REIN: Reusing ImageNet to Improve Open-set Object Detection
International Conference on Digital Image Computing Techniques and Applications(2023)
摘要
There has been a notable advancement in the task of object detection over the last few years. However, recent studies reveal that modern object detectors suffer a considerable performance drop in an open-set setting and tend to incorrectly detect unknown objects and mislabel them as belonging to known categories with a high degree of confidence. We observe that the ‘catch-all background’ approach with limited training data from the detection dataset is insufficient to equip a detector with the ability to reject all unknown objects as background. Based on this observation, we propose to augment the detection dataset by reusing a subset of ImageNet that does not contain any object categories predefined by the detection task. The augmented training on a held-in background dataset allows the detector to reject unknown object instances as background instead of mislabelling them. Our experiments demonstrate that the proposed simple yet effective REusing ImageNet (REIN) technique improves the performance of object detectors in an open-set setting. Given the promising results, we hope that our method can encourage our community to pay more attention to open-set object detection and to rethink the data augmentation in detection.
更多查看译文
关键词
Object Detection,Open-set Detection,ImageNet
AI 理解论文
溯源树
样例
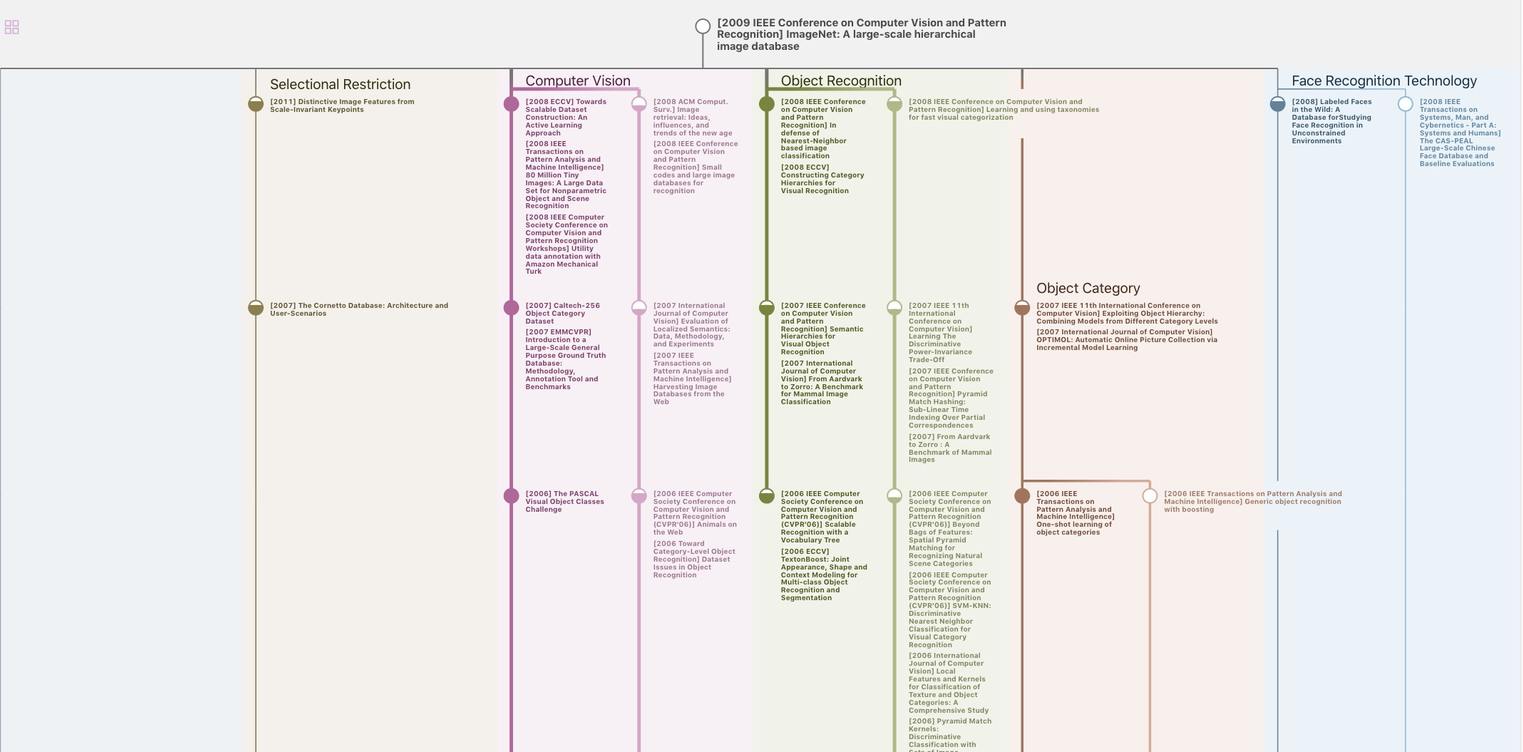
生成溯源树,研究论文发展脉络
Chat Paper
正在生成论文摘要