Distributionally Robust Ground Delay Programs with Learning-Driven Airport Capacity Predictions
arxiv(2024)
摘要
Strategic Traffic Management Initiatives (TMIs) such as Ground Delay Programs
(GDPs) play a crucial role in mitigating operational costs associated with
demand-capacity imbalances. However, GDPs can only be planned (e.g., duration,
delay assignments) with confidence if the future capacities at constrained
resources (i.e., airports) are predictable. In reality, such future capacities
are uncertain, and predictive models may provide forecasts that are vulnerable
to errors and distribution shifts. Motivated by the goal of planning optimal
GDPs that are distributionally robust against airport capacity
prediction errors, we study a fully integrated learning-driven optimization
framework. We design a deep learning-based prediction model capable of
forecasting arrival and departure capacity distributions across a network of
airports. We then integrate the forecasts into a distributionally robust
formulation of the multi-airport ground holding problem (dr-MAGHP). We
show how dr-MAGHP can outperform stochastic optimization when
distribution shifts occur, and conclude with future research directions to
improve both the learning and optimization stages.
更多查看译文
AI 理解论文
溯源树
样例
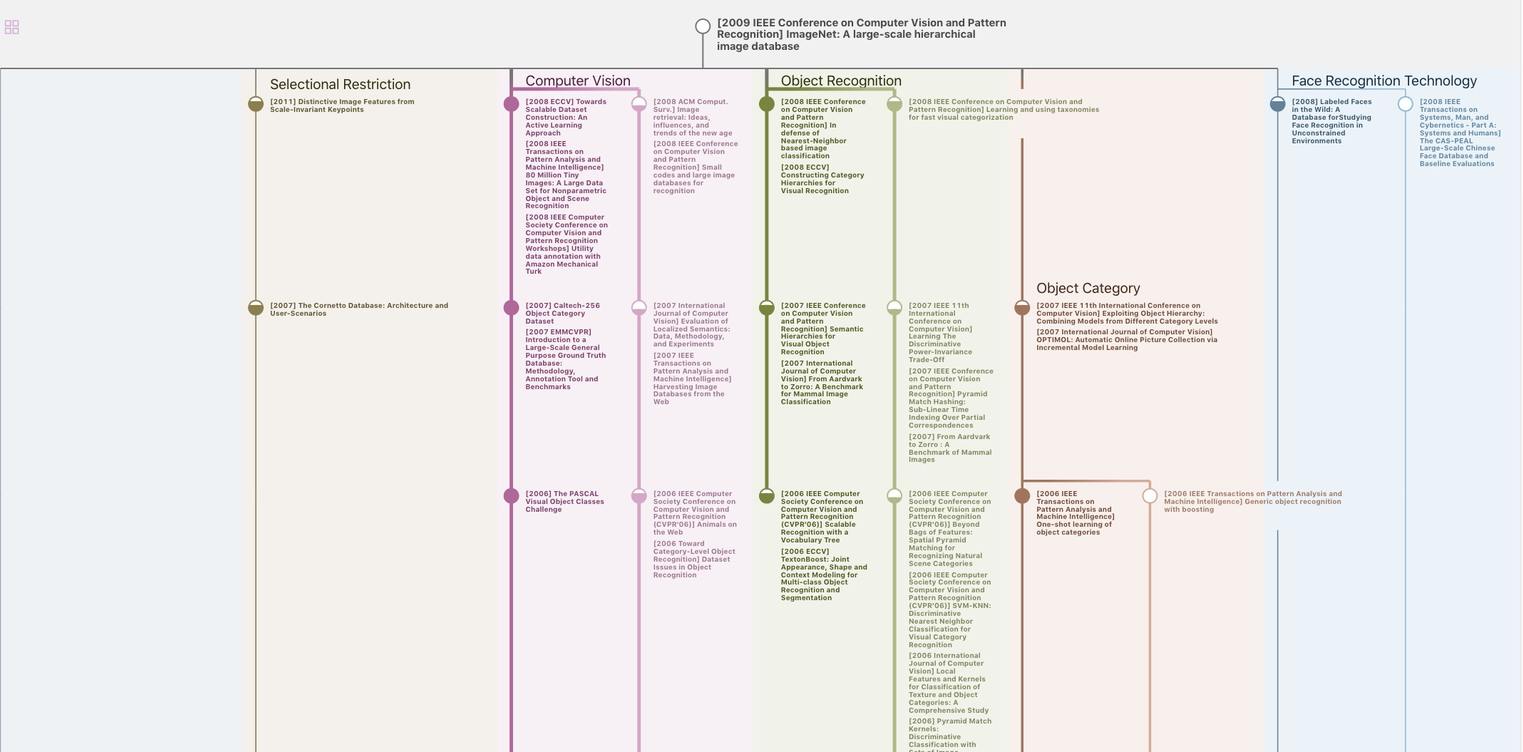
生成溯源树,研究论文发展脉络
Chat Paper
正在生成论文摘要