Device-Unimodal Cloud-Multimodal Collaboration for Livestreaming Content Understanding
2023 IEEE International Conference on Data Mining (ICDM)(2023)
摘要
Mobile livestreaming has revolutionized the online shopping paradigm, enabling streamers to promote products to consumers with an immersive and interactive experience. To guide consumers to the livestreams that involve their interested products, it is necessary to have a good understanding of livestreaming contents with low latency, and the key task is to accurately recognize the products being promoted by the streamers. However, the mainstream cloud-based service framework is challenged by the high concurrency of service requests, the high overhead of multimodal recognition, and the requirement of low response latency. To break the bottleneck, we propose a new device-cloud collaborative learning framework, where each streamer’s mobile device holds a unimodal recognition model that can process most of frames and also uploads the extracted unimodal features to facilitate the cloud-side multimodal recognition of the remaining few frames. In addition, the on-device unimodal model is incrementally trained over the samples constructed by leveraging the streamers’ manual labeling behaviors, thereby adapting to the heterogeneous and dynamic livestreaming contents of different streamers. Nevertheless, the device-side personalized unimodal features are misaligned in feature space and cannot be directly fused into the cloud-side multimodal model. We thus design a pluggable prompt generation module to transform the personalized unimodal features into prompt embeddings, instructing the multimodal backbone network in feature fusion. Both offline and online evaluation results reveal the effectiveness and efficiency of our design as well as its consistent advantage over existing baselines.
更多查看译文
关键词
n/a
AI 理解论文
溯源树
样例
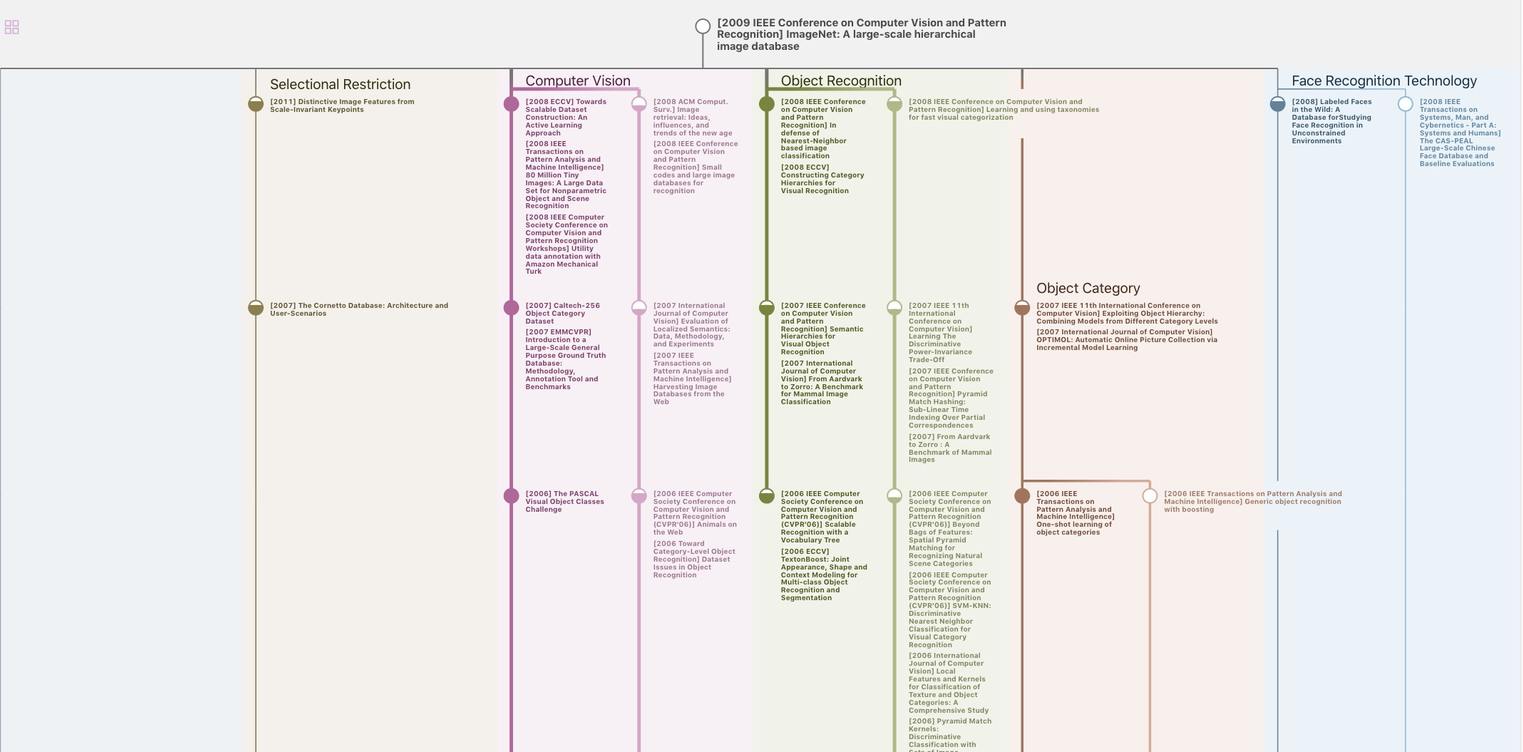
生成溯源树,研究论文发展脉络
Chat Paper
正在生成论文摘要