Distributional Fairness-aware Recommendation
ACM TRANSACTIONS ON INFORMATION SYSTEMS(2024)
摘要
Fairness has been gradually recognized as a significant problem in the recommendation domain. Previous models usually achieve fairness by reducing the average performance gap between different user groups. However, the average performance may not sufficiently represent all the characteristics of the performances in a user group. Thus, equivalent average performance may not mean the recommender model is fair, for example, the variance of the performances can be different. To alleviate this problem, in this article, we define a novel type of fairness, where we require that the performance distributions across different user groups should be similar. We prove that with the same performance distribution, the numerical characteristics of the group performance, including the expectation, variance, and any higher-order moment, are also the same. To achieve distributional fairness, we propose a generative and adversarial training framework. Specifically, we regard the recommender model as the generator to compute the performance for each user in different groups, and then we deploy a discriminator to judge which group the performance is drawn from. By iteratively optimizing the generator and the discriminator, we can theoretically prove that the optimal generator (the recommender model) can indeed lead to the equivalent performance distributions. To smooth the adversarial training process, we propose a novel dual curriculum learning strategy for optimal scheduling of training samples. Additionally, we tailor our framework to better suit top-N recommendation tasks by incorporating softened ranking metrics as measures of performance discrepancies. We conduct extensive experiments based on real-world datasets to demonstrate the effectiveness of our model.
更多查看译文
关键词
Distributional fairness,adversarial training,recommender system
AI 理解论文
溯源树
样例
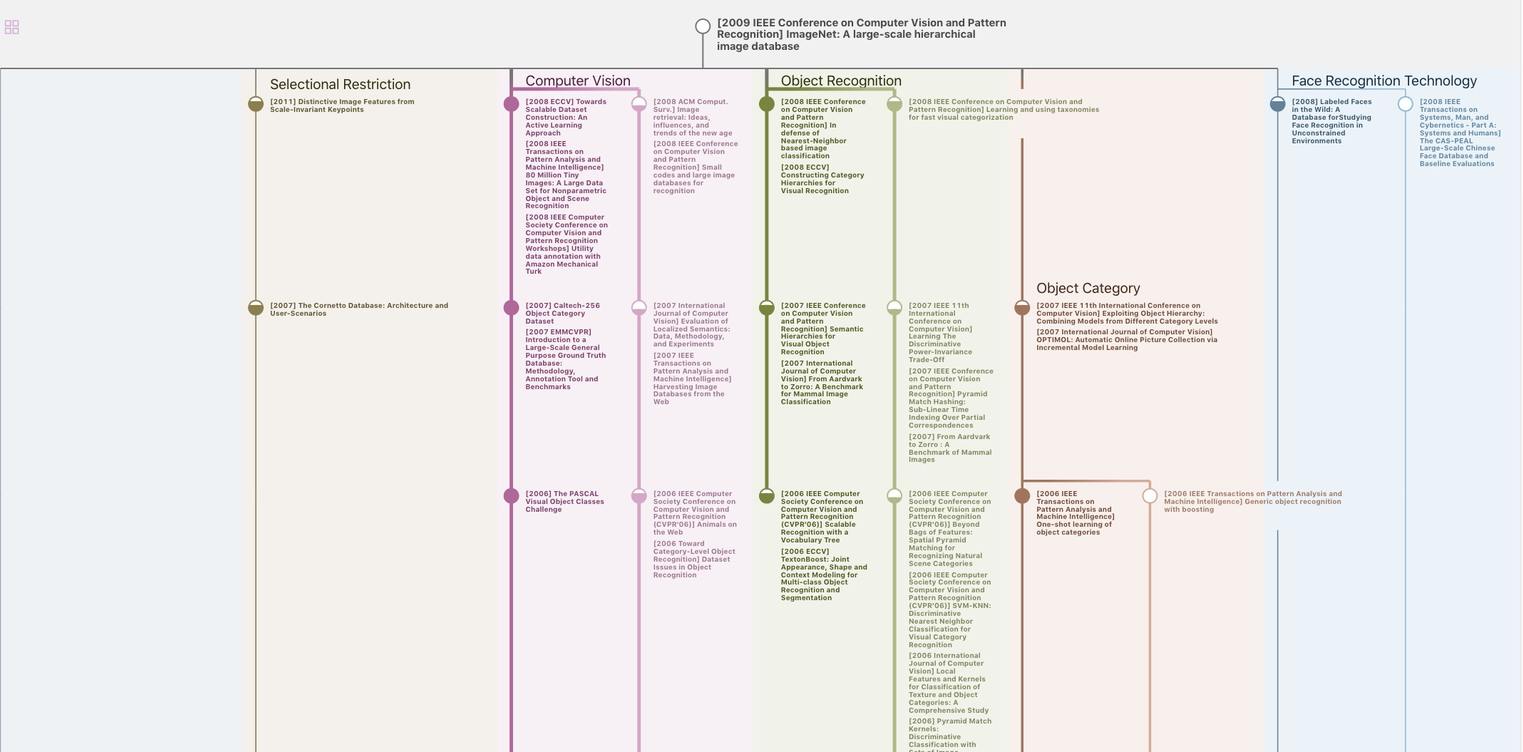
生成溯源树,研究论文发展脉络
Chat Paper
正在生成论文摘要