Dwell in the Beginning: How Language Models Embed Long Documents for Dense Retrieval.
CoRR(2024)
摘要
This study investigates the existence of positional biases inTransformer-based models for text representation learning, particularly in thecontext of web document retrieval. We build on previous research thatdemonstrated loss of information in the middle of input sequences for causallanguage models, extending it to the domain of representation learning. Weexamine positional biases at various stages of training for an encoder-decodermodel, including language model pre-training, contrastive pre-training, andcontrastive fine-tuning. Experiments with the MS-MARCO document collectionreveal that after contrastive pre-training the model already generatesembeddings that better capture early contents of the input, with fine-tuningfurther aggravating this effect.
更多查看译文
AI 理解论文
溯源树
样例
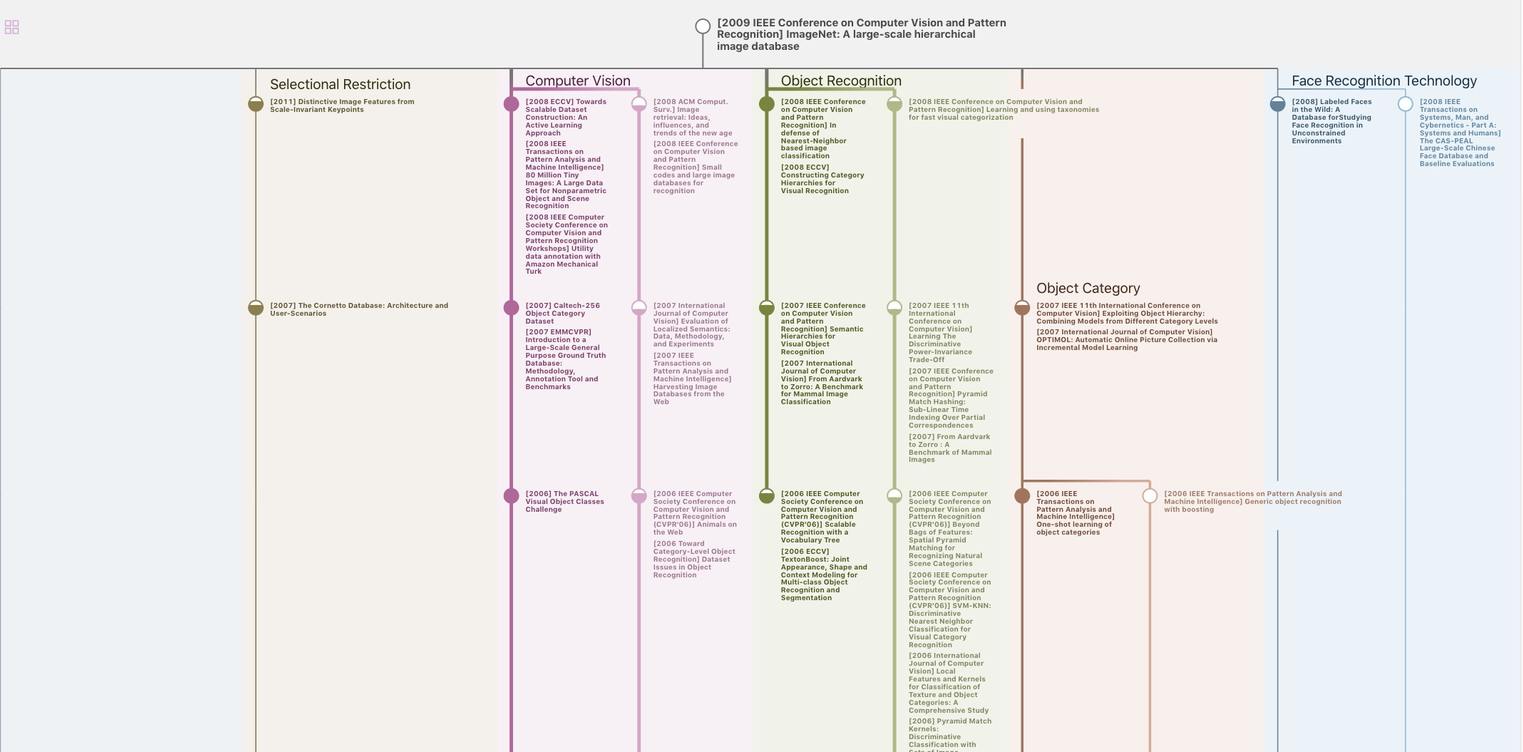
生成溯源树,研究论文发展脉络
Chat Paper
正在生成论文摘要