MRC-based Nested Medical NER with Co-prediction and Adaptive Pre-training
CoRR(2024)
摘要
In medical information extraction, medical Named Entity Recognition (NER) is
indispensable, playing a crucial role in developing medical knowledge graphs,
enhancing medical question-answering systems, and analyzing electronic medical
records. The challenge in medical NER arises from the complex nested structures
and sophisticated medical terminologies, distinguishing it from its
counterparts in traditional domains. In response to these complexities, we
propose a medical NER model based on Machine Reading Comprehension (MRC), which
uses a task-adaptive pre-training strategy to improve the model's capability in
the medical field. Meanwhile, our model introduces multiple word-pair
embeddings and multi-granularity dilated convolution to enhance the model's
representation ability and uses a combined predictor of Biaffine and MLP to
improve the model's recognition performance. Experimental evaluations conducted
on the CMeEE, a benchmark for Chinese nested medical NER, demonstrate that our
proposed model outperforms the compared state-of-the-art (SOTA) models.
更多查看译文
AI 理解论文
溯源树
样例
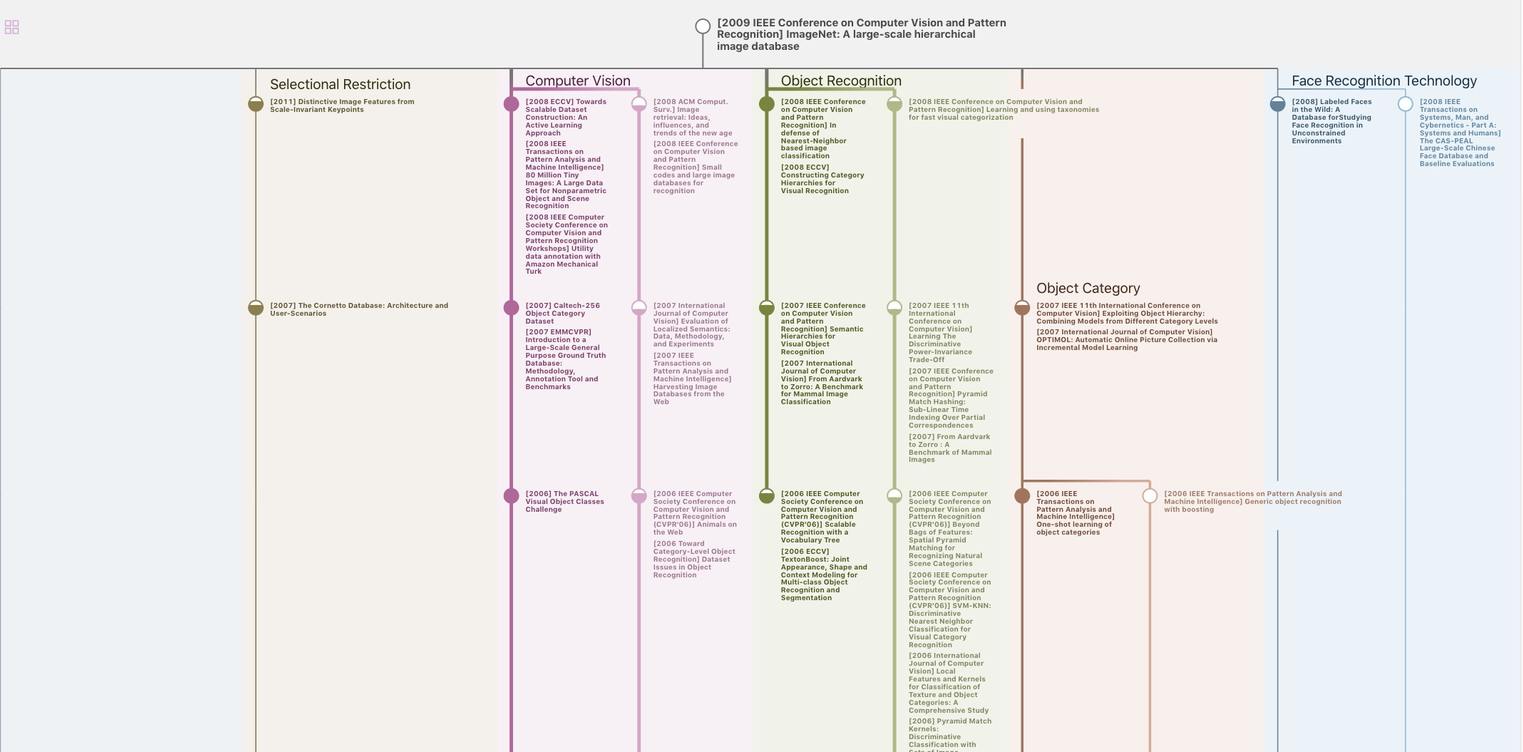
生成溯源树,研究论文发展脉络
Chat Paper
正在生成论文摘要