MCDet: Multi-Content Collaboration Detector for Multiscale Remote Sensing Object
IEEE GEOSCIENCE AND REMOTE SENSING LETTERS(2024)
摘要
In previous works, powerful convolutional neural network (CNN) backbones are typically used for one- or two-stage detectors to facilitate multicategories object classification. Unfortunately, continuous convolution and pooling operations tend to weaken the detailed information. We propose an end-to-end multi-content collaboration detector (MCDet) to improve object recognition accuracy. First, we summarize the reasons for the disappearance of detailed features in the traditional feature extraction backbone networks and propose a shallow clue refinement (SCR) module, which helps us to retain more critical local detail information in the downsampling process. Second, to receive more suitable contextual information, we design a self-dilating spatial pooling (SSP) module; it adaptively learns a contextual reception field, thereby alleviating the mismatch between the theoretical receptive field of the network design and the practical requirements. Finally, extensive experiments on the NWPU VHR-10 and DIOR datasets have shown that the proposed MCDet significantly improves detection accuracy. Our code is available at https://github.com/Xidian-AIGroup190726/RS-objectdetection-MCDet .
更多查看译文
关键词
Feature extraction,Object detection,Detectors,Correlation,Kernel,Convolution,Radio frequency,Contextual information,object detection,reception field,remote sensing images,shallow feature
AI 理解论文
溯源树
样例
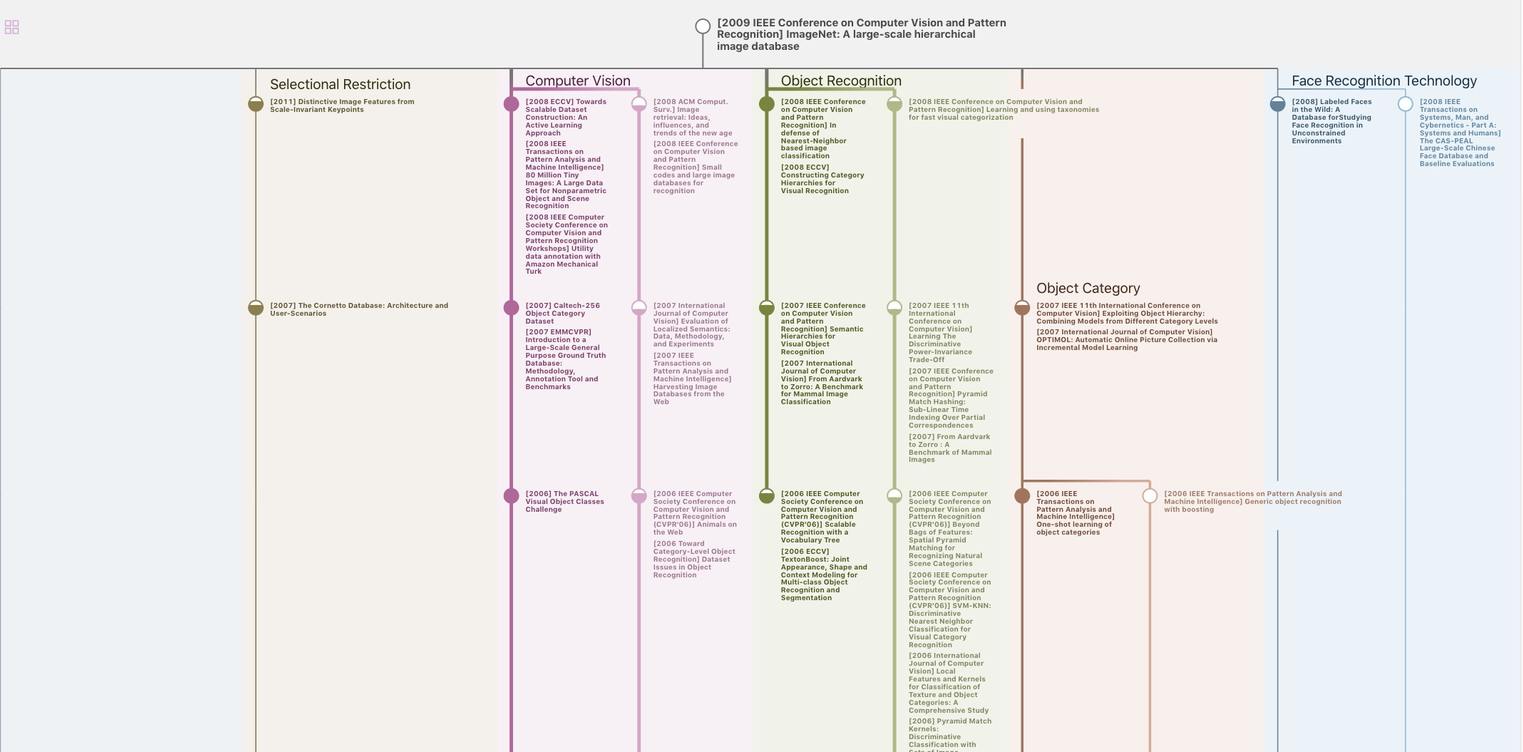
生成溯源树,研究论文发展脉络
Chat Paper
正在生成论文摘要