Counter-Samples: A Stateless Strategy to Neutralize Black Box Adversarial Attacks
arXiv (Cornell University)(2024)
摘要
Our paper presents a novel defence against black box attacks, where attackersuse the victim model as an oracle to craft their adversarial examples. Unliketraditional preprocessing defences that rely on sanitizing input samples, ourstateless strategy counters the attack process itself. For every query weevaluate a counter-sample instead, where the counter-sample is the originalsample optimized against the attacker's objective. By countering every blackbox query with a targeted white box optimization, our strategy effectivelyintroduces an asymmetry to the game to the defender's advantage. This defencenot only effectively misleads the attacker's search for an adversarial example,it also preserves the model's accuracy on legitimate inputs and is generic tomultiple types of attacks. We demonstrate that our approach is remarkably effective againststate-of-the-art black box attacks and outperforms existing defences for boththe CIFAR-10 and ImageNet datasets. Additionally, we also show that theproposed defence is robust against strong adversaries as well.
更多查看译文
关键词
Adversarial Examples,Defenses,Security Analysis
AI 理解论文
溯源树
样例
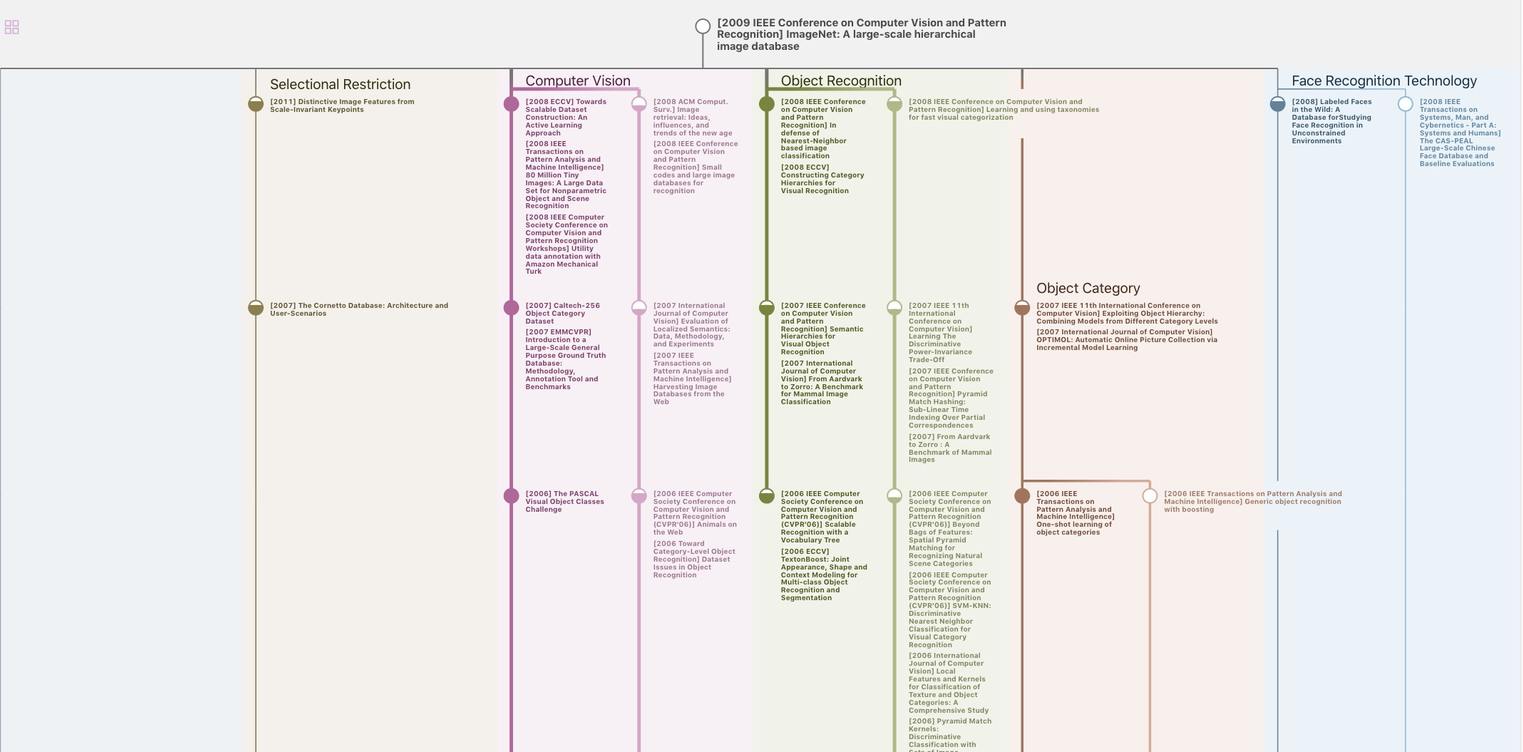
生成溯源树,研究论文发展脉络
Chat Paper
正在生成论文摘要