Gravitational-wave Model for Neutron Star Merger Remnants with Supervised Learning
arxiv(2024)
摘要
We present a time-domain model for the gravitational waves emitted byequal-mass binary neutron star merger remnants for a fixed equation of state.We construct a large set of numerical relativity simulations for a singleequation of state consistent with current constraints, totaling 157 equal-massbinary neutron star merger configurations. The gravitational-wave model isconstructed using the supervised learning method of K-nearest neighborregression. As a first step toward developing a general model with supervisedlearning methods that accounts for the dependencies on equation of state andthe binary masses of the system, we explore the impact of the size of thedataset on the model. We assess the accuracy of the model for a varied datasetsize and number density in total binary mass. Specifically, we consider fivetraining sets of { 20,40, 60, 80, 100} simulations uniformly distributed intotal binary mass. We evaluate the resulting models in terms of faithfulnessusing a test set of 30 additional simulations that are not used during trainingand which are equidistantly spaced in total binary mass. The models achievefaithfulness with maximum values in the range of 0.980 to 0.995. We assessour models simulating signals observed by the three-detector network ofAdvanced LIGO-Virgo. We find that all models with training sets of size equalto or larger than 40 achieve an unbiased measurement of the maingravitational-wave frequency. We confirm that our results do not dependqualitatively on the choice of the (fixed) equation of state. We conclude thattraining sets, with a minimum size of 40 simulations, or a number density ofapproximately 11 simulations per 0.1 M_⊙ of total binary mass, sufficefor the construction of faithful templates for the post-merger signal for asingle equation of state and equal-mass binaries (abbreviated).
更多查看译文
AI 理解论文
溯源树
样例
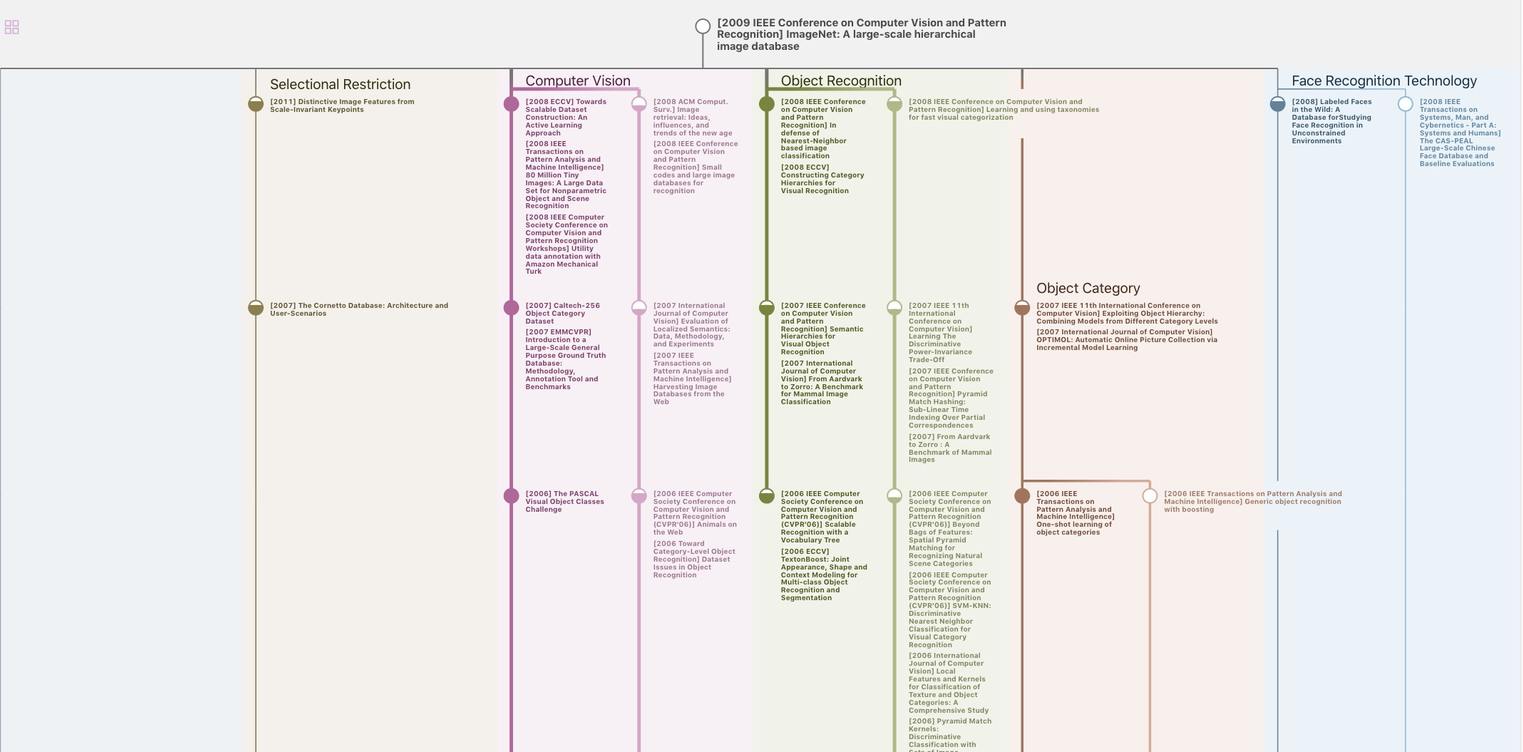
生成溯源树,研究论文发展脉络
Chat Paper
正在生成论文摘要