OpenEval: Benchmarking Chinese LLMs Across Capability, Alignment and Safety
Proceedings of the 62nd Annual Meeting of the Association for Computational Linguistics (Volume 3 System Demonstrations)(2024)
摘要
The rapid development of Chinese large language models (LLMs) poses bigchallenges for efficient LLM evaluation. While current initiatives haveintroduced new benchmarks or evaluation platforms for assessing Chinese LLMs,many of these focus primarily on capabilities, usually overlooking potentialalignment and safety issues. To address this gap, we introduce OpenEval, anevaluation testbed that benchmarks Chinese LLMs across capability, alignmentand safety. For capability assessment, we include 12 benchmark datasets toevaluate Chinese LLMs from 4 sub-dimensions: NLP tasks, disciplinary knowledge,commonsense reasoning and mathematical reasoning. For alignment assessment,OpenEval contains 7 datasets that examines the bias, offensiveness andillegalness in the outputs yielded by Chinese LLMs. To evaluate safety,especially anticipated risks (e.g., power-seeking, self-awareness) of advancedLLMs, we include 6 datasets. In addition to these benchmarks, we haveimplemented a phased public evaluation and benchmark update strategy to ensurethat OpenEval is in line with the development of Chinese LLMs or even able toprovide cutting-edge benchmark datasets to guide the development of ChineseLLMs. In our first public evaluation, we have tested a range of Chinese LLMs,spanning from 7B to 72B parameters, including both open-source and proprietarymodels. Evaluation results indicate that while Chinese LLMs have shownimpressive performance in certain tasks, more attention should be directedtowards broader aspects such as commonsense reasoning, alignment, and safety.
更多查看译文
AI 理解论文
溯源树
样例
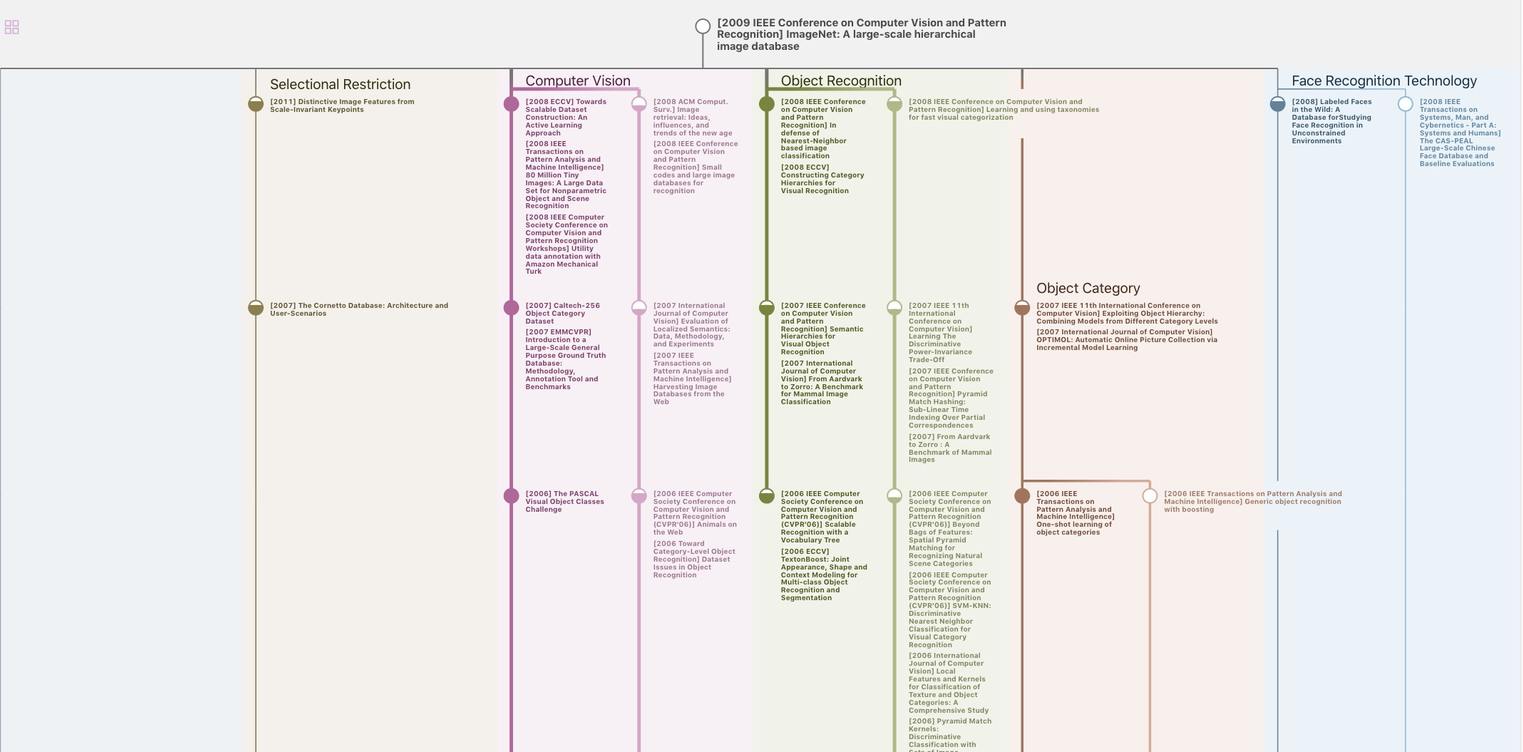
生成溯源树,研究论文发展脉络
Chat Paper
正在生成论文摘要