A Variational Autoencoder for Minimally-Supervised Pulse Shape Discrimination
ANNALS OF NUCLEAR ENERGY(2024)
摘要
We propose a novel approach based on variational autoencoder with Gaussian mixture latent space (GMVAE) to address the challenging problem of pulse shape discrimination (PSD) in organic scintillators, in the presence of pile up. Unlike deterministic charge integration, which is very sensitive to pulse-processing parameters, the GMVAE performances are robust against variations of the hyperparameters. When compared to other supervised machine learning methods, GMVAE requires the fewest training pulses (100) to achieve a classification accuracy within 2% of its optimum performance, i.e., 98.3% accuracy. GMVAE exhibited excellent classification despite the difference in energy spectra between the training and test data sets, which were 14.1 MeV neutron pulses and 239PuBe pulses, respectively. While requiring minimum supervision, GMVAE showed superior PSD performances compared to both deterministic and supervised machine learning approaches. GMVAE is hence particularly suitable for real-time pulse classification, where expert labeling is unavailable and fine tuning of the discrimination parameters impractical.
更多查看译文
关键词
Variational autoencoder,Pulse shape discrimination,Semi-supervised classification,Organic scintillators
AI 理解论文
溯源树
样例
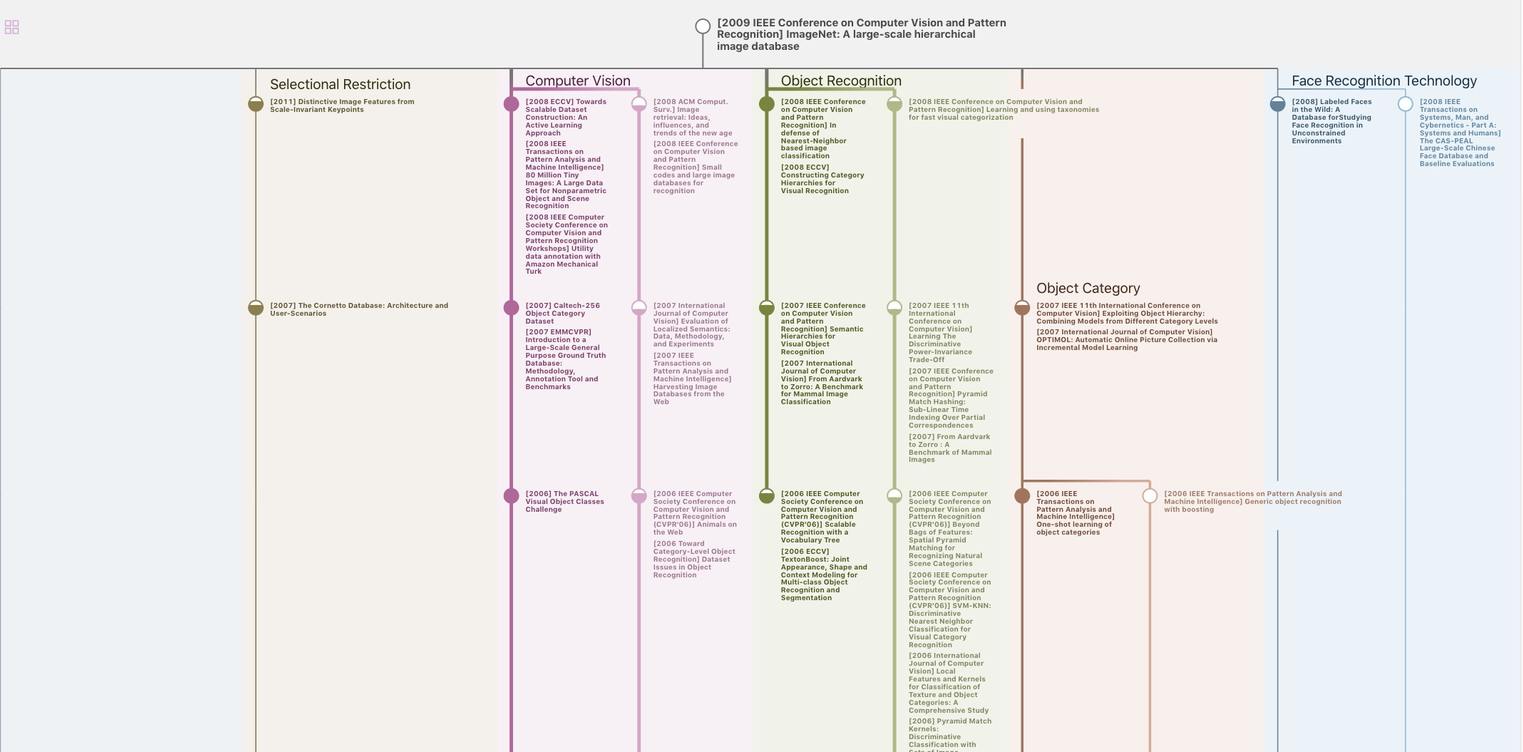
生成溯源树,研究论文发展脉络
Chat Paper
正在生成论文摘要