Progressive Gradient Flow for Robust N:M Sparsity Training in Transformers
CoRR(2024)
摘要
N:M Structured sparsity has garnered significant interest as a result of
relatively modest overhead and improved efficiency. Additionally, this form of
sparsity holds considerable appeal for reducing the memory footprint owing to
their modest representation overhead. There have been efforts to develop
training recipes for N:M structured sparsity, they primarily focus on
low-sparsity regions (∼50%). Nonetheless, performance of models trained
using these approaches tends to decline when confronted with high-sparsity
regions (>80%). In this work, we study the effectiveness of existing sparse
training recipes at high-sparsity regions and argue that these methods
fail to sustain the model quality on par with low-sparsity regions. We
demonstrate that the significant factor contributing to this disparity is the
presence of elevated levels of induced noise in the gradient magnitudes. To
mitigate this undesirable effect, we employ decay mechanisms to progressively
restrict the flow of gradients towards pruned elements. Our approach improves
the model quality by up to 2% and 5% in vision and language models at
high sparsity regime, respectively. We also evaluate the trade-off between
model accuracy and training compute cost in terms of FLOPs. At iso-training
FLOPs, our method yields better performance compared to conventional sparse
training recipes, exhibiting an accuracy improvement of up to 2%. The source
code is available at
https://github.com/abhibambhaniya/progressive_gradient_flow_nm_sparsity.
更多查看译文
AI 理解论文
溯源树
样例
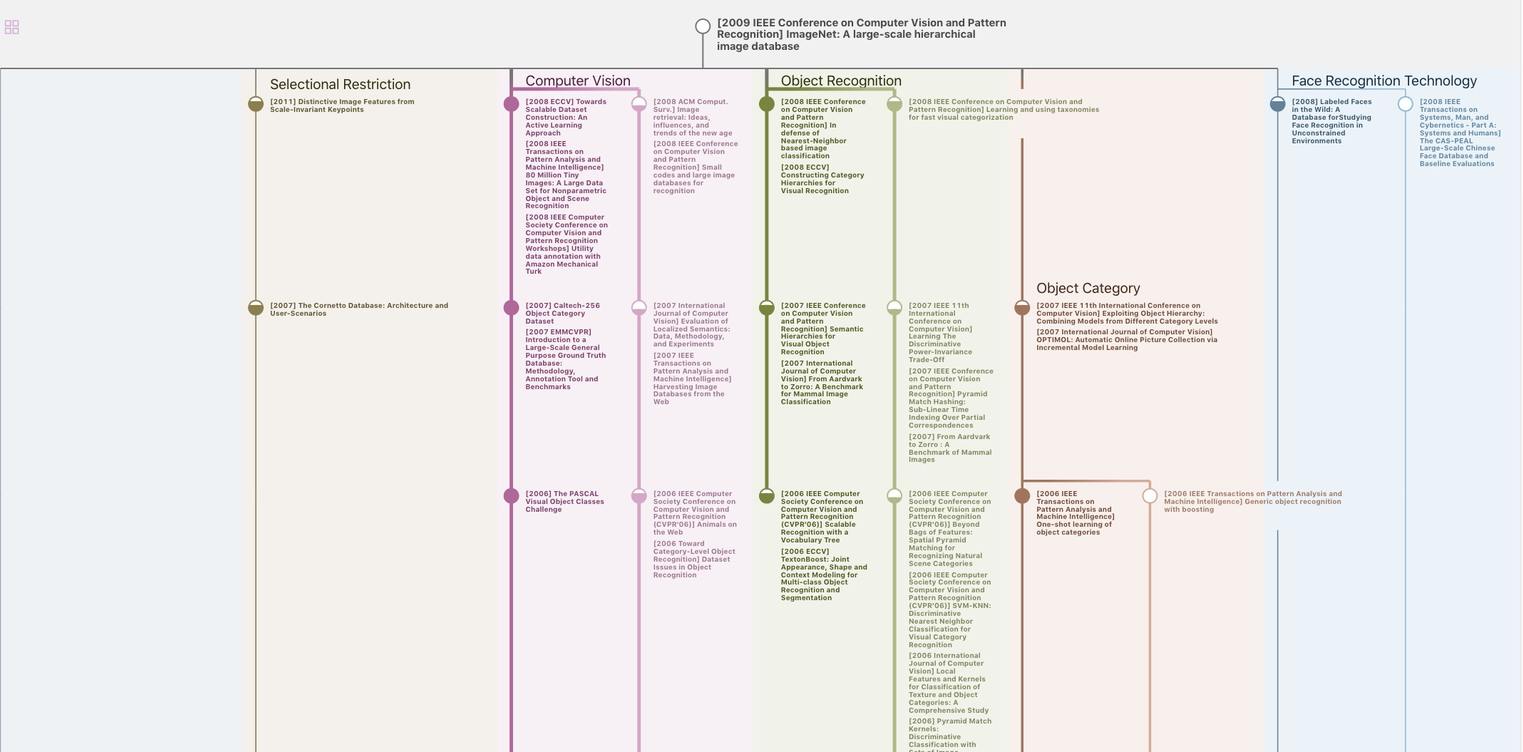
生成溯源树,研究论文发展脉络
Chat Paper
正在生成论文摘要