RAM-Avatar: Real-time Photo-Realistic Avatar from Monocular Videos with Full-body Control
CVPR 2024(2024)
摘要
This paper focuses on advancing the applicability of hu-man avatar learning methods by proposing RAM-Avatar, which learns a Real-time, photo-realistic Avatar that sup-ports full-body control from Monocular videos. To achieve this goal, RAM-Avatar leverages two statistical templates responsible for modeling the facial expression and hand gesture variations, while a sparsely computed dual attention module is introduced upon another body template to facilitate high-fidelity texture rendering for the torsos and limbs. Building on this foundation, we deploy a lightweight yet powerful StyleUnet along with a temporal-aware dis-criminator to achieve real-time realistic rendering. To en-able robust animation for out-of-distribution poses, we pro-pose a Motion Distribution Align module to compensate for the discrepancies between the training and testing motion distribution. Results and extensive experiments conducted in various experimental settings demonstrate the superior-ity of our proposed method, and a real-time live system is proposed to further push research into applications. The training and testing code will be released for research pur-poses.
更多查看译文
关键词
Human Avatar,Generative Adversarial Networks,Monocular
AI 理解论文
溯源树
样例
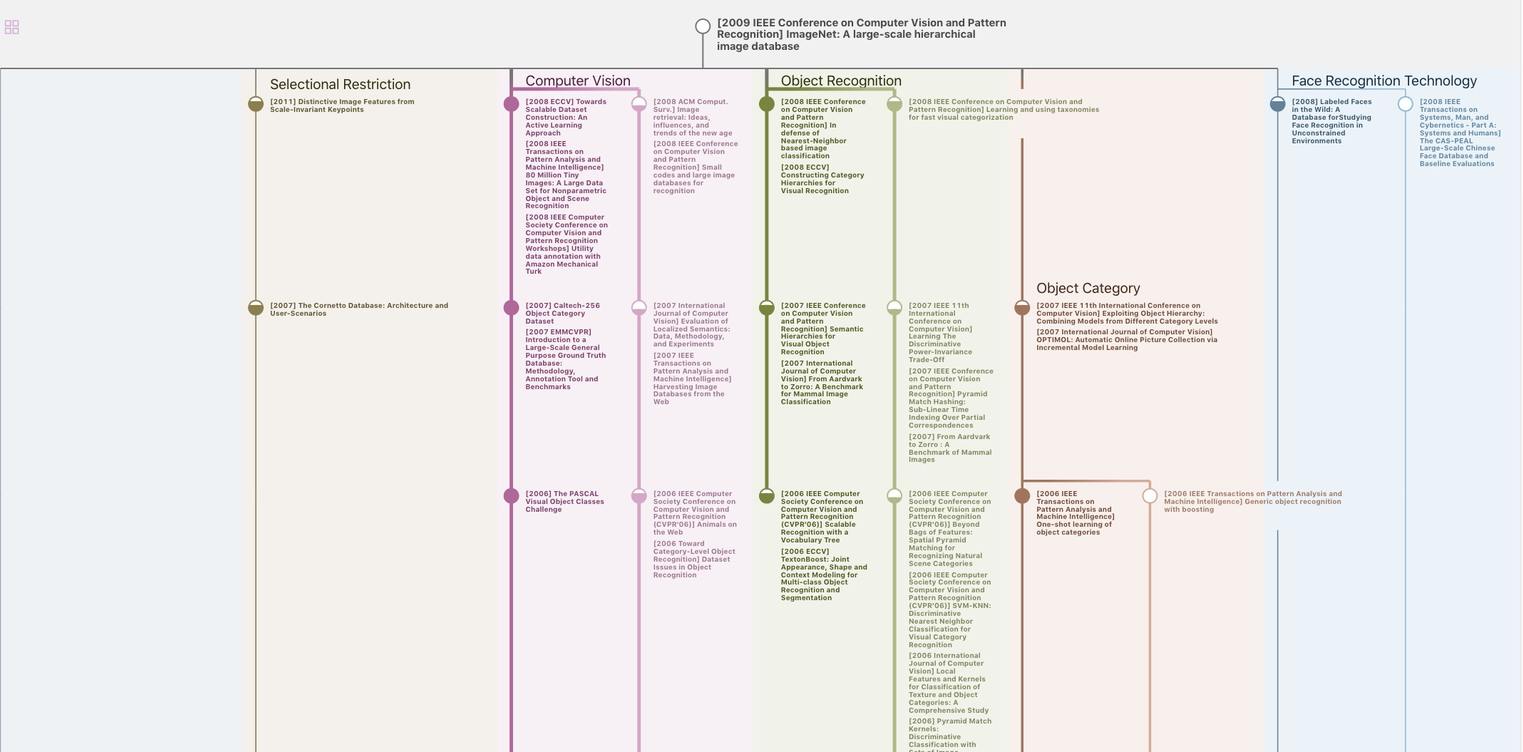
生成溯源树,研究论文发展脉络
Chat Paper
正在生成论文摘要