PhyGrasp: Generalizing Robotic Grasping with Physics-informed Large Multimodal Models
CoRR(2024)
摘要
Robotic grasping is a fundamental aspect of robot functionality, defining how
robots interact with objects. Despite substantial progress, its
generalizability to counter-intuitive or long-tailed scenarios, such as objects
with uncommon materials or shapes, remains a challenge. In contrast, humans can
easily apply their intuitive physics to grasp skillfully and change grasps
efficiently, even for objects they have never seen before.
This work delves into infusing such physical commonsense reasoning into
robotic manipulation. We introduce PhyGrasp, a multimodal large model that
leverages inputs from two modalities: natural language and 3D point clouds,
seamlessly integrated through a bridge module. The language modality exhibits
robust reasoning capabilities concerning the impacts of diverse physical
properties on grasping, while the 3D modality comprehends object shapes and
parts. With these two capabilities, PhyGrasp is able to accurately assess the
physical properties of object parts and determine optimal grasping poses.
Additionally, the model's language comprehension enables human instruction
interpretation, generating grasping poses that align with human preferences. To
train PhyGrasp, we construct a dataset PhyPartNet with 195K object instances
with varying physical properties and human preferences, alongside their
corresponding language descriptions. Extensive experiments conducted in the
simulation and on the real robots demonstrate that PhyGrasp achieves
state-of-the-art performance, particularly in long-tailed cases, e.g., about
10
https://sites.google.com/view/phygrasp
更多查看译文
AI 理解论文
溯源树
样例
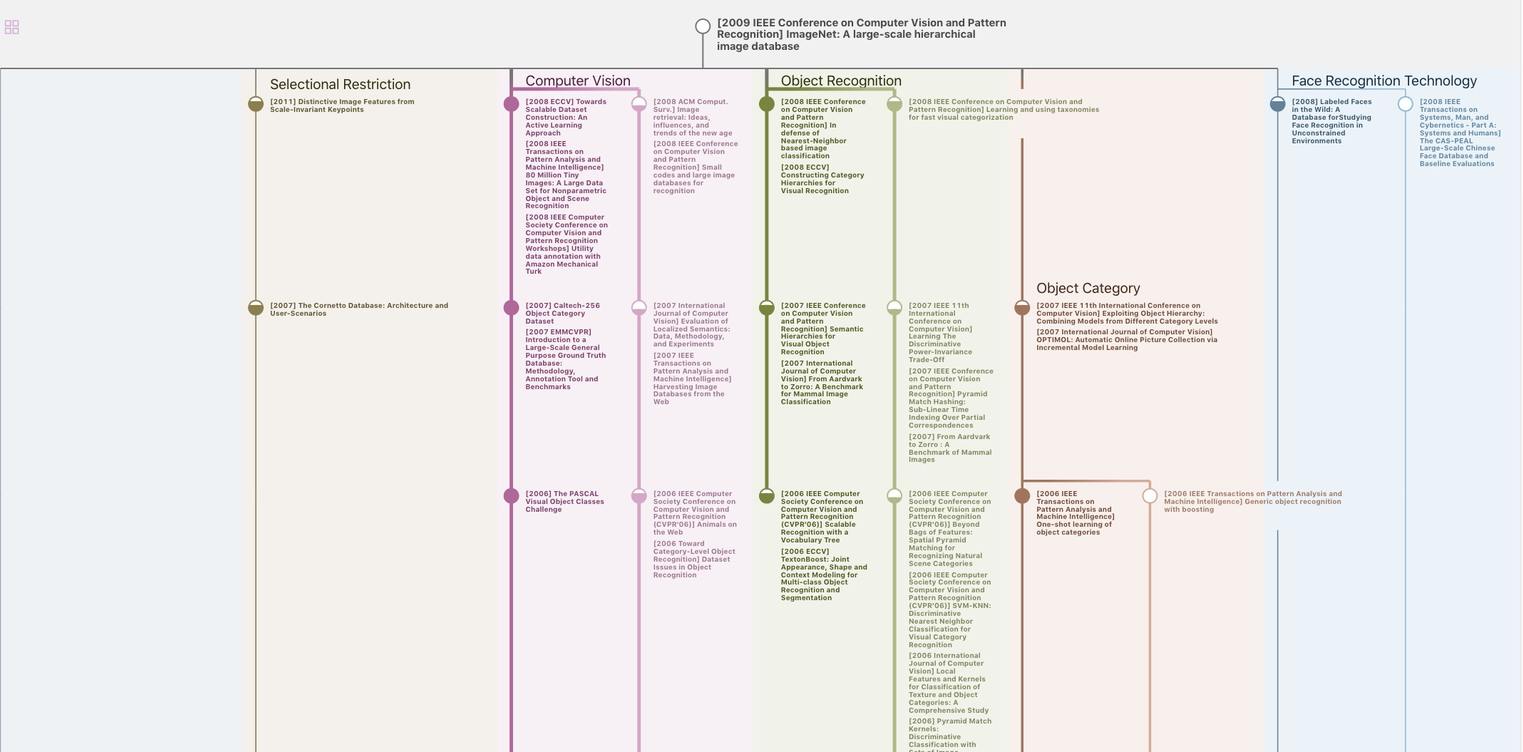
生成溯源树,研究论文发展脉络
Chat Paper
正在生成论文摘要