BioBBC: a Multi-Feature Model That Enhances the Detection of Biomedical Entities
Scientific Reports(2024)
摘要
The rapid increase in biomedical publications necessitates efficient systems to automatically handle Biomedical Named Entity Recognition (BioNER) tasks in unstructured text. However, accurately detecting biomedical entities is quite challenging due to the complexity of their names and the frequent use of abbreviations. In this paper, we propose BioBBC, a deep learning (DL) model that utilizes multi-feature embeddings and is constructed based on the BERT-BiLSTM-CRF to address the BioNER task. BioBBC consists of three main layers; an embedding layer, a Long Short-Term Memory (Bi-LSTM) layer, and a Conditional Random Fields (CRF) layer. BioBBC takes sentences from the biomedical domain as input and identifies the biomedical entities mentioned within the text. The embedding layer generates enriched contextual representation vectors of the input by learning the text through four types of embeddings: part-of-speech tags (POS tags) embedding, char-level embedding, BERT embedding, and data-specific embedding. The BiLSTM layer produces additional syntactic and semantic feature representations. Finally, the CRF layer identifies the best possible tag sequence for the input sentence. Our model is well-constructed and well-optimized for detecting different types of biomedical entities. Based on experimental results, our model outperformed state-of-the-art (SOTA) models with significant improvements based on six benchmark BioNER datasets.
更多查看译文
关键词
Biomedical named entity recognition,Machine learning,Natural language processing,NER,BiLSTM,BioBERT,PubMedBERT
AI 理解论文
溯源树
样例
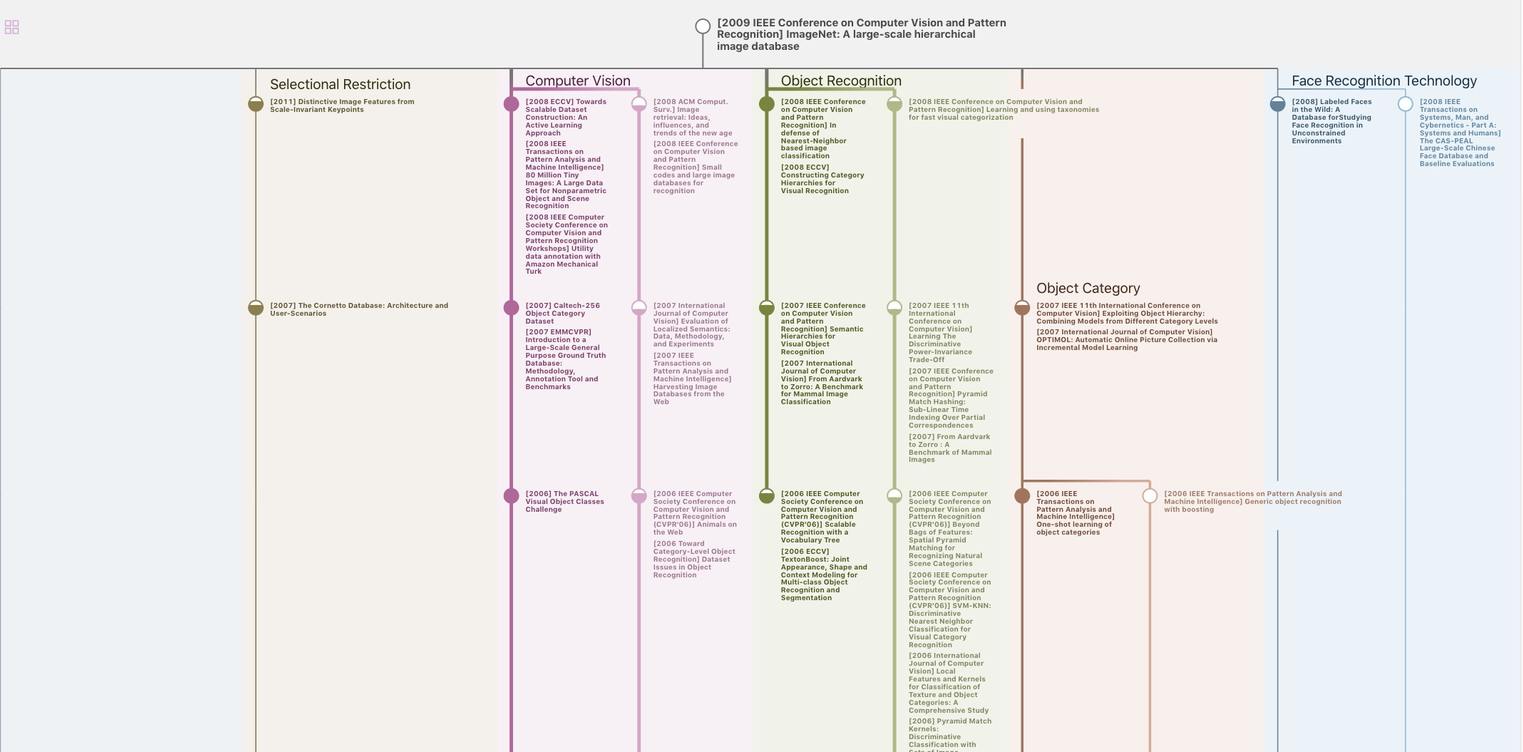
生成溯源树,研究论文发展脉络
Chat Paper
正在生成论文摘要