AED: Adaptable Error Detection for Few-shot Imitation Policy
CoRR(2024)
摘要
We study how to report few-shot imitation (FSI) policies' behavior errors in
novel environments, a novel task named adaptable error detection (AED). The
potential to cause serious damage to surrounding areas limits the application
of FSI policies in real-world scenarios. Thus, a robust system is necessary to
notify operators when FSI policies are inconsistent with the intent of
demonstrations. We develop a cross-domain benchmark for the challenging AED
task, consisting of 329 base and 158 novel environments. This task introduces
three challenges, including (1) detecting behavior errors in novel
environments, (2) behavior errors occurring without revealing notable changes,
and (3) lacking complete temporal information of the rollout due to the
necessity of online detection. To address these challenges, we propose Pattern
Observer (PrObe) to parse discernible patterns in the policy feature
representations of normal or error states, whose effectiveness is verified in
the proposed benchmark. Through our comprehensive evaluation, PrObe
consistently surpasses strong baselines and demonstrates a robust capability to
identify errors arising from a wide range of FSI policies. Moreover, we conduct
comprehensive ablations and experiments (error correction, demonstration
quality, etc.) to validate the practicality of our proposed task and
methodology.
更多查看译文
AI 理解论文
溯源树
样例
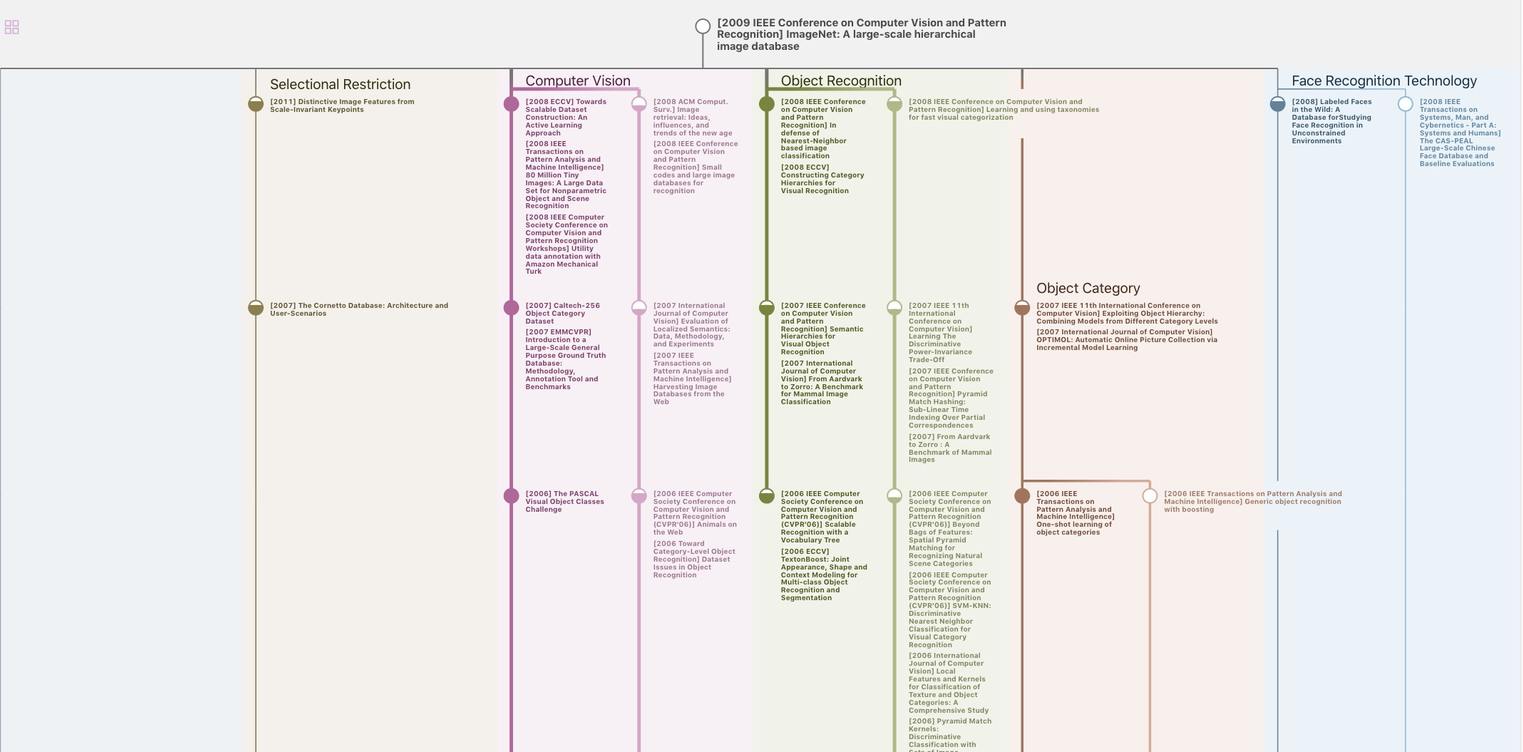
生成溯源树,研究论文发展脉络
Chat Paper
正在生成论文摘要