GaitMotion: A Multitask Dataset for Pathological Gait Forecasting
CoRR(2024)
摘要
Gait benchmark empowers uncounted encouraging research fields such as gait
recognition, humanoid locomotion, etc. Despite the growing focus on gait
analysis, the research community is hindered by the limitations of the
currently available databases, which mostly consist of videos or images with
limited labeling. In this paper, we introduce GaitMotion, a multitask dataset
leveraging wearable sensors to capture the patients' real-time movement with
pathological gait. This dataset offers extensive ground-truth labeling for
multiple tasks, including step/stride segmentation and step/stride length
prediction, empowers researchers with a more holistic understanding of gait
disturbances linked to neurological impairments. The wearable gait analysis
suit captures the gait cycle, pattern, and parameters for both normal and
pathological subjects. This data may prove beneficial for healthcare products
focused on patient progress monitoring and post-disease recovery, as well as
for forensics technologies aimed at person reidentification, and biomechanics
research to aid in the development of humanoid robotics. Moreover, the analysis
has considered the drift in data distribution across individual subjects. This
drift can be attributed to each participant's unique behavioral habits or
potential displacement of the sensor. Stride length variance for normal,
Parkinson's, and stroke patients are compared to recognize the pathological
walking pattern. As the baseline and benchmark, we provide an error of 14.1,
13.3, and 12.2 centimeters of stride length prediction for normal, Parkinson's,
and Stroke gaits separately. We also analyzed the gait characteristics for
normal and pathological gaits in terms of the gait cycle and gait parameters.
更多查看译文
AI 理解论文
溯源树
样例
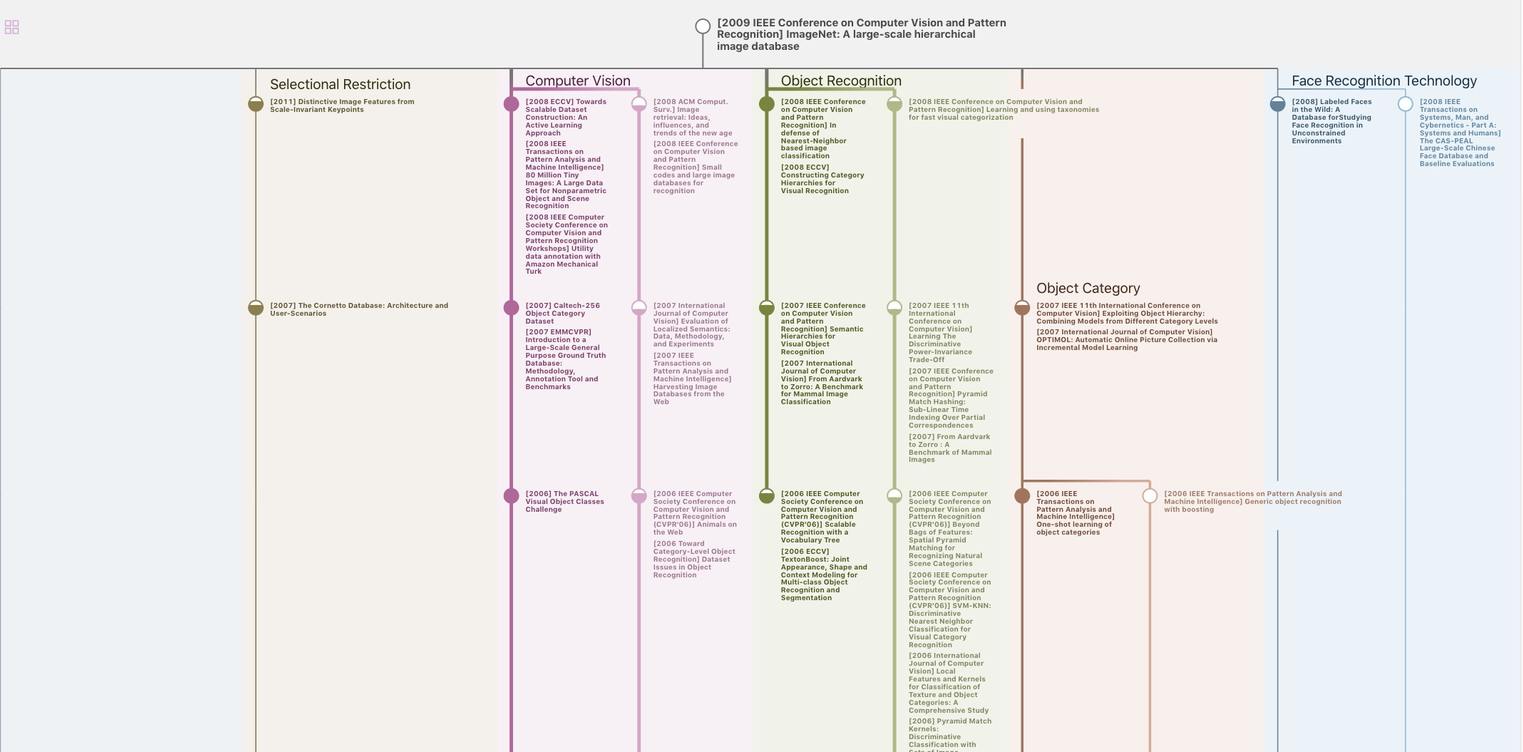
生成溯源树,研究论文发展脉络
Chat Paper
正在生成论文摘要