Multi-perspective Learning for Enhanced User Preferences for Session-Based Recommendation
KNOWLEDGE-BASED SYSTEMS(2024)
摘要
Due to the sparsity and anonymity of interactions within sessions, capturing user preferences by modeling item relationships poses a significant challenge. Previous methods have attempted to utilize collaborative information from adjacent sessions or item attribute knowledge as supplements. However, these methods are prone to introducing interfering information through their session similarity measurement strategies and attribute embedding learning processes, both requiring optimization. Moreover, they often fail to fully model global item relationships. To address these issues, we propose a novel method called Multi -perspective Learning for Enhanced User Preferences (MLEUP) for session -based recommendations. Specifically, we first construct reliable session groups by measuring the overlap and incompatibility of items across sessions to calculate session similarity. Then, by modeling attribute-item and attribute-attribute relationships, along with employing a neighbor -based contrastive self -supervised attribute learning task, we learn high -quality attribute embeddings that capture the user's stable preferences. Next, leveraging the session groups, we model global item multigranularity co -occurrence and high -order sequential relationships through designed attention layers, enabling us to learn informative item embeddings that represent the user's dynamic preferences. Finally, we further model the correlated relationship between the two user preference representations. Our approach ensures that effective attribute knowledge and global collaborative information are learned to mitigate the data sparsity issue, while comprehensively modeling global item relationships to capture the user's complex dynamic preferences. Experimental results demonstrate that MLEUP achieves state-of-the-art performance in terms of precision, MRR, and NDCG. The experimental datasets and source code of MLEUP are publicly available at https://github.com/lean030/MLEUP.
更多查看译文
关键词
Session-based recommendation,Session similarity measurement,Attribute knowledge,Global item relationships
AI 理解论文
溯源树
样例
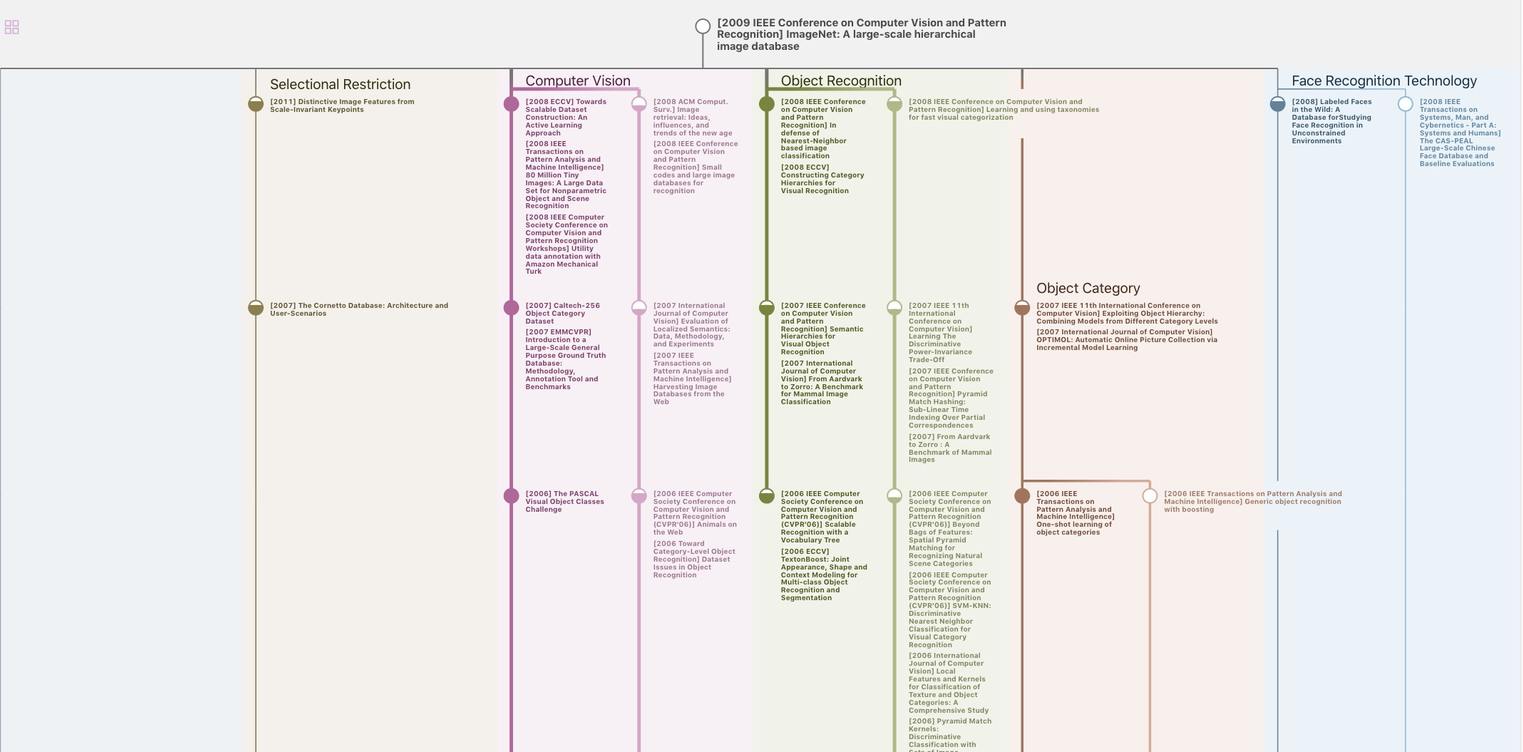
生成溯源树,研究论文发展脉络
Chat Paper
正在生成论文摘要