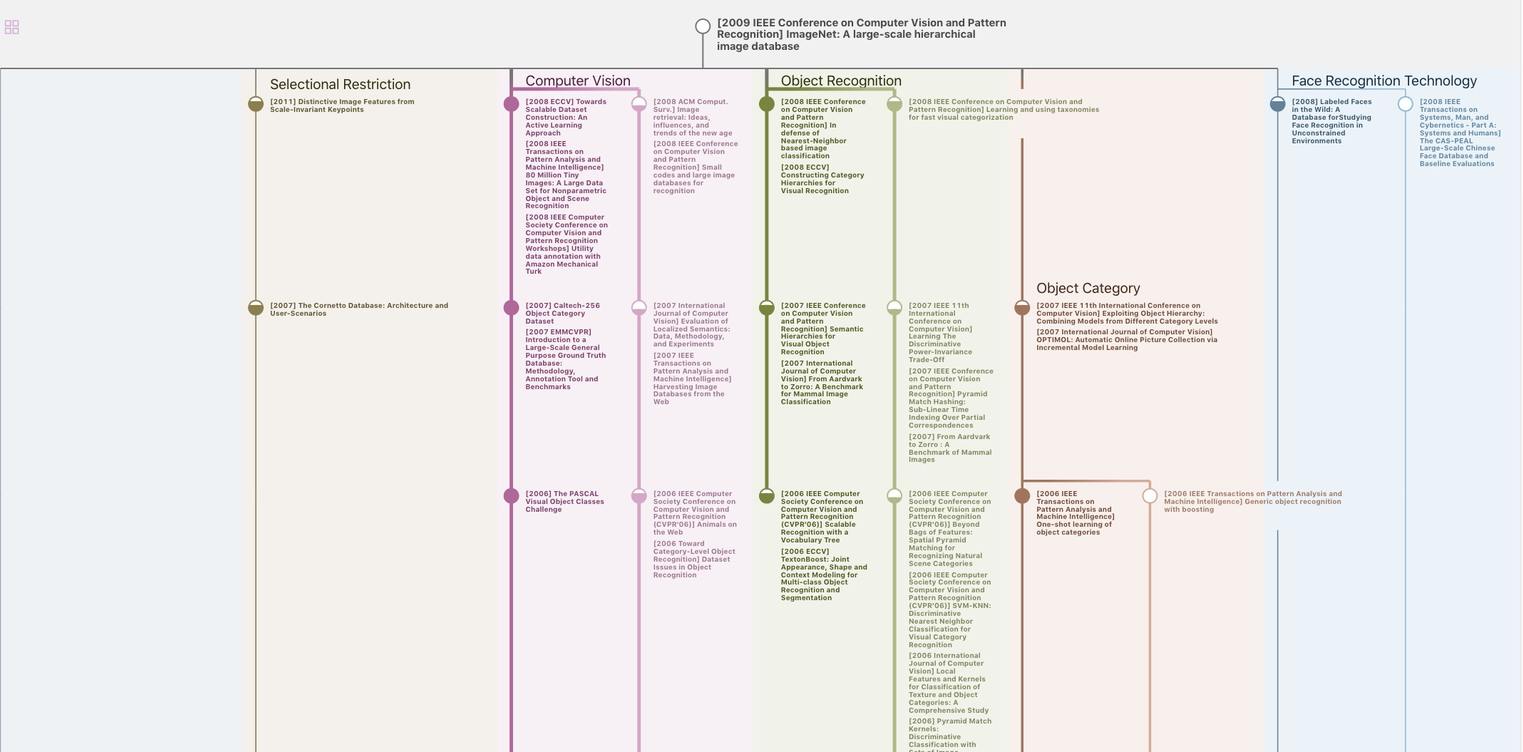
生成溯源树,研究论文发展脉络
Chat Paper
正在生成论文摘要