FairDeDup: Detecting and Mitigating Vision-Language Fairness Disparities in Semantic Dataset Deduplication
2024 IEEE/CVF Conference on Computer Vision and Pattern Recognition (CVPR)(2024)
摘要
Recent dataset deduplication techniques have demonstrated that content-awaredataset pruning can dramatically reduce the cost of training Vision-LanguagePretrained (VLP) models without significant performance losses compared totraining on the original dataset. These results have been based on pruningcommonly used image-caption datasets collected from the web – datasets thatare known to harbor harmful social biases that may then be codified in trainedmodels. In this work, we evaluate how deduplication affects the prevalence ofthese biases in the resulting trained models and introduce an easy-to-implementmodification to the recent SemDeDup algorithm that can reduce the negativeeffects that we observe. When examining CLIP-style models trained ondeduplicated variants of LAION-400M, we find our proposed FairDeDup algorithmconsistently leads to improved fairness metrics over SemDeDup on the FairFaceand FACET datasets while maintaining zero-shot performance on CLIP benchmarks.
更多查看译文
关键词
multimodal,fairness,vision-language,foundation models,human-centered ai,pretraining,vision,language,deduplication
AI 理解论文
溯源树
样例
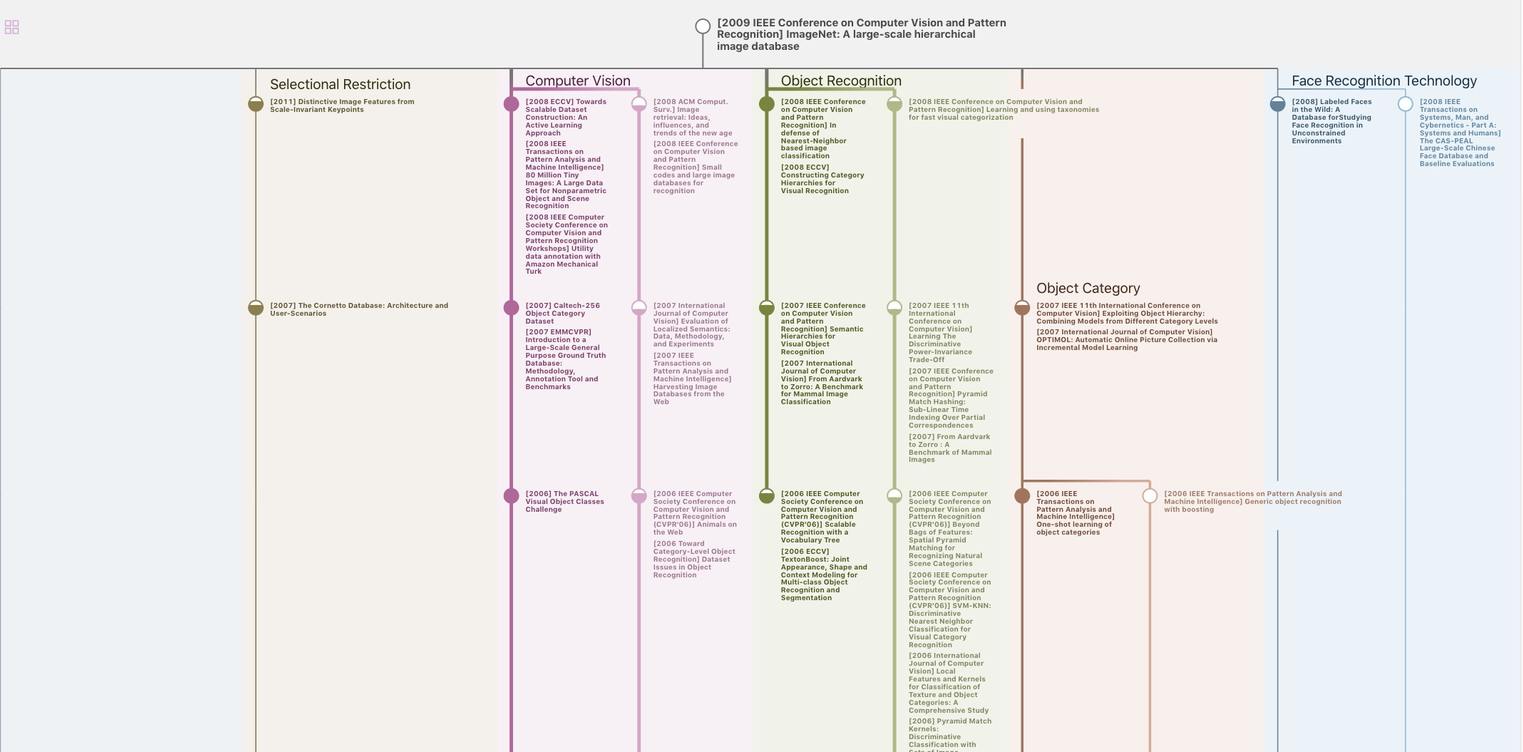
生成溯源树,研究论文发展脉络
Chat Paper
正在生成论文摘要