Only My Model On My Data: A Privacy Preserving Approach Protecting one Model and Deceiving Unauthorized Black-Box Models
CoRR(2024)
摘要
Deep neural networks are extensively applied to real-world tasks, such as
face recognition and medical image classification, where privacy and data
protection are critical. Image data, if not protected, can be exploited to
infer personal or contextual information. Existing privacy preservation
methods, like encryption, generate perturbed images that are unrecognizable to
even humans. Adversarial attack approaches prohibit automated inference even
for authorized stakeholders, limiting practical incentives for commercial and
widespread adaptation. This pioneering study tackles an unexplored practical
privacy preservation use case by generating human-perceivable images that
maintain accurate inference by an authorized model while evading other
unauthorized black-box models of similar or dissimilar objectives, and
addresses the previous research gaps. The datasets employed are ImageNet, for
image classification, Celeba-HQ dataset, for identity classification, and
AffectNet, for emotion classification. Our results show that the generated
images can successfully maintain the accuracy of a protected model and degrade
the average accuracy of the unauthorized black-box models to 11.97
55.51
更多查看译文
AI 理解论文
溯源树
样例
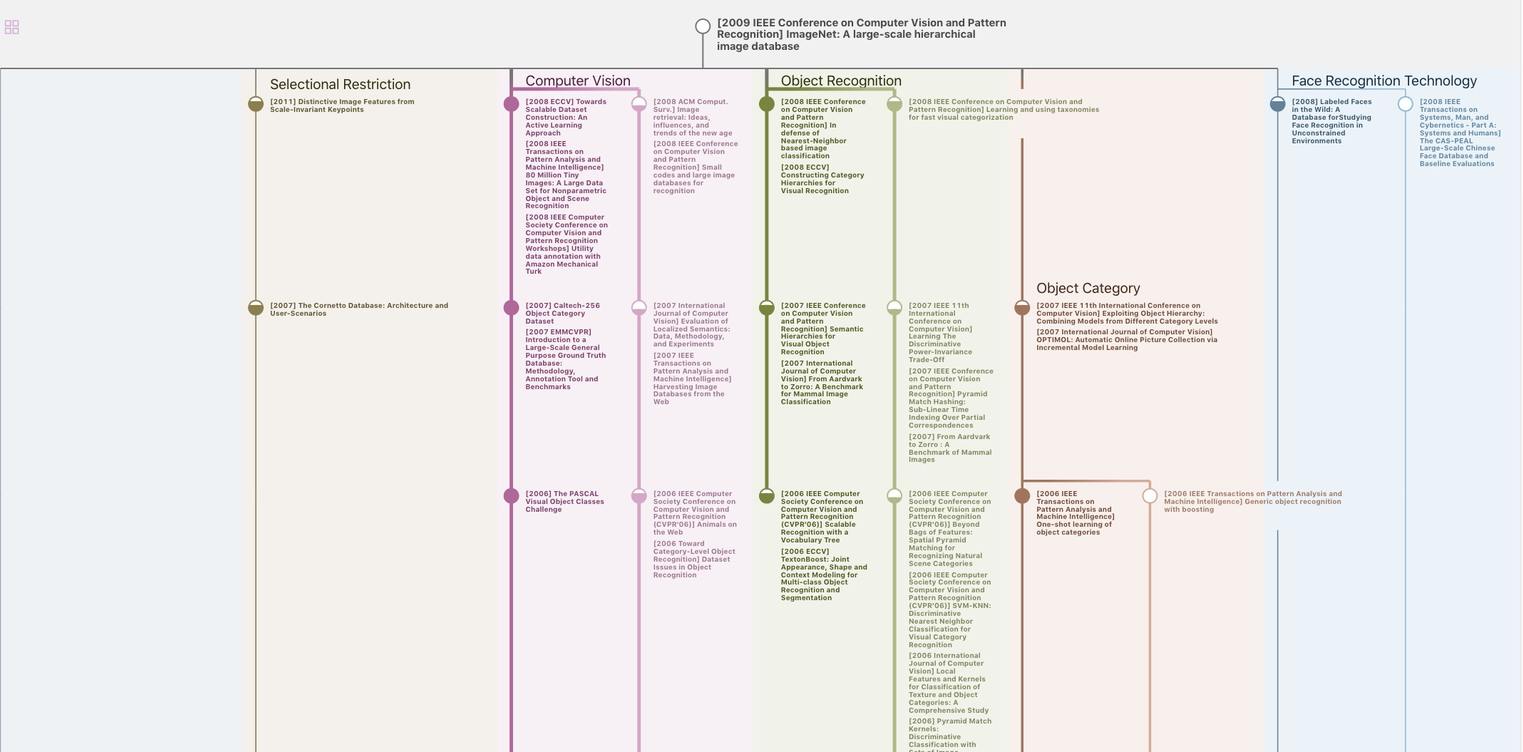
生成溯源树,研究论文发展脉络
Chat Paper
正在生成论文摘要