FireANTs: Adaptive Riemannian Optimization for Multi-Scale Diffeomorphic Registration
CoRR(2024)
摘要
Diffeomorphic Image Registration is a critical part of the analysis in
various imaging modalities and downstream tasks like image translation,
segmentation, and atlas building. Registration algorithms based on optimization
have stood the test of time in terms of accuracy, reliability, and robustness
across a wide spectrum of modalities and acquisition settings. However, these
algorithms converge slowly, are prohibitively expensive to run, and their usage
requires a steep learning curve, limiting their scalability to larger clinical
and scientific studies. In this paper, we develop multi-scale Adaptive
Riemannian Optimization algorithms for diffeomorphic image registration. We
demonstrate compelling improvements on image registration across a spectrum of
modalities and anatomies by measuring structural and landmark overlap of the
registered image volumes. Our proposed framework leads to a consistent
improvement in performance, and from 300x up to 2000x speedup over existing
algorithms. Our modular library design makes it easy to use and allows
customization via user-defined cost functions.
更多查看译文
AI 理解论文
溯源树
样例
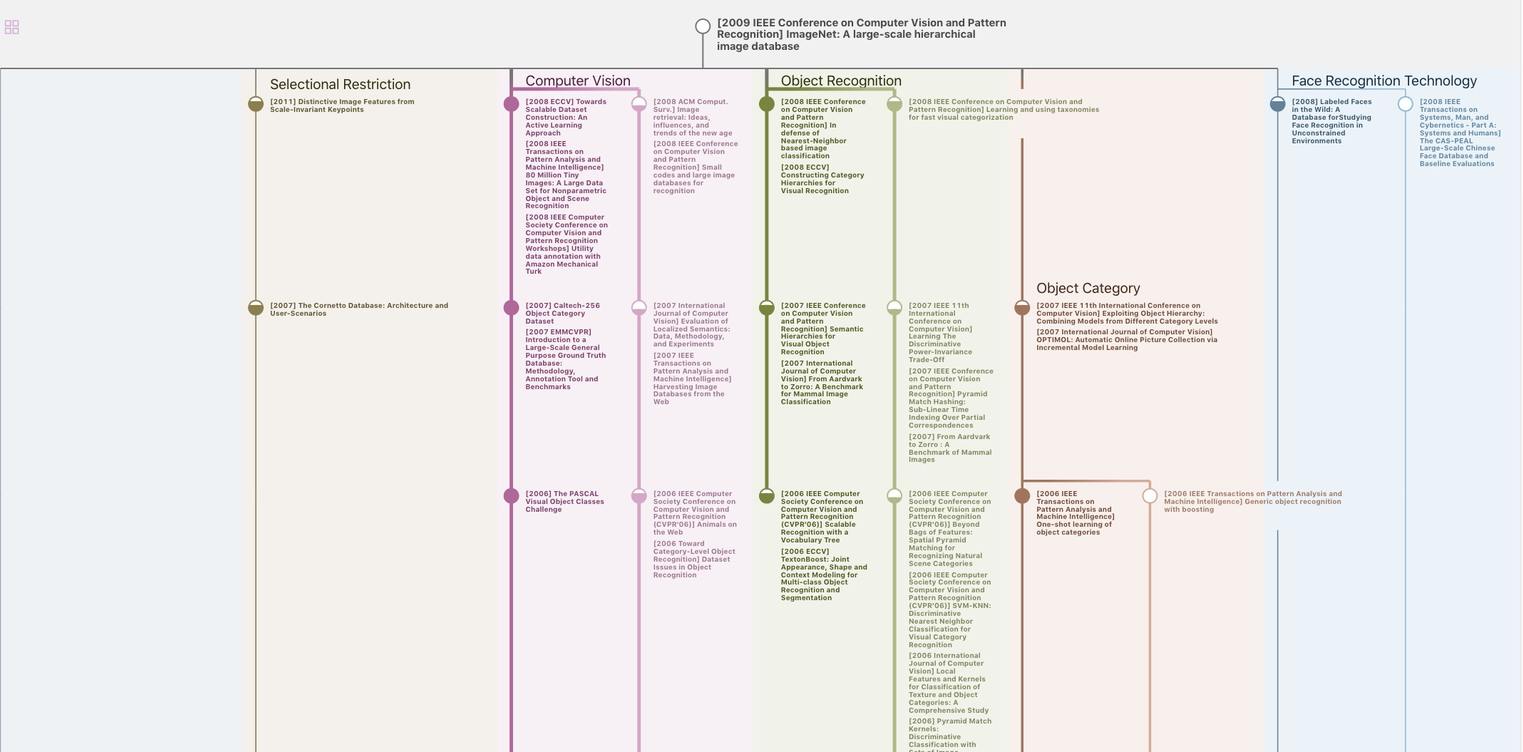
生成溯源树,研究论文发展脉络
Chat Paper
正在生成论文摘要