Self-Supervised Learning Reveals Clinically Relevant Histomorphological Patterns for Therapeutic Strategies in Colon Cancer
bioRxiv the preprint server for biology(2024)
摘要
Self-supervised learning (SSL) automates the extraction and interpretation of histopathology features on unannotated hematoxylin-and-eosin-stained whole-slide images (WSIs). We trained an SSL Barlow Twins-encoder on 435 TCGA colon adenocarcinoma WSIs to extract features from small image patches. Leiden community detection then grouped tiles into histomorphological phenotype clusters (HPCs). HPC reproducibility and predictive ability for overall survival was confirmed in an independent clinical trial cohort (N=1213 WSIs). This unbiased atlas resulted in 47 HPCs displaying unique and sharing clinically significant histomorphological traits, highlighting tissue type, quantity, and architecture, especially in the context of tumor stroma. Through in-depth analysis of these HPCs, including immune landscape and gene set enrichment analysis, and association to clinical outcomes, we shed light on the factors influencing survival and responses to treatments like standard adjuvant chemotherapy and experimental therapies. Further exploration of HPCs may unveil new insights and aid decision-making and personalized treatments for colon cancer patients.
更多查看译文
关键词
Histopathology Images,Whole Slide Imaging,Digital Pathology
AI 理解论文
溯源树
样例
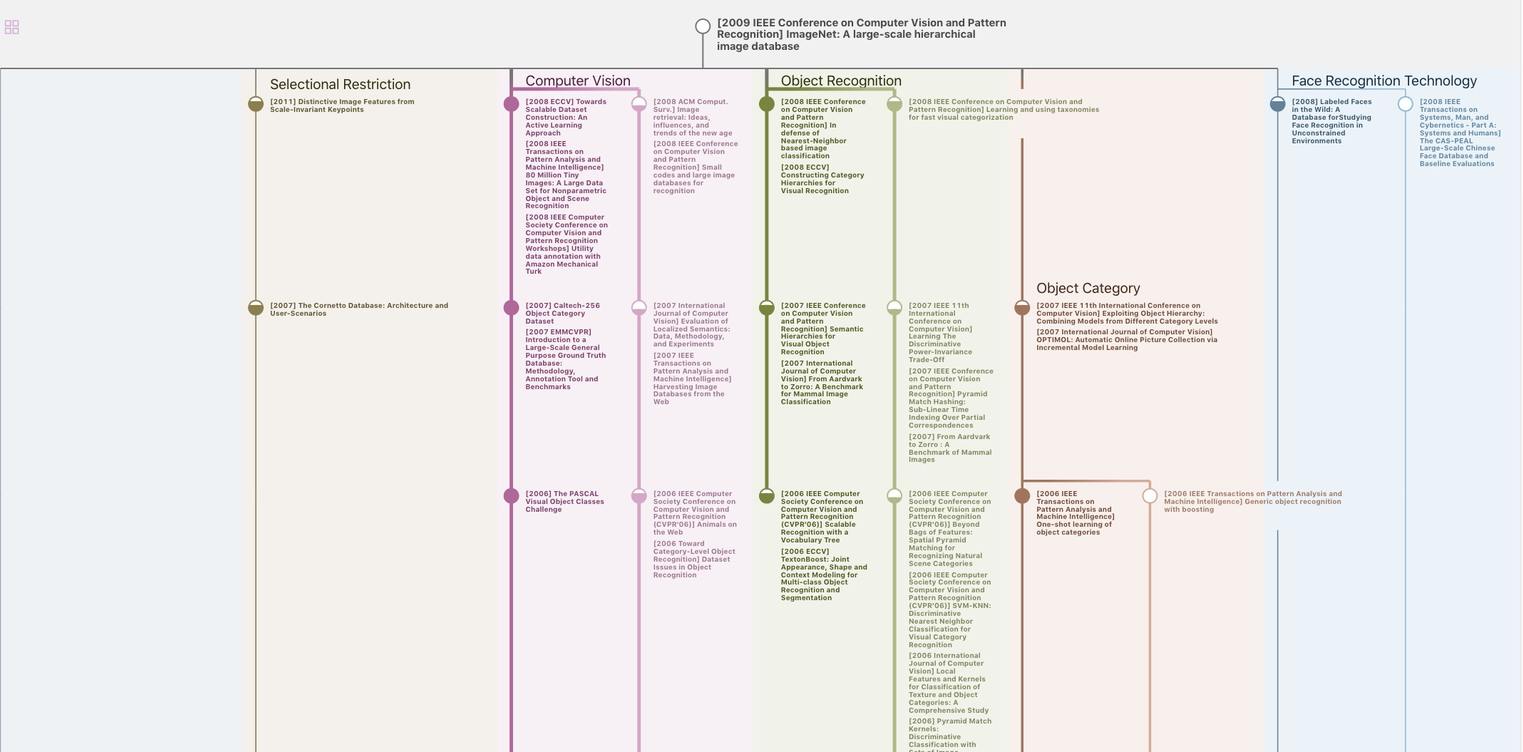
生成溯源树,研究论文发展脉络
Chat Paper
正在生成论文摘要