MRAnnotator: A Multi-Anatomy Deep Learning Model for MRI Segmentation
arXiv (Cornell University)(2024)
摘要
Purpose To develop a deep learning model for multi-anatomy and many-classsegmentation of diverse anatomic structures on MRI imaging. Materials and Methods In this retrospective study, two datasets were curatedand annotated for model development and evaluation. An internal dataset of 1022MRI sequences from various clinical sites within a health system and anexternal dataset of 264 MRI sequences from an independent imaging center werecollected. In both datasets, 49 anatomic structures were annotated as theground truth. The internal dataset was divided into training, validation, andtest sets and used to train and evaluate an nnU-Net model. The external datasetwas used to evaluate nnU-Net model generalizability and performance in allclasses on independent imaging data. Dice scores were calculated to evaluatemodel segmentation performance. Results The model achieved an average Dice score of 0.801 on the internaltest set, and an average score of 0.814 on the complete external dataset across49 classes. Conclusion The developed model achieves robust and generalizable segmentationof 49 anatomic structures on MRI imaging. A future direction is focused on theincorporation of additional anatomic regions and structures into the datasetsand model.
更多查看译文
关键词
MRI Segmentation,Medical Image Analysis,MRI
AI 理解论文
溯源树
样例
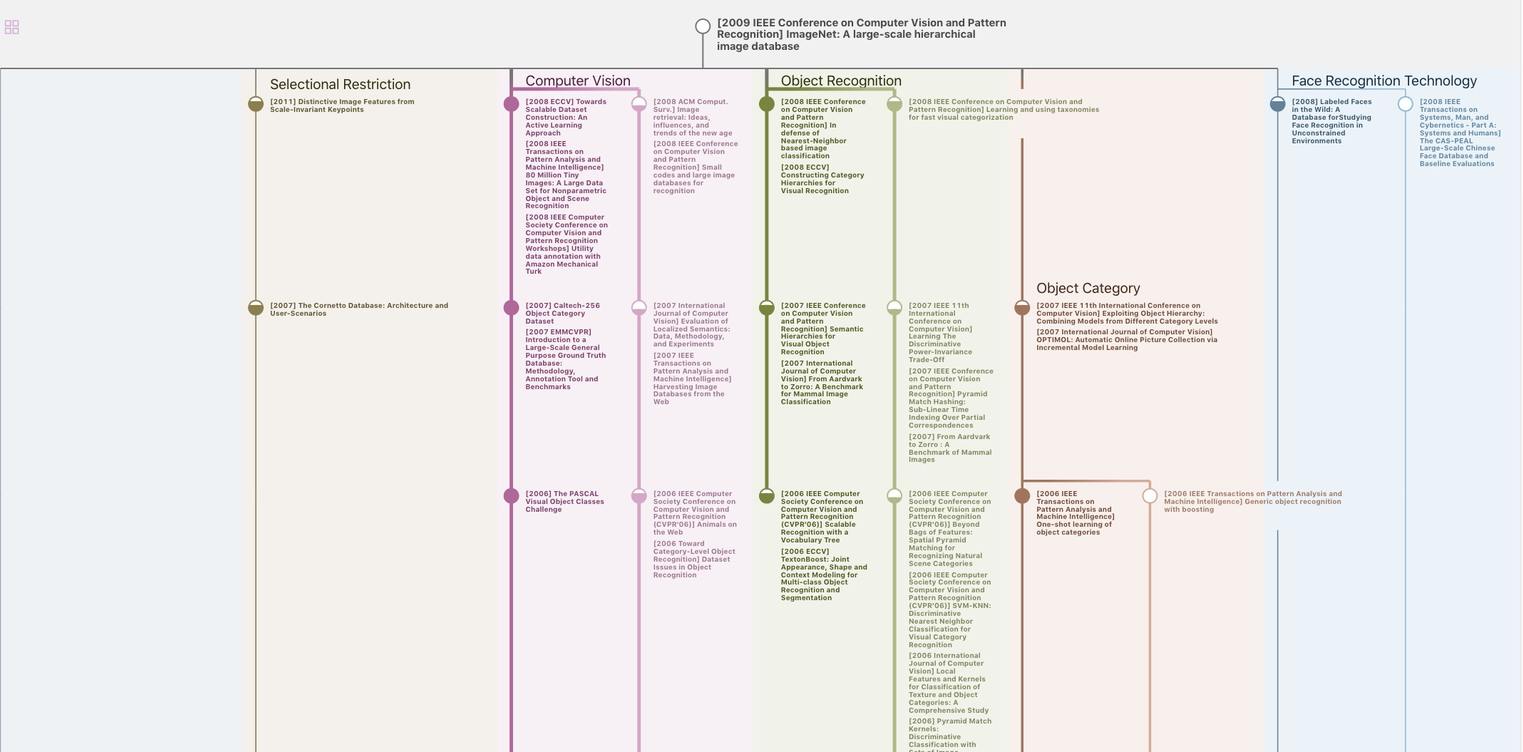
生成溯源树,研究论文发展脉络
Chat Paper
正在生成论文摘要