MEMORYLLM: Towards Self-Updatable Large Language Models
ICML 2024(2024)
摘要
Existing Large Language Models (LLMs) usually remain static after deployment,which might make it hard to inject new knowledge into the model. We aim tobuild models containing a considerable portion of self-updatable parameters,enabling the model to integrate new knowledge effectively and efficiently. Tothis end, we introduce MEMORYLLM, a model that comprises a transformer and afixed-size memory pool within the latent space of the transformer. MEMORYLLMcan self-update with text knowledge and memorize the knowledge injectedearlier. Our evaluations demonstrate the ability of MEMORYLLM to effectivelyincorporate new knowledge, as evidenced by its performance on model editingbenchmarks. Meanwhile, the model exhibits long-term information retentioncapacity, which is validated through our custom-designed evaluations andlong-context benchmarks. MEMORYLLM also shows operational integrity without anysign of performance degradation even after nearly a million memory updates. Ourcode and model are open-sourced at https://github.com/wangyu-ustc/MemoryLLM.
更多查看译文
AI 理解论文
溯源树
样例
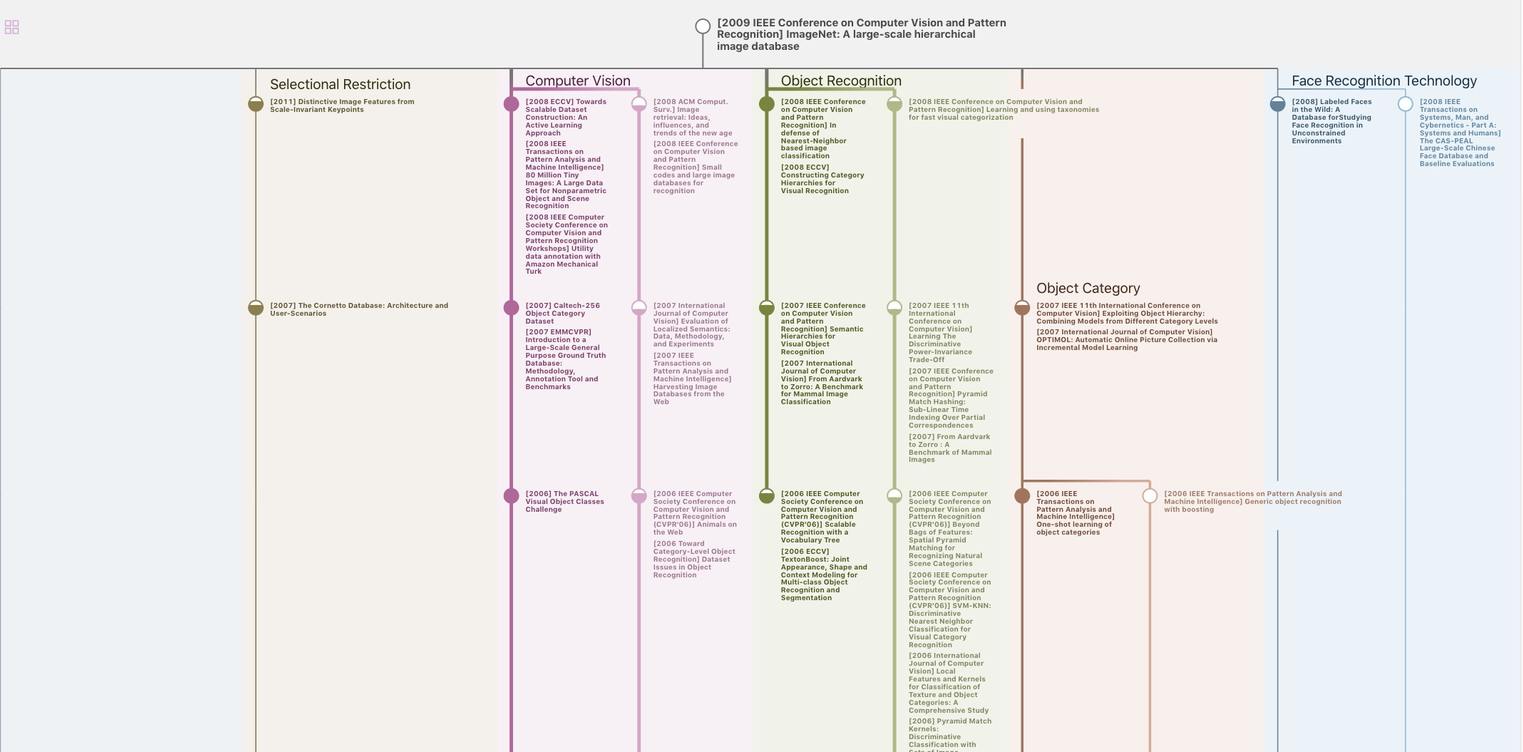
生成溯源树,研究论文发展脉络
Chat Paper
正在生成论文摘要